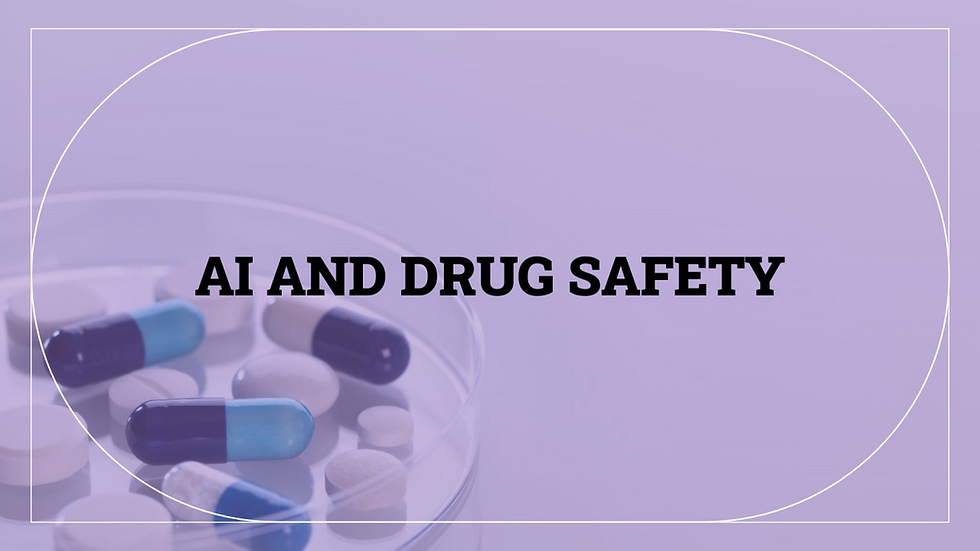
Post-marketing commitments (PMCs) are essential for ensuring the ongoing safety and efficacy of drugs after they have been approved and are available to the public. AI (Artificial Intelligence) plays a crucial role in supporting these commitments by enhancing pharmacovigilance, streamlining data analysis, and improving regulatory compliance. This blog explores the significance of PMCs, challenges faced in fulfilling them, and how AI technologies contribute to their successful implementation in drug safety monitoring.
Understanding Post-Marketing Commitments (PMCs):
Post-marketing commitments are regulatory requirements imposed by health authorities, such as the FDA in the United States or the EMA in Europe, to gather additional data on a drug's safety, efficacy, or optimal use after it has been approved for market. These commitments aim to:
Ensure Continued Safety Monitoring: Monitor for adverse events and safety signals that may not have been detected during clinical trials due to their limited scope or duration.
Evaluate Long-Term Efficacy: Assess the drug's effectiveness in real-world clinical settings, considering factors such as patient demographics, co-morbidities, and concomitant medications.
Validate Risk Management Strategies: Evaluate the effectiveness of risk minimization measures implemented during the drug's approval process.
Inform Regulatory Decisions: Provide regulators with additional data to support decisions related to labeling updates, post-approval changes, or risk mitigation actions.
PMCs typically include commitments to conduct post-approval studies, post-marketing surveillance, or risk evaluation and mitigation strategies (REMS) to ensure ongoing safety monitoring and compliance with regulatory requirements.
Challenges in Fulfilling Post-Marketing Commitments:
Fulfilling PMCs poses several challenges to pharmaceutical companies and regulatory agencies:
Data Complexity and Volume: Managing large volumes of diverse data from multiple sources, including electronic health records (EHRs), adverse event reports, and clinical trial data, can be overwhelming.
Data Integration and Standardization: Integrating data from disparate sources and ensuring consistency and standardization are critical for meaningful analysis and reporting.
Timeliness: Meeting deadlines for reporting and completing post-marketing studies requires efficient data collection, analysis, and submission processes.
Resource Constraints: Allocating sufficient resources, including personnel, technology infrastructure, and budget, to fulfill PMC requirements can be challenging for pharmaceutical companies.
Regulatory Compliance: Ensuring compliance with evolving regulatory standards and guidelines while interpreting and applying complex regulatory requirements adds complexity to PMC fulfillment.
Role of AI in Supporting Post-Marketing Commitments:
AI technologies offer significant advantages in addressing the challenges associated with PMCs and enhancing drug safety monitoring:
Enhanced Pharmacovigilance: AI algorithms can analyze large datasets, including structured and unstructured data, to detect patterns and identify potential safety signals more efficiently than traditional methods.
Real-Time Data Analysis: AI enables real-time monitoring of adverse events and safety signals, facilitating early detection and intervention.
Predictive Analytics: Machine learning models can predict patient outcomes, identify at-risk populations, and prioritize safety concerns for proactive risk management.
Natural Language Processing (NLP): NLP techniques extract insights from unstructured data sources, such as medical literature, social media, and patient forums, to augment pharmacovigilance efforts.
Automated Reporting: AI-driven automation streamlines reporting processes, improves data accuracy, and enhances compliance with regulatory reporting requirements.
Decision Support Systems: AI-powered decision support systems provide evidence-based insights to support regulatory submissions, labeling updates, and risk management strategies.
Best Practices for Leveraging AI in PMCs:
To maximize the benefits of AI in supporting PMCs and drug safety monitoring, pharmaceutical companies can adopt the following best practices:
Data Integration and Management: Implement robust data integration strategies to centralize and standardize data from multiple sources, ensuring data quality and accessibility.
AI Model Development: Develop customized AI models tailored to specific PMC requirements and therapeutic areas, considering factors such as patient demographics and disease characteristics.
Continuous Monitoring and Surveillance: Establish real-time monitoring systems powered by AI to continuously monitor safety data and identify emerging risks promptly.
Collaboration and Partnerships: Foster collaboration with AI technology providers, research institutions, and regulatory agencies to leverage expertise and resources in AI application development.
Regulatory Compliance: Ensure AI-driven processes comply with regulatory standards for data privacy, security, and transparency, incorporating regulatory guidance into AI model validation and deployment.
Case Studies and Applications:
Several examples demonstrate the successful application of AI in supporting PMCs and enhancing drug safety:
FDA Sentinel Initiative: The FDA's Sentinel System uses AI and big data analytics to monitor the safety of FDA-regulated medical products, including drugs, vaccines, and medical devices, in real time.
EMA Edra Vigilance: Edra Vigilance, the European database of suspected adverse drug reaction reports, utilizes AI and machine learning to enhance pharmacovigilance activities and support regulatory decision-making.
Pharmaceutical Industry Initiatives: Leading pharmaceutical companies integrate AI technologies into their pharmacovigilance and post-marketing surveillance strategies to improve data analysis capabilities and meet PMC requirements.
Future Trends in AI and Drug Safety Monitoring:
The future of AI in supporting PMCs and drug safety monitoring is promising, with emerging trends focusing on:
Advanced AI Algorithms: Continued development of advanced AI algorithms, including deep learning and reinforcement learning, to enhance predictive capabilities and support personalized medicine.
Real-World Evidence Generation: AI-driven analysis of real-world data (RWD) from EHRs, wearables, and digital health platforms to generate real-world evidence for regulatory submissions and PMC fulfillment.
AI-Powered Digital Twins: Digital twins of drugs and medical devices, simulated using AI models, to predict safety profiles, optimize clinical trial designs, and inform post-marketing studies.
Ethical AI and Regulatory Frameworks: Integration of ethical AI principles and regulatory frameworks to ensure responsible AI deployment in drug safety monitoring and compliance.
Conclusion:
AI technologies play a pivotal role in supporting post-marketing commitments for drug safety by enhancing pharmacovigilance, facilitating real-time data analysis, and improving regulatory compliance. By leveraging AI-driven insights and automation, pharmaceutical companies can fulfill PMC requirements more effectively, detect safety signals promptly, and ensure ongoing monitoring of drug safety in real-world clinical settings. Continued investment in AI innovation, collaboration with regulatory agencies, and adoption of best practices will further strengthen the role of AI in advancing drug safety and enhancing patient outcomes globally.
Comentários