How AI Transforms Data Aggregation for Drug Safety Analysis
- Chaitali Gaikwad
- Jul 10, 2024
- 5 min read
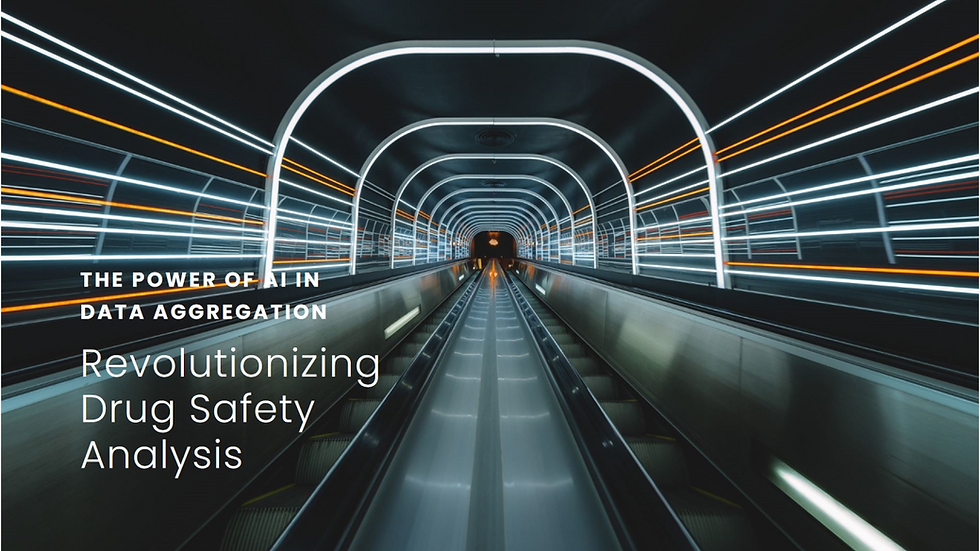
The integration of artificial intelligence (AI) in healthcare is revolutionizing numerous facets of the industry, with drug safety analysis standing out as a particularly transformed domain. AI's ability to aggregate and analyze vast amounts of data swiftly and accurately is enhancing the way we understand, monitor, and ensure drug safety. This blog delves into how AI is transforming data aggregation for drug safety analysis, exploring its methodologies, benefits, and future potential.
Drug safety analysis, also known as pharmacovigilance, is crucial in ensuring that medications are safe and effective for public use. It involves the detection, assessment, understanding, and prevention of adverse effects or any other drug-related problems. Historically, this process has been labor-intensive and time-consuming, relying heavily on manual data collection and analysis.
Traditional Challenges in Drug Safety Analysis:
Volume of Data: The sheer amount of data generated from clinical trials, adverse event reports, medical literature, and patient records is overwhelming. Manually processing this data is not only inefficient but also prone to errors.
Data Silos: Information relevant to drug safety is often stored in disparate systems and formats, making it difficult to access and integrate.
Latency in Detection: Identifying adverse drug reactions (ADRs) and other safety signals promptly is critical. Traditional methods can delay detection, potentially leading to patient harm.
Complexity of Data: Medical data is highly complex, often containing unstructured information such as doctor’s notes, patient feedback, and social media posts. Extracting meaningful insights from this data requires sophisticated analytical capabilities.
How AI Enhances Data Aggregation for Drug Safety:
Advanced Data Integration
AI excels in integrating data from various sources, including electronic health records (EHRs), clinical trial data, social media, and adverse event reporting systems. By breaking down data silos, AI creates a comprehensive dataset that provides a holistic view of drug safety.
Natural Language Processing (NLP): NLP algorithms can process unstructured data, such as physician notes and patient reports, to extract relevant information on drug safety. This enhances the depth and breadth of data available for analysis.
Interoperability: AI facilitates interoperability between different data systems, allowing seamless data sharing and integration. This ensures that all relevant data points are considered in safety analysis.
Real-Time Monitoring and Signal Detection:
AI-powered systems can monitor drug safety data in real-time, rapidly identifying potential safety signals. This capability is critical for timely interventions and mitigating risks associated with adverse drug reactions.
Machine Learning Algorithms: Machine learning (ML) models can be trained to recognize patterns indicative of safety issues. These models continuously learn and improve as they process more data, enhancing their accuracy over time.
Automated Signal Detection: AI systems can automatically detect signals of adverse reactions, reducing the time it takes to identify and address potential safety concerns. This proactive approach contrasts with traditional, reactive methods.
Enhanced Predictive Analytics:
Predictive analytics, powered by AI, enables the anticipation of potential drug safety issues before they manifest widely. By analyzing historical data and identifying trends, AI can forecast future safety risks.
Risk Stratification: AI algorithms can stratify patients based on their risk of experiencing adverse reactions, allowing for personalized monitoring and intervention strategies.
Simulation Models: AI can create simulation models to predict the impact of drugs on various patient populations, identifying potential risks and benefits before widespread use.
Improved Accuracy and Efficiency:
The use of AI in data aggregation for drug safety significantly improves both the accuracy and efficiency of the process. AI systems can process large datasets quickly, reducing the time required for analysis and minimizing human error.
Automated Data Processing: AI automates the tedious and repetitive tasks involved in data aggregation, freeing up human resources for more complex analytical tasks.
Error Reduction: By minimizing manual data handling, AI reduces the likelihood of errors in data entry and analysis, ensuring more reliable safety assessments.
Case Studies and Real-World Applications:
AI in Pharmacovigilance: The FDA's Sentinel Initiative
The U.S. Food and Drug Administration (FDA) has implemented the Sentinel Initiative, an active surveillance system that uses AI to monitor the safety of medical products. Sentinel analyzes data from multiple sources, including EHRs and insurance claims, to identify potential safety signals. The system has successfully identified several safety issues, leading to timely regulatory actions.
AI in Clinical Trials: Accelerating Safety Assessments
Pharmaceutical companies are increasingly using AI to enhance safety monitoring in clinical trials. AI systems can analyze real-time data from ongoing trials, quickly identifying adverse events and ensuring participant safety. For example, IBM Watson has been used to analyze clinical trial data, reducing the time required for safety assessments and speeding up the drug development process.
Social Media Monitoring: Harnessing Public Feedback
Social media platforms are valuable sources of real-world data on drug safety. AI-driven tools can scan social media posts for mentions of adverse drug reactions, providing early warning signals. Companies like AstraZeneca have employed AI to monitor social media for safety signals, supplementing traditional pharmacovigilance methods.
Future Directions and Potential of AI in Drug Safety:
Advanced Machine Learning Techniques
Future advancements in machine learning, such as deep learning and reinforcement learning, promise to further enhance drug safety analysis. These techniques can model complex relationships in data, providing more accurate predictions and insights.
Deep Learning: Deep learning algorithms can analyze vast amounts of data with multiple layers of abstraction, identifying intricate patterns indicative of drug safety issues.
Reinforcement Learning: This approach can optimize decision-making processes in drug safety, continuously learning from interactions and outcomes to improve safety monitoring strategies.
Integration with Genomic Data:
The integration of AI with genomic data can lead to personalized drug safety analysis. By understanding how genetic variations influence drug responses, AI can help predict adverse reactions and tailor treatments to individual patients.
Pharmacogenomics: AI can analyze genomic data to identify genetic markers associated with adverse drug reactions, enabling personalized medicine approaches.
Precision Medicine: Combining AI with genomic data supports the development of precision medicine, where treatments are customized based on an individual's genetic makeup.
Blockchain for Data Integrity:
Blockchain technology can enhance the integrity and transparency of drug safety data. By providing a secure and immutable ledger, blockchain ensures that data used in safety analysis is accurate and tamper-proof.
Secure Data Sharing: Blockchain facilitates secure and transparent data sharing among stakeholders, enhancing collaboration in drug safety monitoring.
Data Provenance: Blockchain tracks the origin and changes to data, ensuring its authenticity and reliability.
Ethical Considerations and AI Governance:
As AI becomes more integral to drug safety analysis, ethical considerations and governance frameworks will be crucial to ensure responsible use.
Bias Mitigation: Ensuring that AI algorithms are free from biases that could impact safety assessments is essential. Regular auditing and validation of AI models can help mitigate bias.
Transparency and Accountability: Clear guidelines on the use of AI in drug safety, along with transparent reporting of AI-driven decisions, will enhance accountability and trust.
Conclusion:
AI is undeniably transforming data aggregation for drug safety analysis, offering enhanced accuracy, efficiency, and predictive capabilities. By integrating data from diverse sources, providing real-time monitoring, and leveraging advanced machine learning techniques, AI is revolutionizing how we ensure the safety of medications. As technology continues to evolve, the potential for AI to further improve drug safety analysis is immense, promising a future where adverse drug reactions are promptly identified and mitigated, ultimately safeguarding public health. Embracing AI in drug safety not only enhances current methodologies but also paves the way for innovative approaches that can adapt to the ever-changing landscape of healthcare.
Comentarios