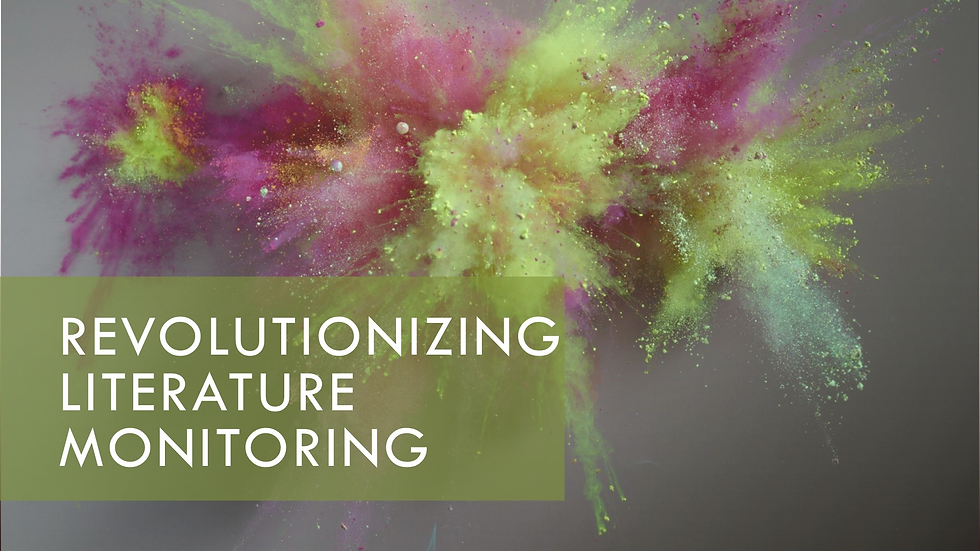
In the complex and ever-evolving field of pharmacovigilance, literature monitoring plays a crucial role in ensuring drug safety. Traditionally, this process has involved extensive manual review of scientific publications, clinical trial reports, and other sources to identify potential adverse drug reactions (ADRs) and safety concerns. However, with the advent of artificial intelligence (AI), the landscape of literature monitoring is undergoing a significant transformation. This blog explores how AI is revolutionizing literature monitoring in pharmacovigilance, enhancing efficiency, accuracy, and overall drug safety.
The Traditional Approach to Literature Monitoring:
Before delving into AI’s impact, it’s important to understand the traditional methods of literature monitoring in pharmacovigilance. Historically, pharmacovigilance professionals manually sifted through a vast number of scientific journals, medical databases, and other literature sources to identify relevant information about drug safety. This process involves:
Database Searches: Utilizing databases like PubMed, Embase, and others to find relevant studies and reports.
Manual Review: Experts read through articles, abstracts, and reports to identify potential signals of ADRs.
Data Extraction: Extracting pertinent data from identified sources to be analyzed and incorporated into safety profiles.
Signal Detection: Analyzing the extracted data to detect potential safety signals and assess their significance.
This method, while effective, is labor-intensive and time-consuming. With the increasing volume of medical literature, the traditional approach faces challenges related to scalability, speed, and human error.
The Rise of AI in Pharmacovigilance:
AI, particularly machine learning and natural language processing (NLP), has introduced innovative solutions to the challenges faced by traditional literature monitoring methods. Here’s how AI is transforming the field:
1. Enhanced Data Processing
AI algorithms can process vast amounts of data far more quickly than human reviewers. Machine learning models, particularly those utilizing NLP, can scan and analyze thousands of articles and reports in a fraction of the time it would take a human. This capability allows for:
Rapid Literature Review: AI systems can perform extensive searches across multiple databases simultaneously, identifying relevant literature with high efficiency.
Automated Data Extraction: AI can automatically extract relevant information from articles, such as drug names, adverse events, and patient demographics, reducing manual data entry errors.
2. Improved Accuracy and Consistency
AI algorithms, especially those based on deep learning, can enhance the accuracy of literature monitoring by minimizing human error and bias. Key advantages include:
Consistent Analysis: Unlike human reviewers who may have varying levels of expertise or bias, AI systems apply consistent criteria to all literature, ensuring uniformity in the review process.
Precision in Signal Detection: Advanced machine learning models can identify subtle patterns and correlations in data that might be missed by human reviewers, leading to more accurate signal detection.000
3. Sophisticated Natural Language Processing
Natural Language Processing (NLP) is a subset of AI that focuses on the interaction between computers and human language. In pharmacovigilance, NLP algorithms can:
Understand Context: NLP can interpret the context in which drugs and adverse events are mentioned, distinguishing between relevant and irrelevant information.
Extract Relevant Data: Advanced NLP models can extract specific data points from unstructured text, such as descriptions of adverse reactions and their severity.
Identify Emerging Trends: NLP can detect emerging safety trends by analyzing large volumes of text data, helping to identify potential safety signals early.
4. Real-Time Monitoring and Updates
AI enables real-time monitoring of literature, allowing for timely updates to drug safety profiles. Key benefits include:
Continuous Surveillance: AI systems can continuously scan new literature and updates in real-time, ensuring that the latest information is incorporated into safety assessments.
Timely Alerts: Automated alerts can notify pharmacovigilance professionals of new safety signals or updates, facilitating swift action and decision-making.
5. Integration with Other Data Sources
AI can integrate literature monitoring with other data sources, such as electronic health records (EHRs), clinical trial data, and post-marketing surveillance reports. This integration provides a more comprehensive view of drug safety and helps to:
Correlate Data: By combining literature findings with real-world data, AI can help correlate ADRs with specific patient populations, drug interactions, and other factors.
Enhance Signal Detection: A holistic view of safety data improves the ability to detect and assess potential signals, leading to more informed risk management decisions.
Case Studies and Real-World Application:
To illustrate the impact of AI on literature monitoring in pharmacovigilance, let’s explore a few real-world applications and case studies:
1. IBM Watson for Drug Discovery
IBM Watson for Drug Discovery is a prominent example of AI applied to literature monitoring. The platform uses AI and NLP to analyze scientific literature and clinical trial data to identify potential drug candidates and safety signals. Key features include:
Automated Literature Review: Watson can analyze vast amounts of scientific literature to identify relevant research and extract pertinent data.
Predictive Analytics: The platform uses AI to predict potential drug interactions and adverse effects, supporting proactive safety management.
2. Elsevier’s PharmaPendium
Elsevier’s PharmaPendium utilizes AI and machine learning to enhance the monitoring of pharmaceutical literature. Key capabilities include:
Enhanced Search: The platform uses AI to improve search accuracy and retrieve relevant literature more effectively.
Automated Data Extraction: PharmaPendium’s AI-driven tools automate the extraction of critical safety information from literature sources.
3. MedDRA and AI Integration
The Medical Dictionary for Regulatory Activities (MedDRA) is a standardized medical terminology used in pharmacovigilance. Recent advancements in AI have integrated with MedDRA to improve literature monitoring by:
Automated Coding: AI algorithms can automatically code adverse events using MedDRA terminology, streamlining the data analysis process.
Enhanced Signal Detection: Integration with AI helps in detecting and analyzing safety signals more effectively by leveraging MedDRA’s standardized vocabulary.
Challenges and Considerations:
Despite the numerous benefits of AI in literature monitoring, several challenges and considerations remain:
Data Quality: The effectiveness of AI algorithms depends on the quality of the data they are trained on. Ensuring high-quality, diverse datasets is crucial for accurate outcomes.
Algorithm Bias: AI systems can inherit biases present in training data, leading to skewed results. Continuous monitoring and refinement of algorithms are necessary to mitigate bias.
Regulatory Compliance: AI applications in pharmacovigilance must comply with regulatory standards and guidelines to ensure data privacy, security, and accuracy.
Human Oversight: While AI enhances efficiency, human expertise is still essential for interpreting complex safety signals and making informed decisions.
Future Directions:
The future of AI in literature monitoring holds exciting possibilities:
Advanced Machine Learning Models: Ongoing advancements in machine learning, including reinforcement learning and generative models, will further enhance AI’s capabilities in literature monitoring.
Integration with Other Technologies: Combining AI with emerging technologies such as blockchain for data integrity and augmented reality for visualization could offer new opportunities in pharmacovigilance.
Personalized Safety Monitoring: AI could facilitate personalized safety monitoring by integrating individual patient data with literature findings, leading to more tailored risk management strategies.
Conclusion:
AI is fundamentally transforming literature monitoring in pharmacovigilance by enhancing data processing, accuracy, and real-time surveillance. Through advanced machine learning and natural language processing, AI enables more efficient and accurate detection of safety signals, ultimately contributing to better drug safety and patient outcomes. While challenges remain, the ongoing development and integration of AI technologies promise to further revolutionize pharmacovigilance practices, paving the way for more proactive and informed approaches to drug safety. As AI continues to evolve, its impact on literature monitoring will undoubtedly become even more profound, driving innovations in the field and ensuring safer therapies for patients worldwide.
Comments