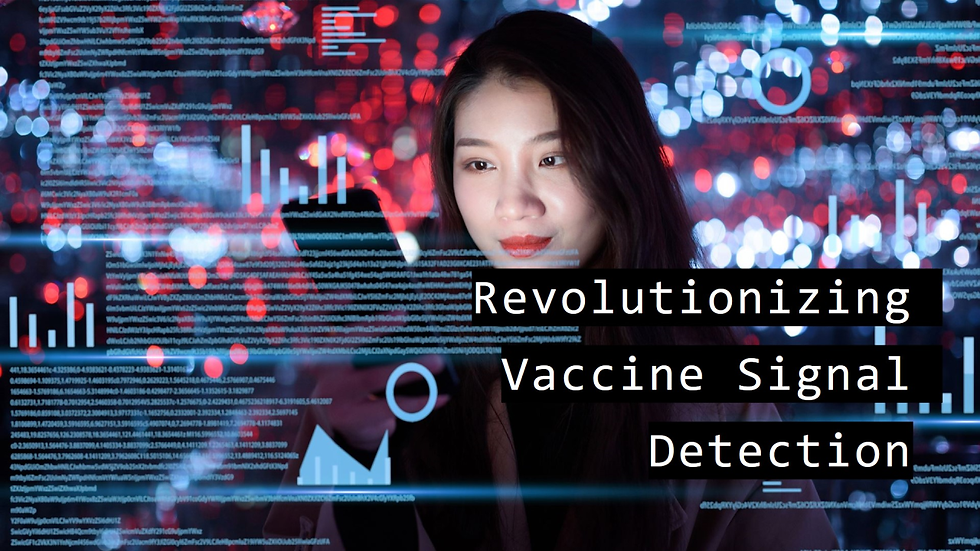
Artificial Intelligence (AI) has emerged as a transformative technology in healthcare, revolutionizing various aspects of medical research, diagnosis, and treatment. In the context of vaccine safety, AI plays a crucial role in improving signal detection — the process of identifying potential safety concerns or adverse events associated with vaccines. This blog explores how AI transforms signal detection for vaccines, its applications, benefits, challenges, and implications for enhancing safety monitoring and public health outcomes.
1. Introduction to AI in Healthcare and Vaccine Safety:
Artificial Intelligence encompasses algorithms and computational models that mimic human intelligence to analyze data, derive insights, and make predictions. In healthcare, AI applications range from diagnostic imaging and personalized medicine to drug discovery and safety surveillance, including vaccine safety.
2. Importance of Signal Detection in Vaccine Safety:
Signal detection in vaccine safety involves identifying potential associations between vaccines and adverse events following immunization (AEFI). It plays a critical role in ensuring the ongoing safety and efficacy of vaccines administered to populations worldwide.
Key Objectives:
Early Identification: Detecting safety signals early to mitigate potential risks and inform timely interventions.
Risk Assessment: Assessing the severity and likelihood of adverse events associated with specific vaccines or demographic groups.
Public Health Surveillance: Monitoring vaccine safety in real-time to maintain public confidence and support immunization programs.
3. How AI Transforms Signal Detection for Vaccines:
Artificial Intelligence enhances signal detection capabilities through advanced analytics, machine learning algorithms, and data-driven approaches:
a. Predictive Analytics:
Risk Prediction: AI models analyze historical data to predict the likelihood of adverse events based on demographic, clinical, and vaccine-related factors.
Early Warning Systems: AI-driven predictive analytics enable early identification of potential safety signals before they escalate.
b. Pattern Recognition:
Identifying Complex Patterns: AI algorithms can detect subtle, complex patterns in adverse event data that may indicate safety concerns or emerging risks.
Cluster Analysis: Grouping similar adverse events to identify clusters that suggest common underlying causes or risk factors.
c. Natural Language Processing (NLP):
Text Mining: NLP techniques extract valuable information from unstructured data sources, such as electronic health records (EHRs) or social media, to enhance signal detection.
Semantic Analysis: Analyzing patient narratives and healthcare provider notes to identify potential adverse events and improve reporting accuracy.
4. Applications of AI in Vaccine Safety Signal Detection:
AI-driven technologies are applied across various stages of vaccine safety surveillance and signal detection:
a. Real-Time Surveillance:
Continuous Monitoring: AI algorithms analyze incoming data in real-time from diverse sources, including healthcare facilities, public health databases, and social media platforms.
Automated Alerts: AI-powered systems generate automated alerts for potential safety signals, enabling rapid response and investigation.
b. Pharmacovigilance and Adverse Event Reporting:
Automated Adverse Event Detection: AI tools automate the detection and classification of adverse events from large volumes of structured and unstructured data.
Signal Triaging: Prioritizing signals based on severity, frequency, and potential impact to facilitate timely regulatory actions and risk communication.
c. Vaccine Effectiveness Studies:
Comparative Effectiveness Research: AI facilitates large-scale comparative studies to evaluate vaccine effectiveness, safety profiles, and long-term outcomes across diverse populations.
Risk-Benefit Analysis: AI models assess the overall risk-benefit ratio of vaccines to guide immunization strategies and public health policies.
5. Challenges and Considerations:
Despite its transformative potential, integrating AI into vaccine safety signal detection poses several challenges:
a. Data Quality and Integration:
Ensuring the accuracy, completeness, and interoperability of data from disparate sources (e.g., EHRs, registries) to maintain reliable AI models.
Addressing biases and variability in data reporting practices among healthcare providers and reporting systems.
b. Interpretability and Transparency:
AI models often lack transparency in decision-making processes, making it challenging to interpret the rationale behind predictions or recommendations.
Enhancing explain ability and accountability in AI-driven signal detection to build trust among healthcare professionals, regulators, and the public.
c. Regulatory and Ethical Considerations:
Adhering to regulatory guidelines (e.g., FDA, EMA) for AI applications in healthcare and pharmacovigilance to ensure compliance and patient safety.
Safeguarding patient privacy, confidentiality, and data security in AI-driven surveillance and signal detection systems.
6. Future Directions and Innovations:
The future of AI in vaccine safety signal detection is promising, with ongoing innovations and advancements:
a. Enhanced Data Analytics:
Integration of advanced analytics, such as deep learning and reinforcement learning, to improve the accuracy and efficiency of signal detection.
Utilization of big data analytics and cloud computing for scalable and real-time surveillance of vaccine safety.
b. AI-Driven Surveillance Platforms:
Development of integrated AI platforms for global vaccine safety surveillance, facilitating data sharing, collaboration, and harmonization across regions.
Implementation of AI-powered dashboards and visualization tools for intuitive data analysis and decision support.
c. Ethical AI Frameworks:
Integration of ethical AI frameworks and governance principles to ensure responsible use of AI in vaccine safety surveillance and public health interventions.
Engagement with stakeholders, including patients, healthcare providers, and policymakers, to promote transparency and accountability in AI-driven signal detection.
7. Case Studies and Success Stories:
Explore case studies and success stories where AI has been successfully implemented to enhance vaccine safety signal detection:
Global Vaccine Safety Network (GVSN): Highlight collaborations and initiatives within the GVSN leveraging AI for improved signal detection and safety monitoring.
Real-World Impact: Discuss tangible outcomes and benefits of AI-driven signal detection in supporting evidence-based decision-making and public health interventions.
Conclusion:
Artificial Intelligence is transforming vaccine safety signal detection by enhancing the speed, accuracy, and efficiency of adverse event monitoring and surveillance. By harnessing AI-driven technologies, healthcare systems can detect potential safety signals early, assess risks effectively, and ensure the ongoing safety and efficacy of vaccines for global populations. As AI continues to evolve, its integration into vaccine safety surveillance represents a significant step toward advancing public health and enhancing confidence in immunization programs worldwide.
Comments