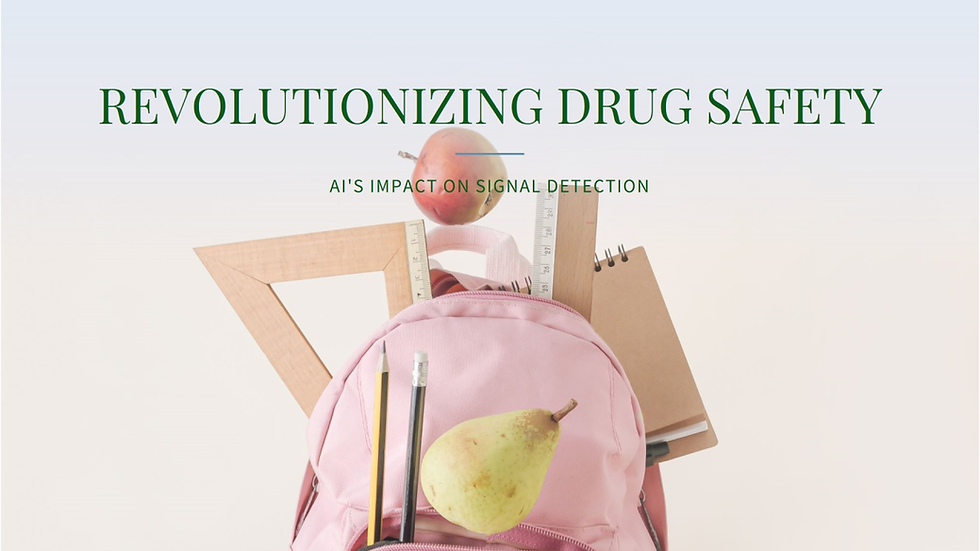
The rapid advancement of Artificial Intelligence (AI) is revolutionizing various industries, and one of the critical areas benefiting from AI is pharmacovigilance, particularly in the realm of signal detection in drug safety databases. Signal detection refers to the identification of potential safety concerns associated with pharmaceuticals, which is essential for protecting public health. This blog explores how AI is transforming signal detection in drug safety databases, highlighting the significant improvements and future potential of this technology.
Understanding Signal Detection in Drug Safety:
Signal detection in pharmacovigilance involves the identification of new, previously unknown, or changing safety information about pharmaceutical products. It is a crucial process for the early detection of adverse drug reactions (ADRs) and other drug-related risks. Traditional methods of signal detection rely heavily on manual reviews and statistical algorithms, which can be time-consuming and prone to human error.
Key challenges in traditional signal detection include:
Data Overload: The vast amount of data generated from clinical trials, post-marketing surveillance, and spontaneous reporting systems can overwhelm traditional methods.
Complexity of Data: Data from various sources such as electronic health records, patient registries, and social media are often unstructured and complex.
Latency: The time taken to identify and act upon potential signals can delay important safety interventions.
AI and machine learning (ML) technologies offer powerful tools to address these challenges, making signal detection more efficient, accurate, and timely.
The Role of AI in Signal Detection:
AI, particularly machine learning, enhances signal detection in several ways:
1. Data Integration and Processing
AI algorithms excel at integrating and processing large volumes of heterogeneous data. This capability is critical in pharmacovigilance, where data comes from diverse sources like:
Spontaneous Reporting Systems: Databases where healthcare professionals and consumers report adverse events.
Electronic Health Records (EHRs): Digital versions of patients’ paper charts.
Clinical Trials: Structured and semi-structured data from clinical studies.
Social Media and Patient Forums: Unstructured data that can provide early signals of adverse effects.
AI can harmonize these data sources, ensuring that relevant information is extracted and standardized for further analysis.
2. Automated Signal Detection
AI can automate the detection of signals by using sophisticated algorithms to identify patterns and anomalies in the data. Machine learning models can be trained to recognize the characteristics of adverse drug reactions, enabling:
Pattern Recognition: Detecting unusual patterns that may indicate a potential safety signal.
Anomaly Detection: Identifying outliers and unexpected occurrences that warrant further investigation.
Natural Language Processing (NLP): Analyzing textual data from medical literature, EHRs, and social media to identify potential safety concerns.
3. Enhanced Predictive Analytics
Machine learning models, particularly those employing deep learning techniques, can predict the likelihood of adverse drug reactions. These models can analyze historical data to forecast potential risks, allowing for proactive risk management. Key approaches include:
Supervised Learning: Training models on labeled data to predict known adverse events.
Unsupervised Learning: Identifying previously unknown patterns without pre-labeled data.
Reinforcement Learning: Continuously improving models based on new data and outcomes.
4. Real-time Monitoring and Alerts
AI-powered systems can provide real-time monitoring and alerts, significantly reducing the latency between signal detection and intervention. These systems continuously analyze incoming data, providing immediate alerts when potential signals are detected. This real-time capability is crucial for timely safety measures.
5. Risk Stratification and Prioritization
AI can help prioritize signals based on the severity and likelihood of risk. By stratifying risks, AI systems enable pharmacovigilance professionals to focus on the most critical signals first, optimizing resource allocation and response times.
Key AI Techniques in Signal Detection:
Several AI techniques are particularly useful in transforming signal detection in drug safety databases:
1. Machine Learning
Machine learning algorithms, such as logistic regression, decision trees, random forests, and support vector machines, are widely used for predicting adverse drug reactions. These algorithms can handle large datasets and identify complex relationships between variables.
2. Deep Learning
Deep learning, a subset of machine learning, involves neural networks with multiple layers. Techniques such as convolutional neural networks (CNNs) and recurrent neural networks (RNNs) are particularly effective for processing unstructured data like text and images.
3. Natural Language Processing (NLP)
NLP enables the extraction of meaningful information from unstructured text data. In pharmacovigilance, NLP can be used to analyze medical literature, patient forums, and social media to identify mentions of adverse drug reactions and other safety concerns.
4. Anomaly Detection
Anomaly detection algorithms identify data points that deviate significantly from the norm. These algorithms are crucial for spotting rare or unexpected adverse events in large datasets.
5. Clustering and Association Rule Learning
Clustering algorithms group similar data points together, which can help identify common patterns in adverse drug reactions. Association rule learning identifies relationships between different variables, providing insights into potential drug interactions and side effects.
Benefits of AI in Signal Detection:
The integration of AI into signal detection processes offers numerous benefits:
1. Increased Efficiency
AI automates many of the labor-intensive tasks involved in signal detection, freeing up pharmacovigilance professionals to focus on more strategic activities.
2. Improved Accuracy
AI algorithms can identify patterns and anomalies that may be missed by human analysts, reducing the likelihood of false negatives and false positives.
3. Timeliness
Real-time monitoring and alerts enable faster identification and response to potential safety signals, reducing the time between detection and intervention.
4. Scalability
AI systems can scale to handle the ever-increasing volume of data in drug safety databases, ensuring that signal detection processes remain robust and effective as data grows.
5. Enhanced Insights
By integrating data from multiple sources and employing advanced analytics, AI provides deeper insights into the safety profile of drugs, helping to identify potential risks earlier.
Challenges and Considerations:
While AI holds great promise for transforming signal detection in drug safety databases, several challenges and considerations must be addressed:
1. Data Quality and Integration
Ensuring high-quality, standardized data from diverse sources is crucial for the effectiveness of AI models. Poor data quality can lead to inaccurate predictions and unreliable insights.
2. Interpretability
AI models, particularly deep learning models, can be complex and difficult to interpret. Ensuring that AI-driven insights are transparent and understandable is important for regulatory compliance and trust.
3. Regulatory Compliance
Pharmacovigilance is a highly regulated field. AI systems must comply with regulatory requirements and guidelines, such as those set by the FDA and EMA. Ensuring compliance while leveraging AI capabilities is a critical balance.
4. Ethical Considerations
The use of AI in healthcare raises ethical considerations, including data privacy and the potential for algorithmic bias. Ensuring ethical use of AI is essential for maintaining public trust and safeguarding patient welfare.
5. Continuous Learning and Adaptation
AI models need to be continuously updated and validated with new data to remain effective. Implementing robust systems for ongoing learning and adaptation is essential for maintaining the relevance and accuracy of AI-driven signal detection.
Future Directions:
The future of AI in signal detection for drug safety databases is promising, with several emerging trends and advancements:
1. Integration of Genomic Data
Incorporating genomic data into AI models can provide personalized insights into drug safety, helping to identify individuals who may be at higher risk for adverse reactions based on their genetic profile.
2. Advanced NLP Techniques
Continued advancements in NLP, such as the development of more sophisticated language models, will enhance the ability to extract meaningful insights from unstructured text data.
3. Collaborative AI
Collaborative AI systems that involve human-AI interaction can combine the strengths of both human expertise and AI capabilities, improving the overall effectiveness of signal detection.
4. Blockchain for Data Integrity
Blockchain technology can enhance the integrity and traceability of data in pharmacovigilance, ensuring that AI models are built on reliable and tamper-proof data sources.
5. Real-world Evidence Integration
Leveraging real-world evidence, including data from wearable devices and patient-reported outcomes, can provide a more comprehensive view of drug safety and enhance the predictive power of AI models.
Conclusion:
AI is transforming signal detection in drug safety databases by making the process more efficient, accurate, and timely. By leveraging AI techniques such as machine learning, deep learning, NLP, and anomaly detection, pharmacovigilance professionals can better identify and respond to potential safety signals. While challenges such as data quality, interpretability, and regulatory compliance remain, the benefits of AI in enhancing drug safety are substantial. As technology continues to evolve, AI will play an increasingly vital role in ensuring the safety and well-being of patients worldwide.
Comments