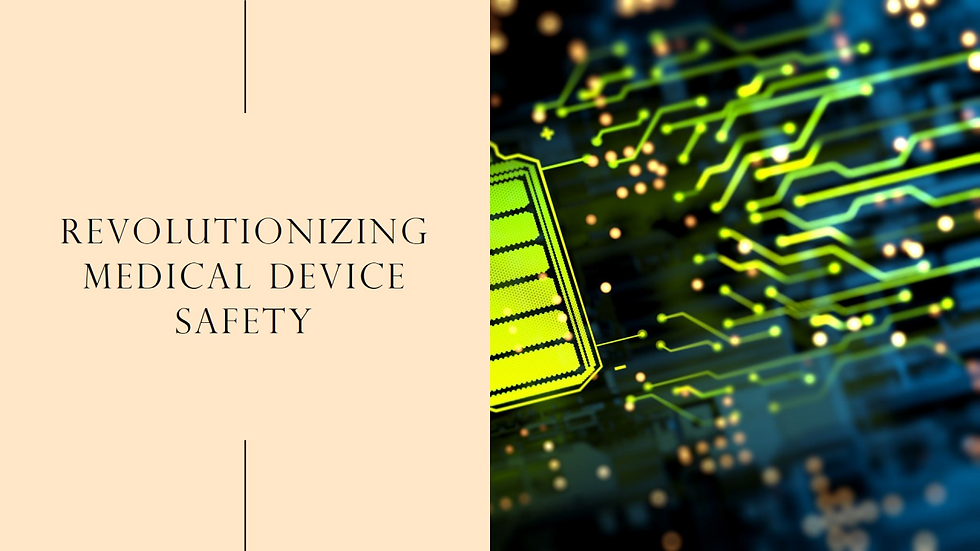
In the realm of healthcare, medical devices play a crucial role in diagnosing, monitoring, and treating various medical conditions. Ensuring their safety and efficacy is paramount to safeguarding patient health. Artificial Intelligence (AI) is revolutionizing signal evaluation in medical device safety by enhancing the detection, analysis, and response to potential safety issues. This blog explores the transformative impact of AI on signal evaluation in medical device safety, its benefits, challenges, and future implications for healthcare.
Understanding Signal Evaluation in Medical Device Safety:
Signal evaluation involves the systematic assessment of data to identify potential safety signals associated with medical devices:
Adverse Events: Monitoring and analyzing adverse events reported by healthcare providers and patients.
Post-Market Surveillance: Conducting ongoing surveillance to detect safety concerns after device approval and deployment.
Signal Detection: Identifying patterns or trends that may indicate safety issues requiring further investigation.
Role of Artificial Intelligence in Signal Evaluation:
1. Enhanced Data Analysis
AI-driven algorithms improve the efficiency and accuracy of data analysis:
Pattern Recognition: Identifying subtle patterns or anomalies in large datasets that may indicate safety signals.
Natural Language Processing (NLP): Analyzing unstructured data from medical records, patient reports, and social media.
2. Real-Time Monitoring
AI enables continuous and real-time monitoring of device safety:
Automated Surveillance: Monitoring adverse events and safety signals in real-time for prompt intervention.
Predictive Analytics: Forecasting potential safety issues based on historical data and risk factors.
3. Early Detection of Safety Signals
AI algorithms facilitate early detection of emerging safety concerns:
Alert Systems: Generating alerts for potential safety signals that require immediate attention.
Risk Prediction: Predicting adverse events before they occur based on patient data and device usage patterns.
Benefits of AI in Medical Device Safety Signal Evaluation:
1. Timely Intervention
AI enables faster identification and response to safety signals:
Reduced Response Time: Minimizing the time between signal detection and regulatory action.
Enhanced Patient Safety: Improving outcomes by addressing safety concerns promptly.
2. Improved Accuracy and Efficiency
AI-driven analysis enhances the accuracy and efficiency of signal evaluation:
High Precision: Identifying relevant safety signals with greater accuracy than traditional methods.
Scalability: Handling large volumes of data efficiently for comprehensive surveillance.
3. Enhanced Regulatory Compliance
Meeting regulatory requirements for device safety reporting:
FDA Guidelines: Complying with U.S. Food and Drug Administration (FDA) regulations for medical device safety.
EMA Standards: Adhering to European Medicines Agency (EMA) standards for post-market surveillance.
Challenges and Considerations:
1. Data Quality and Integration
Ensuring the reliability and interoperability of data from diverse sources:
Standardization: Establishing uniform data formats and coding systems for consistent analysis.
Integration Challenges: Integrating data from electronic health records (EHRs), device registries, and other sources.
2. Interpretability of AI Models
Understanding and interpreting AI-generated insights for clinical decision-making:
Transparency: Ensuring transparency in AI algorithms and decision processes.
Validation: Validating AI models to ensure accuracy and reliability in signal evaluation.
3. Ethical and Legal Implications
Addressing ethical considerations in AI-driven signal evaluation:
Privacy: Protecting patient confidentiality and data privacy in medical device surveillance.
Bias Mitigation: Minimizing biases in AI algorithms that could impact signal detection and analysis.
Case Studies and Examples:
1. FDA Sentinel Initiative
The FDA utilizes AI for signal evaluation in the Sentinel Initiative:
Data Analytics: Applying AI to analyze real-world data for medical device safety surveillance.
Collaborative Networks: Partnering with healthcare organizations to enhance signal detection capabilities.
2. Medical Device Registries
National and international registries leverage AI for comprehensive signal evaluation:
Longitudinal Data Analysis: Tracking device performance and safety outcomes over time.
Outcome Measures: Assessing real-world effectiveness and safety based on registry data.
Future Directions in AI-Enhanced Signal Evaluation:
1. Integration with Real-World Evidence (RWE)
Harnessing AI to integrate real-world data for signal evaluation:
RWE Databases: Using electronic health records and patient registries to enhance data analysis.
Predictive Modeling: Developing predictive models to anticipate safety signals based on patient characteristics and device usage.
2. Blockchain and Secure Data Management
Exploring blockchain technology for secure and transparent data management:
Data Integrity: Ensuring immutable records and traceability in medical device surveillance.
Decentralized Platforms: Facilitating data sharing while protecting patient privacy and confidentiality.
Conclusion:
Artificial Intelligence is transforming signal evaluation in medical device safety by enhancing data analysis, enabling real-time monitoring, and facilitating early detection of safety signals. Despite challenges such as data quality, interpretability of AI models, and ethical considerations, the benefits of AI-driven signal evaluation are clear. Embracing technological innovations and collaborative efforts will pave the way for more efficient and effective medical device surveillance systems, ensuring patient safety and regulatory compliance in healthcare worldwide. As AI continues to evolve, its role in signal evaluation will become increasingly integral to advancing public health and enhancing the quality of medical care.
Comments