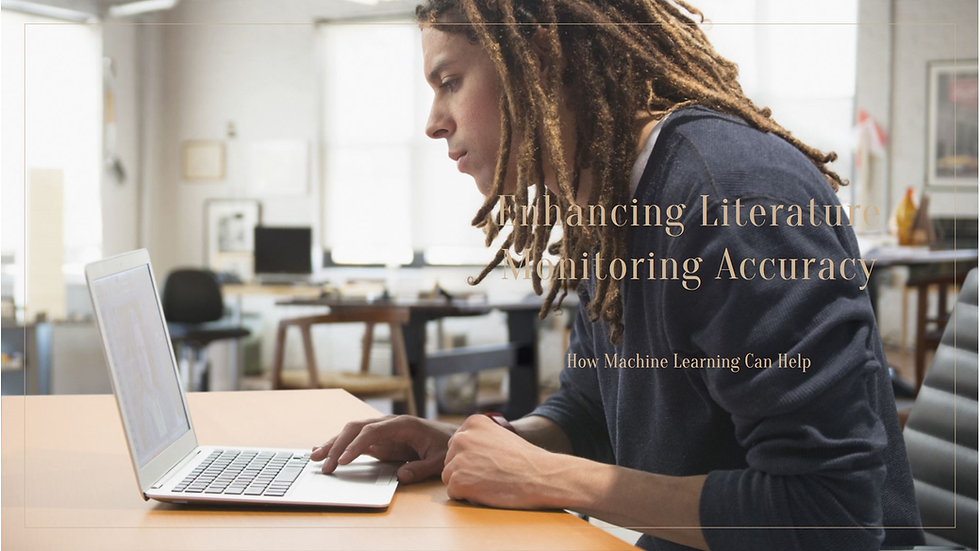
In today's rapidly evolving medical and pharmaceutical fields, staying current with emerging research is vital for ensuring the safety and efficacy of treatments, including drugs, vaccines, and medical devices. One of the critical components of this vigilance is literature monitoring, which involves reviewing and analyzing medical publications for safety signals, adverse events, and relevant findings. However, manual literature review is time-consuming, prone to human error, and increasingly impractical given the sheer volume of scientific papers published daily.
Machine learning (ML), a subset of artificial intelligence (AI), is transforming literature monitoring by automating the process, increasing accuracy, and ensuring that critical information is not overlooked. This blog will explore how machine learning enhances literature monitoring accuracy, providing benefits such as increased efficiency, improved safety, and better decision-making.
The Challenges of Manual Literature Monitoring
Manual literature monitoring, traditionally conducted by teams of experts, involves screening thousands of publications, extracting relevant information, and assessing the potential impact of new findings. While this approach has been the standard for years, it comes with several challenges:
Volume of Publications: The exponential growth in the number of medical research articles makes it impossible for humans to keep pace. According to a report by PubMed, over 1 million new medical research articles are published annually.
Inconsistency: Human reviewers may interpret and categorize information differently, leading to inconsistent results. This is especially true when multiple teams work on the same literature.
Time Constraints: Manually reviewing literature takes a significant amount of time, delaying critical decision-making.
Data Overload: The abundance of data can overwhelm even the most experienced reviewers, leading to missed safety signals or relevant findings.
These challenges emphasize the need for more efficient and accurate solutions, which is where machine learning comes in.
What Is Machine Learning in Literature Monitoring?
Machine learning is an AI technique that enables systems to learn from data and improve their performance over time. In the context of literature monitoring, ML algorithms are trained on vast datasets of medical literature to identify relevant patterns, extract information, and classify data with minimal human intervention.
The goal of using ML in literature monitoring is to automate tasks such as identifying adverse events, extracting key insights, and categorizing articles based on their relevance to a specific query or safety concern. By doing so, ML improves accuracy and efficiency while reducing the workload for human reviewers.
How Machine Learning Enhances Accuracy in Literature Monitoring
1. Efficient Data Processing and Categorization
Machine learning algorithms are adept at processing large volumes of data quickly and accurately. In literature monitoring, ML can sift through thousands of research articles, abstracts, and reports in a fraction of the time it would take a human reviewer. By using natural language processing (NLP) and text mining techniques, ML systems can extract key pieces of information such as:
Drug names
Adverse event terms
Study outcomes
Dosages and side effects
Safety signals
ML models can categorize and tag articles based on predefined criteria such as relevance to pharmacovigilance, therapeutic area, or adverse event reporting. This automation not only increases accuracy but also reduces the likelihood of human error or oversight in the screening process.
2. Reducing False Positives and False Negatives
In manual literature monitoring, false positives (incorrectly identifying a finding as relevant) and false negatives (failing to identify a relevant finding) are common issues. Machine learning can significantly reduce these errors by refining search parameters and learning from past data.
ML models are trained on historical data, meaning they can "learn" what constitutes a relevant or irrelevant article based on previous findings. This allows for more accurate filtering, reducing the number of irrelevant articles flagged for review and ensuring that important safety signals are not missed.
3. Continuous Learning and Improvement
One of the most significant advantages of machine learning in literature monitoring is its ability to improve over time. Unlike traditional rule-based systems, ML models can evolve and adapt to new information. As more data is processed, the algorithms become better at recognizing patterns, identifying relevant findings, and filtering out irrelevant content.
For example, if a new type of adverse event is discovered in relation to a specific drug, the ML model can be retrained to recognize this signal in future publications. This continuous learning ensures that literature monitoring systems remain up-to-date and accurate, even as medical knowledge evolves.
4. Enhanced Natural Language Processing (NLP)
Natural language processing, a critical component of machine learning, allows computers to understand and interpret human language. In literature monitoring, NLP is used to analyze unstructured text data from research articles, extracting relevant information such as adverse events, treatment outcomes, and safety signals.
Advanced NLP techniques, such as named entity recognition (NER) and sentiment analysis, enable ML systems to identify key entities (e.g., drug names, patient populations) and assess the overall sentiment of a study (e.g., positive, negative, or neutral). This enhances the accuracy of literature monitoring by ensuring that all relevant information is captured and classified correctly.
For example, an NLP-powered ML model can read through an article about a clinical trial, identify the drugs involved, detect any reported adverse events, and classify the findings based on their relevance to pharmacovigilance. This level of precision is difficult to achieve with manual review alone.
5. Improved Signal Detection
Detecting safety signals—indications of potential adverse effects or risks associated with a drug or vaccine—is a critical aspect of literature monitoring. Machine learning enhances signal detection by identifying patterns and trends across multiple publications that may not be immediately obvious to human reviewers.
For instance, an ML model can analyze a large dataset of clinical trial reports and patient records, cross-referencing adverse event terms with specific drugs or treatments. By identifying correlations between these variables, the system can flag potential safety signals for further investigation. This proactive approach reduces the likelihood of missing critical findings that could affect patient safety.
6. Automation of Routine Tasks
One of the primary benefits of machine learning in literature monitoring is its ability to automate routine tasks, freeing up human experts to focus on more complex and high-value activities. Tasks such as screening articles, extracting key data points, and categorizing publications can be fully automated using ML algorithms.
Automation not only improves accuracy but also ensures that literature monitoring is conducted consistently and efficiently. By eliminating the need for manual data entry and classification, ML reduces the risk of human error and allows organizations to scale their literature monitoring efforts without increasing resource requirements.
7. Real-Time Monitoring and Updates
With the volume of medical literature increasing daily, it's crucial for organizations to stay updated with the latest research. Machine learning systems can be integrated with literature monitoring platforms to provide real-time updates on newly published articles that are relevant to specific drugs, adverse events, or therapeutic areas.
This real-time monitoring ensures that organizations are always aware of emerging safety signals or new findings that could impact their products. ML systems can automatically flag new publications for review, providing timely insights and allowing for quicker decision-making.
8. Enhancing Compliance with Regulatory Requirements
Regulatory authorities such as the FDA, EMA, and WHO require pharmaceutical companies to monitor the literature for adverse events and safety signals continuously. Machine learning enhances compliance by ensuring that all relevant literature is screened and analyzed accurately, reducing the risk of non-compliance.
ML systems can be configured to adhere to specific regulatory requirements, ensuring that literature monitoring is conducted in line with international guidelines. Additionally, automated reporting features can generate compliance reports, providing a clear audit trail of literature monitoring activities.
9. Enabling Collaboration and Knowledge Sharing
In large organizations, literature monitoring often involves multiple teams, including pharmacovigilance, clinical research, and regulatory affairs. Machine learning platforms facilitate collaboration by providing a centralized system where data can be shared and reviewed across teams.
ML-enhanced literature monitoring platforms can integrate with existing pharmacovigilance systems, allowing different departments to access relevant findings, review flagged articles, and contribute to signal detection efforts. This collaborative approach ensures that all stakeholders are informed and that decision-making is based on the most accurate and up-to-date information.
Future of Machine Learning in Literature Monitoring
The future of literature monitoring will likely see further advancements in machine learning and AI technologies. As these systems become more sophisticated, they will be able to process even larger volumes of data, extract more nuanced insights, and provide predictive analytics to identify potential safety risks before they manifest in clinical practice.
For example, future ML models may incorporate predictive modeling techniques to forecast the likelihood of adverse events based on historical data. This would enable proactive risk management, allowing pharmaceutical companies and regulatory bodies to take preventative measures before safety concerns become widespread.
Additionally, advancements in NLP and text mining will continue to improve the accuracy of literature monitoring, ensuring that all relevant information is captured and analyzed in real-time.
Conclusion
Machine learning is revolutionizing literature monitoring by automating the review process, increasing accuracy, and enhancing the ability to detect safety signals and adverse events. By reducing human error, improving data processing capabilities, and enabling real-time monitoring, ML systems are transforming how organizations stay current with the latest medical research and ensure the safety of their products.
As the volume of published medical literature continues to grow, adopting machine learning in literature monitoring will become essential for pharmaceutical companies, healthcare providers, and regulatory bodies alike. The ability to accurately and efficiently analyze vast amounts of data is crucial for maintaining patient safety and meeting regulatory requirements in an increasingly complex healthcare landscape.
Comments