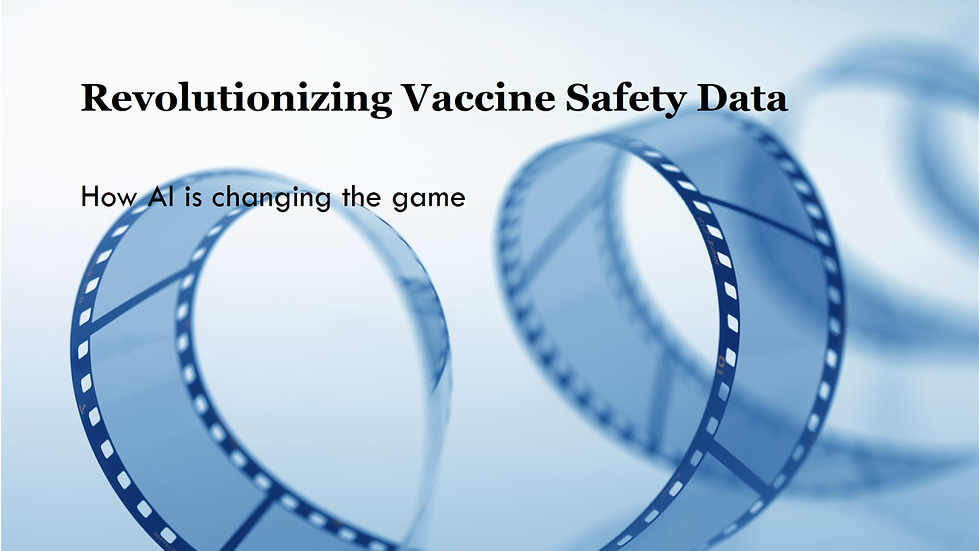
Artificial Intelligence (AI) is revolutionizing the aggregation and analysis of vaccine safety data, enhancing surveillance capabilities and improving public health outcomes. This blog explores how AI transforms vaccine safety data aggregation, its applications, benefits, challenges, and future implications.
Introduction to AI in Vaccine Safety Data Aggregation:
AI technologies are reshaping how vaccine safety data is aggregated, analyzed, and utilized by healthcare systems and regulatory agencies. By leveraging machine learning algorithms, natural language processing (NLP), and predictive analytics, AI enables faster detection of adverse events following immunization (AEFI) and enhances the efficiency of vaccine safety monitoring processes.
Benefits of AI in Vaccine Safety Data Aggregation:
1. Early Signal Detection
Real-time Monitoring: AI algorithms can analyze large datasets in real-time, detecting potential safety signals faster than traditional methods.
Anomaly Detection: Identify unexpected patterns or correlations that may indicate adverse reactions to vaccines, enabling prompt investigation.
2. Improved Accuracy and Efficiency
Automated Data Processing: AI automates data aggregation and analysis tasks, reducing human error and streamlining surveillance workflows.
Enhanced Predictive Modeling: Predict future trends in vaccine safety based on historical data and epidemiological factors.
3. Enhanced Surveillance Capabilities
Scalability: AI systems can scale to process vast amounts of data from diverse sources, including electronic health records (EHRs) and pharmacovigilance databases.
Global Integration: Facilitate data sharing and collaboration among international healthcare organizations and regulatory agencies.
Applications of AI in Vaccine Safety Data Aggregation:
1. Automated Adverse Event Detection
Pattern Recognition: Identify patterns of symptoms or adverse reactions that may indicate potential AEFI.
Natural Language Processing: Analyze unstructured data from medical reports, social media, and online forums to detect early signals.
2. Vaccine Effectiveness Assessment
Epidemiological Studies: Analyze population-level data to assess vaccine effectiveness and safety profiles across different demographics.
Comparative Analysis: Compare outcomes of vaccinated versus unvaccinated populations to evaluate vaccine efficacy.
3. Predictive Analytics
Forecasting Outbreaks: Predict and prepare for potential disease outbreaks based on vaccination coverage and AEFI surveillance data.
Risk Stratification: Identify high-risk groups or regions susceptible to adverse reactions, guiding targeted vaccination strategies.
Challenges and Considerations:
1. Data Quality and Standardization
Data Integration: Ensure interoperability and compatibility of data sources for accurate aggregation and analysis.
Quality Assurance: Address biases and inconsistencies in data collection to improve the reliability of AI-driven insights.
2. Ethical and Privacy Concerns
Data Privacy: Protect patient confidentiality and comply with regulatory requirements (e.g., GDPR, HIPAA) when handling sensitive health data.
Algorithm Transparency: Ensure transparency in AI algorithms to maintain trust and accountability in vaccine safety monitoring.
3. Regulatory Compliance
Validation and Verification: Validate AI models for accuracy and reliability in detecting AEFI before widespread implementation.
Regulatory Approval: Obtain regulatory approval for AI-driven systems used in vaccine safety monitoring to ensure adherence to safety standards.
Future Directions and Innovations:
1. Advanced Machine Learning Techniques
Deep Learning: Apply deep neural networks to enhance pattern recognition and anomaly detection in vaccine safety data.
Reinforcement Learning: Develop AI systems that learn and adapt in real-time based on feedback from surveillance data.
2. Blockchain and Decentralized Systems
Data Security: Utilize blockchain technology for secure, immutable data storage and transparent tracking of vaccine safety records.
Smart Contracts: Automate data sharing agreements and ensure compliance with privacy regulations through smart contract protocols.
3. Integration with Digital Health Platforms
IoT Devices: Integrate data from IoT devices and wearables to monitor vaccine responses and detect early signs of adverse reactions.
Telehealth Solutions: Utilize telehealth platforms for remote monitoring and reporting of AEFIs, enhancing accessibility and timely intervention.
Case Studies and Success Stories:
1. FDA Sentinel Initiative
Use Case: Leveraging AI and big data analytics to monitor vaccine safety through the FDA Sentinel System, enhancing post-market surveillance capabilities.
Impact: Rapid detection of safety signals and evidence-based regulatory decisions to protect public health.
2. WHO Global Vaccine Safety Initiative
Use Case: Applying AI-driven surveillance systems to monitor vaccine safety across multiple countries, improving global collaboration and data sharing.
Impact: Strengthened vaccine safety monitoring frameworks and accelerated response to emerging health threats.
Conclusion:
AI is transforming vaccine safety data aggregation by enhancing early detection, accuracy, and efficiency in monitoring adverse events following immunization. By harnessing machine learning, predictive analytics, and advanced technologies, healthcare systems and regulatory agencies can improve surveillance capabilities, inform evidence-based decision-making, and enhance public trust in vaccination programs. As AI continues to evolve, its integration into vaccine safety monitoring holds promise for advancing global health outcomes and ensuring the safety and efficacy of vaccines for all populations.
Comments