How Innovations in Signal Detection Improve Drug Safety
- Chaitali Gaikwad
- Aug 20, 2024
- 6 min read
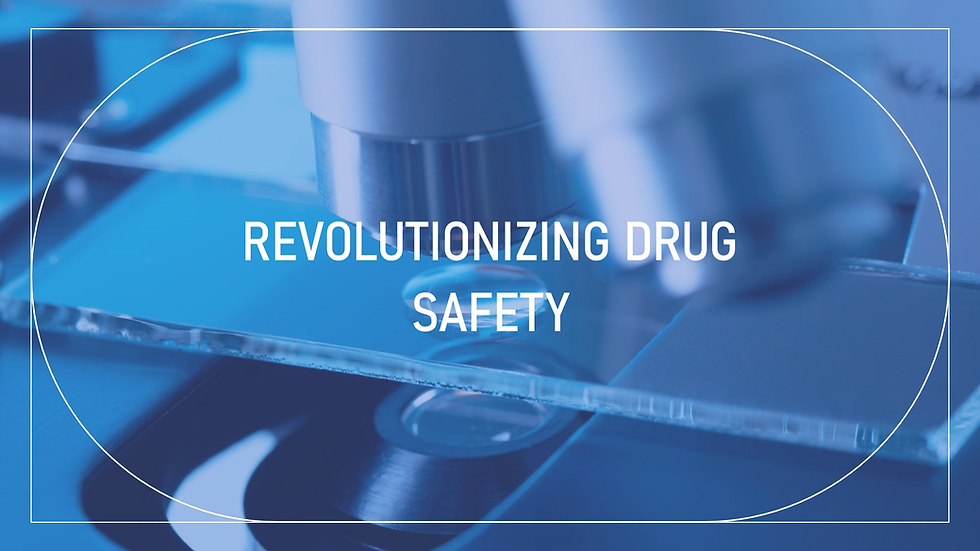
Drug safety is a critical component of public health, ensuring that medications used by millions of people worldwide are effective and free from harmful side effects. One of the key processes in maintaining drug safety is signal detection, which involves identifying potential safety issues related to pharmaceutical products. Recent innovations in signal detection have significantly improved the ability to monitor, identify, and mitigate drug safety risks, ultimately leading to better patient outcomes and enhanced public health.
In this blog, we will explore the advancements in signal detection technologies and methodologies, their impact on pharmacovigilance, and how they are shaping the future of drug safety.
Understanding Signal Detection in Pharmacovigilance:
Signal detection in pharmacovigilance refers to the process of identifying new safety information or risks associated with a pharmaceutical product. This process involves analyzing data from various sources, including spontaneous reports, clinical trials, literature, and electronic health records, to detect patterns or trends that may indicate a potential safety concern.
A "signal" is considered to be any information that suggests a new potential association between a drug and an adverse event. Signals need to be further investigated and validated to determine whether they represent a real risk that requires regulatory action, such as updating product labeling, issuing safety warnings, or even withdrawing the product from the market.
The Role of Signal Detection in Drug Safety:
Signal detection plays a pivotal role in drug safety by enabling early identification of potential risks. It allows regulatory authorities, pharmaceutical companies, and healthcare professionals to take proactive measures to protect patients from harm. The traditional approach to signal detection has relied heavily on manual review and analysis of spontaneous adverse event reports, which can be time-consuming and limited by the quality and completeness of the data.
However, the growing volume of data and the complexity of modern pharmaceuticals have necessitated more sophisticated methods for signal detection. Innovations in technology and data science have paved the way for advanced signal detection systems that are more accurate, efficient, and capable of handling large datasets.
Innovations in Signal Detection Technologies:
Artificial Intelligence and Machine Learning
Artificial Intelligence (AI) and Machine Learning (ML) have revolutionized the field of signal detection by automating and enhancing the analysis of large datasets. These technologies can identify patterns and correlations in data that might be missed by traditional methods. AI and ML algorithms can process vast amounts of information from various sources, such as electronic health records, social media, and literature, to detect potential safety signals in real-time.
Natural Language Processing (NLP): NLP, a subset of AI, enables the extraction of meaningful information from unstructured text data, such as medical records and scientific literature. NLP algorithms can analyze adverse event reports and identify potential signals more efficiently than manual methods.
Predictive Analytics: Machine learning models can predict the likelihood of adverse events based on historical data and patient characteristics. This allows for the identification of at-risk populations and the early detection of potential safety issues
Big Data and Data Mining
The advent of big data has transformed signal detection by providing access to a vast amount of information from diverse sources. Data mining techniques allow for the systematic analysis of large datasets to identify patterns, trends, and correlations that may indicate potential safety signals.
Integrated Databases: The integration of various data sources, such as electronic health records, clinical trial data, and post-marketing surveillance reports, enables a more comprehensive analysis of drug safety. These integrated databases can be mined to identify rare adverse events and long-term safety concerns that may not be evident in smaller datasets.
Real-World Evidence (RWE): The use of real-world data, such as electronic health records and insurance claims, provides valuable insights into the safety and effectiveness of drugs in everyday clinical practice. Data mining techniques can be applied to RWE to detect signals that may not have been identified in controlled clinical trials.
Signal Detection Algorithms
Advanced signal detection algorithms have been developed to improve the accuracy and efficiency of identifying potential safety signals. These algorithms use statistical methods to analyze data and identify signals that warrant further investigation.
Bayesian Data Mining: Bayesian data mining techniques, such as the Bayesian Confidence Propagation Neural Network (BCPNN), are used to calculate the strength of the association between a drug and an adverse event. These methods take into account prior knowledge and the likelihood of observing the data under different hypotheses.
Proportional Reporting Ratios (PRR): PRR is a commonly used statistical method in signal detection that compares the observed frequency of an adverse event for a specific drug with the expected frequency based on all other drugs in the database. A higher-than-expected PRR may indicate a potential safety signal.
Automated Signal Detection Systems
The development of automated signal detection systems has streamlined the process of identifying potential safety issues. These systems use advanced algorithms and machine learning to continuously monitor and analyze data, providing real-time alerts when potential signals are detected.
Vii Base: Vii Base is the World Health Organization's global database of individual case safety reports. It uses automated signal detection algorithms to identify potential safety signals from reports submitted by member countries. The system continuously monitors the database and generates signals for further evaluation.
FAERS (FDA Adverse Event Reporting System): The FDA's FAERS database is another example of an automated signal detection system. It collects adverse event reports and uses statistical methods to detect potential safety signals. The system generates reports that are reviewed by FDA analysts to determine whether further investigation is needed.
The Impact of Innovations in Signal Detection on Drug Safety:
The innovations in signal detection technologies have had a profound impact on drug safety. These advancements have led to more accurate and timely identification of potential safety issues, allowing for quicker regulatory action and improved patient safety.
Early Detection of Safety Signals
The use of AI, machine learning, and advanced algorithms has significantly reduced the time it takes to detect safety signals. Early detection allows for prompt investigation and intervention, minimizing the risk of harm to patients. This is particularly important for identifying rare or serious adverse events that may not be apparent during clinical trials.
Improved Accuracy and Precision
Innovations in signal detection have improved the accuracy and precision of identifying potential safety signals. Machine learning models and data mining techniques can analyze large datasets with greater accuracy, reducing the likelihood of false positives and ensuring that true signals are not missed.
Enhanced Post-Marketing Surveillance
The integration of real-world data and automated signal detection systems has enhanced post-marketing surveillance of pharmaceutical products. These systems can continuously monitor drug safety in real-time, providing ongoing assessment of a drug's risk-benefit profile throughout its lifecycle.
Informed Regulatory Decisions
The ability to detect and analyze safety signals more effectively has informed regulatory decisions and actions. Regulatory authorities can use the insights gained from advanced signal detection methods to make data-driven decisions regarding product labeling, safety warnings, and market withdrawals.
Personalized Medicine and Risk Management
Innovations in signal detection have also contributed to the development of personalized medicine. By identifying specific patient populations that are at higher risk for adverse events, healthcare providers can tailor treatments to individual patients, improving safety and outcomes.
Challenges and Future Directions:
While the innovations in signal detection have significantly improved drug safety, there are still challenges to overcome. The quality and completeness of data, the need for standardization across different systems, and the ethical considerations of using AI and machine learning in pharmacovigilance are ongoing challenges.
Future directions in signal detection may include the development of more sophisticated AI models that can predict safety signals before they occur, the integration of more diverse data sources, such as genetic and environmental data, and the advancement of personalized pharmacovigilance, where safety monitoring is tailored to individual patients based on their unique characteristics.
Conclusion:
Innovations in signal detection are revolutionizing the field of pharmacovigilance and improving drug safety. The integration of AI, machine learning, big data, and advanced algorithms has enhanced the ability to detect and analyze safety signals, leading to more accurate and timely identification of potential risks. These advancements have not only improved patient safety but also informed regulatory decisions and contributed to the development of personalized medicine.
As technology continues to evolve, the future of signal detection in drug safety looks promising. By embracing these innovations, the pharmaceutical industry and regulatory authorities can ensure that the medications used by patients worldwide are as safe and effective as possible, ultimately protecting public health and saving lives.
Comments