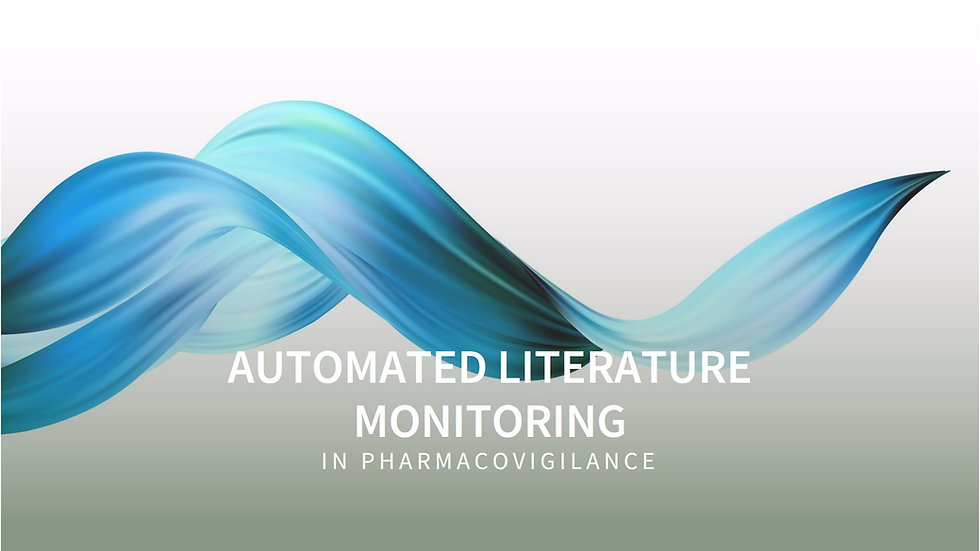
Pharmacovigilance, the practice of monitoring the safety of drugs and medical devices, heavily relies on literature review to detect adverse events and emerging safety signals. Traditionally, this process has been manual, time-consuming, and prone to human error. However, Artificial Intelligence (AI) is revolutionizing literature review by automating tasks, improving accuracy, and enabling faster detection of critical safety information. This blog explores how AI transforms literature review in pharmacovigilance, the tools and technologies driving this change, and the benefits for the pharmaceutical industry.
1. Challenges in Traditional Literature Review
Literature monitoring involves scanning a wide range of sources, including scientific journals, case reports, conference proceedings, and regulatory updates. However, the conventional approach poses several challenges:
Manual Processes: Pharmacovigilance teams must manually read through thousands of abstracts and articles.
Information Overload: The volume of literature published daily makes it difficult to stay up-to-date.
Inconsistent Reporting: Adverse events are often reported in varied formats, complicating data extraction.
Delays in Signal Detection: Manual reviews can introduce delays, increasing the risk of missing early warning signs.
High Resource Costs: Organizations must allocate significant time and personnel to maintain comprehensive surveillance.
AI offers an efficient alternative to traditional methods by automating repetitive tasks and enhancing the quality of literature review.
2. How AI is Integrated into Literature Review Processes
AI technologies, including natural language processing (NLP) and machine learning (ML), are used to automate different aspects of literature monitoring. These tools enhance pharmacovigilance processes by quickly identifying relevant information and extracting insights from unstructured data sources.
2.1 Automated Literature Search
AI-powered search engines are designed to sift through vast repositories of scientific literature, preprints, and regulatory databases. These engines use NLP to:
Identify articles relevant to specific drugs or medical conditions.
Search for synonyms, abbreviations, and contextual terms to avoid missing critical information.
Rank articles by relevance, saving reviewers time.
This automation reduces the need for manual searches and ensures comprehensive coverage.
2.2 Intelligent Filtering and Prioritization
AI algorithms classify and filter articles based on their relevance to pharmacovigilance. For instance, AI can prioritize articles reporting serious adverse events (SAEs) over those with non-critical information. The system can assign confidence scores to prioritize urgent reviews and reduce false positives.
2.3 Named Entity Recognition (NER) for Data Extraction
NER algorithms extract specific information, such as drug names, patient demographics, dosages, and reported outcomes, from unstructured texts. By transforming this information into structured data, AI ensures consistent and accurate reporting.
3. AI-Driven Platforms and Tools for Literature Monitoring
Several AI-based platforms are transforming how pharmaceutical companies perform literature reviews.
Embase and PubMed Integrations: AI-enabled tools integrate with Embase and PubMed to continuously monitor scientific articles.
AI-Powered Dashboards: These dashboards visualize literature trends, offering real-time updates on emerging safety signals.
Smart Alerts: Automated alerts notify pharmacovigilance teams when new literature relevant to a drug or adverse event is published.
Collaborative Platforms: Cloud-based AI solutions enable multiple team members to work on reviews simultaneously, improving workflow efficiency.
These platforms streamline the end-to-end literature review process, ensuring no critical information is overlooked.
4. The Role of Machine Learning in Literature Review
Machine learning (ML) models improve their accuracy over time by learning from historical data. In pharmacovigilance literature review, ML can:
Identify patterns in previous safety reports to predict potential risks.
Refine search strategies based on user feedback, ensuring continuous improvement.
Detect correlations between multiple adverse events and their potential causes, even in complex data sets.
By leveraging ML, pharmacovigilance teams can identify emerging risks earlier than traditional methods allow.
5. Case Study: Automating Literature Review for Drug Safety Monitoring
A leading pharmaceutical company implemented AI-powered literature monitoring to automate the detection of adverse events related to one of their high-risk medications.
Process Overview:
Data Collection: The AI tool scanned multiple databases and journals, extracting all articles mentioning the drug and its adverse effects.
Filtering and Categorization: Irrelevant articles were filtered out, and priority was given to those reporting serious side effects.
Data Extraction: The AI system automatically extracted adverse event information and integrated it into the company’s pharmacovigilance database.
Alerts and Reporting: Automated alerts were sent to the safety team, enabling timely review and reporting to regulatory authorities.
Outcome:
The company experienced a 30% reduction in review time and detected emerging safety signals two months earlier than expected. This early detection facilitated swift action, minimizing potential risks to patients.
6. Benefits of AI-Driven Literature Review in Pharmacovigilance
The integration of AI brings several advantages to pharmacovigilance literature monitoring:
6.1 Increased Efficiency and Speed
AI significantly reduces the time required to search, filter, and review literature. Automated processes free up pharmacovigilance teams to focus on more strategic activities, such as analyzing safety trends.
6.2 Improved Accuracy and Consistency
By minimizing human errors, AI ensures that literature reviews are thorough and consistent. NLP algorithms identify adverse events accurately, even when reported using varied terminology.
6.3 Comprehensive Coverage
AI-powered tools can monitor multiple sources simultaneously, ensuring that no relevant information is missed. They can also translate and analyze non-English articles, offering a global perspective on drug safety.
6.4 Real-Time Monitoring
With continuous scanning and smart alerts, AI enables real-time literature monitoring. This allows pharmaceutical companies to respond quickly to emerging safety concerns.
6.5 Cost Reduction
By automating labor-intensive processes, AI reduces the costs associated with manual literature review. This cost efficiency benefits organizations of all sizes, from pharmaceutical giants to smaller research firms.
7. Regulatory Compliance and AI
Regulatory bodies, such as the European Medicines Agency (EMA) and the U.S. Food and Drug Administration (FDA), require pharmaceutical companies to maintain rigorous pharmacovigilance practices. AI-based literature review ensures compliance by:
Fulfilling Timely Reporting Obligations: Automated systems ensure literature reviews are completed within regulatory deadlines.
Audit-Ready Documentation: AI tools maintain a detailed audit trail, documenting search strategies, filtering criteria, and review decisions.
Ensuring Transparency: AI systems provide clear insights into how data is collected and analyzed, satisfying regulatory scrutiny.
Many regulatory authorities are also encouraging the adoption of innovative technologies, including AI, to enhance drug safety practices.
8. Challenges and Future Directions
While AI brings numerous benefits to literature review, there are still challenges to address:
8.1 Data Quality Issues
AI systems rely on high-quality data for accurate results. Poorly reported adverse events or incomplete data can affect the performance of AI models.
8.2 Integration with Legacy Systems
Pharmaceutical companies may face challenges integrating AI-powered tools with existing pharmacovigilance databases and workflows.
8.3 Regulatory Acceptance
Although regulatory bodies are embracing AI, companies must ensure their AI tools meet compliance standards and produce transparent, auditable outputs.
Future Trends
AI-Augmented Human Review: AI will assist, rather than replace, human reviewers by pre-analyzing data and suggesting relevant insights.
Predictive Safety Monitoring: Advanced ML models will predict emerging safety concerns, allowing companies to take preventive actions.
Collaborative AI Platforms: AI-driven platforms will enable seamless collaboration across global pharmacovigilance teams.
9. Conclusion
AI is transforming literature review in pharmacovigilance by automating repetitive tasks, improving data quality, and enabling faster detection of safety signals. With tools that offer intelligent filtering, data extraction, and real-time monitoring, pharmaceutical companies can stay ahead of emerging risks and ensure regulatory compliance. As AI continues to evolve, its role in pharmacovigilance will become even more integral, enabling safer medicines and better patient outcomes. By embracing AI, organizations can streamline their literature review processes, reduce costs, and enhance their ability to protect public health.
This blog demonstrates how AI has become a game-changer in pharmacovigilance, enabling pharmaceutical companies to monitor literature with greater accuracy, speed, and efficiency. The future promises even more innovative applications, making AI indispensable for ensuring drug safety in an increasingly complex world.
Comments