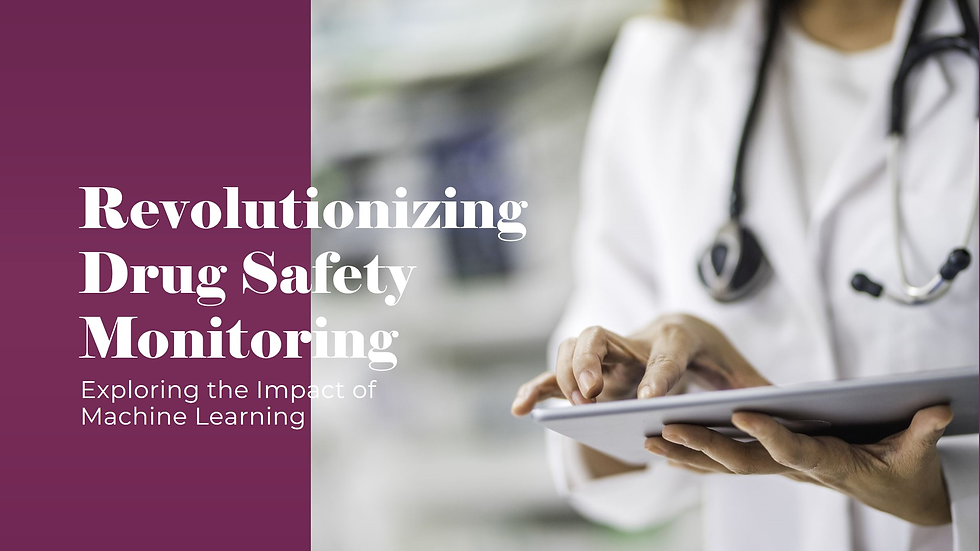
Drug safety monitoring, or pharmacovigilance, is a critical aspect of healthcare that ensures the safety and efficacy of pharmaceutical products. Traditionally, this process has relied on manual data collection, analysis, and reporting, which can be time-consuming and prone to human error. However, the advent of machine learning (ML) is revolutionizing drug safety monitoring, offering new ways to analyze vast amounts of data quickly and accurately. This blog explores how machine learning impacts drug safety monitoring, the benefits it brings, the challenges it poses, and the future of this transformative technology in healthcare.
Understanding Machine Learning and Drug Safety Monitoring:
Machine Learning (ML) is a subset of artificial intelligence (AI) that focuses on building systems that can learn from and make decisions based on data. By analyzing large datasets, ML algorithms can identify patterns, make predictions, and even adapt to new data inputs without explicit programming. These capabilities make ML particularly well-suited for handling complex tasks in drug safety monitoring.
Drug safety monitoring involves the detection, assessment, understanding, and prevention of adverse effects or any other drug-related problems. This process is essential for ensuring that the benefits of a drug outweigh its risks. Traditionally, drug safety monitoring has relied on clinical trials, post-marketing surveillance, and spontaneous reporting systems. While effective, these methods have limitations, including delayed reporting, underreporting, and difficulties in managing large volumes of data.
Machine learning offers a powerful solution to these challenges by automating data analysis, improving the accuracy of predictions, and enabling real-time monitoring of drug safety.
The Role of Machine Learning in Drug Safety Monitoring:
Machine learning impacts drug safety monitoring in several key areas, including:
1. Adverse Drug Reaction (ADR) Detection
One of the most significant contributions of machine learning to drug safety monitoring is its ability to detect adverse drug reactions (ADRs) more quickly and accurately than traditional methods. ADRs are unwanted or harmful reactions to a drug that occur at normal doses. Early detection of ADRs is crucial for preventing harm to patients and ensuring the safe use of medications.
Machine learning algorithms can analyze large datasets from electronic health records (EHRs), social media, clinical trials, and spontaneous reporting systems to identify patterns and correlations that may indicate an ADR. For example, natural language processing (NLP) techniques can be used to analyze unstructured data, such as patient reports and social media posts, to detect mentions of potential ADRs. These algorithms can also identify rare and unexpected ADRs that might be missed by traditional methods.
By automating the detection process, machine learning reduces the time required to identify ADRs, allowing for faster intervention and improved patient safety.
2. Predictive Modeling for Drug Safety
Machine learning enables predictive modeling, which can forecast potential safety issues before they become widespread. By analyzing historical data, such as clinical trial results and post-marketing surveillance reports, machine learning models can predict which patients are at higher risk of experiencing ADRs based on factors like age, gender, genetic profile, and medical history.
These predictive models can also be used to simulate how a drug will perform in different populations, identifying potential risks and adverse effects before the drug is widely distributed. This capability is particularly valuable during the drug development process, as it allows pharmaceutical companies to make more informed decisions about whether to proceed with a drug or modify its formulation.
Predictive modeling helps in the early identification of high-risk patients and in tailoring treatment plans to minimize the risk of ADRs, ultimately improving patient outcomes.
3. Enhancing Signal Detection
Signal detection is a critical component of pharmacovigilance that involves identifying signals, or potential safety issues, from various data sources. These signals may indicate a new or previously unrecognized ADR or a change in the frequency or severity of known ADRs.
Machine learning enhances signal detection by automating the analysis of large datasets and identifying patterns that human analysts might overlook. For example, clustering algorithms can group similar ADRs together, making it easier to identify emerging safety signals. Additionally, machine learning can prioritize signals based on their potential impact, helping pharmacovigilance professionals focus on the most critical issues.
By improving the accuracy and efficiency of signal detection, machine learning enables faster identification of potential safety risks, leading to more timely interventions.
4. Automating Data Processing and Reporting
Pharmacovigilance involves the collection, processing, and reporting of vast amounts of data from various sources, including clinical trials, EHRs, spontaneous reporting systems, and social media. Traditionally, these tasks have been manual and labor-intensive, leading to delays and the potential for human error.
Machine learning can automate many of these processes, reducing the workload for pharmacovigilance professionals and increasing the speed and accuracy of data processing. For instance, ML algorithms can automatically categorize and code ADR reports, extract relevant information from unstructured data, and generate standardized safety reports for regulatory authorities.
Automation not only streamlines pharmacovigilance workflows but also ensures that data is processed consistently and accurately, reducing the risk of errors and improving the overall quality of drug safety monitoring.
5. Real-Time Monitoring and Post-Marketing Surveillance
Post-marketing surveillance is a crucial aspect of drug safety monitoring that continues after a drug has been approved and released to the market. This phase is essential for identifying long-term ADRs and monitoring the safety of drugs in the broader population.
Machine learning enables real-time monitoring of drug safety by continuously analyzing data from various sources, such as EHRs, wearable devices, and social media. This real-time analysis allows for the early detection of safety signals and the rapid identification of emerging risks.
For example, machine learning algorithms can analyze data from wearable devices to monitor patients' vital signs and detect any abnormal changes that may indicate an ADR. This real-time monitoring enables healthcare providers to intervene quickly, preventing serious adverse events and improving patient outcomes.
Benefits of Machine Learning in Drug Safety Monitoring:
The integration of machine learning into drug safety monitoring offers several significant benefits:
1. Improved Accuracy and Efficiency
Machine learning algorithms can process and analyze vast amounts of data much faster and more accurately than humans. This improved efficiency allows for quicker identification of ADRs and safety signals, leading to faster interventions and better patient outcomes.
2. Early Detection of ADRs
By continuously analyzing data from various sources, machine learning enables the early detection of ADRs, even those that are rare or unexpected. This early detection is crucial for preventing harm to patients and ensuring the safe use of medications.
3. Personalized Medicine
Machine learning allows for the development of predictive models that can identify which patients are at higher risk of experiencing ADRs. This capability supports personalized medicine by enabling healthcare providers to tailor treatment plans to individual patients, minimizing the risk of ADRs and improving patient outcomes.
4. Enhanced Decision-Making
Machine learning provides pharmacovigilance professionals with data-driven insights that support more informed decision-making. By identifying patterns and correlations in large datasets, machine learning helps professionals make better decisions about drug safety and regulatory actions.
5. Scalability
Machine learning algorithms can easily scale to handle large and complex datasets, making them well-suited for analyzing data from global pharmacovigilance systems. This scalability is particularly valuable as the volume of data generated by healthcare systems continues to grow.
Challenges of Machine Learning in Drug Safety Monitoring:
While machine learning offers many benefits for drug safety monitoring, it also presents several challenges:
1. Data Quality and Availability
The effectiveness of machine learning algorithms depends on the quality and availability of data. Incomplete, inaccurate, or biased data can lead to incorrect predictions and conclusions. Ensuring that high-quality data is available for analysis is critical for the success of machine learning in pharmacovigilance.
2. Interpretability and Transparency
Machine learning models, particularly complex ones like deep learning, can be challenging to interpret. This lack of interpretability can make it difficult for pharmacovigilance professionals and regulatory authorities to understand how a model arrived at a particular decision. Ensuring transparency and interpretability is essential for building trust in machine learning algorithms.
3. Regulatory and Ethical Considerations
The use of machine learning in drug safety monitoring raises several regulatory and ethical considerations. For example, there are concerns about data privacy, the potential for algorithmic bias, and the need for regulatory oversight of machine learning models. Addressing these issues is crucial for the responsible use of machine learning in pharmacovigilance.
4. Integration with Existing Systems
Integrating machine learning into existing pharmacovigilance systems can be challenging, particularly when these systems rely on legacy technologies. Ensuring interoperability and seamless integration is essential for the successful adoption of machine learning in drug safety monitoring.
The Future of Machine Learning in Drug Safety Monitoring:
The future of machine learning in drug safety monitoring is promising, with several trends and developments expected to shape the field:
1. Advanced AI and Deep Learning Models
As AI and deep learning models continue to advance, they will likely play a more significant role in drug safety monitoring. These models can analyze more complex data and provide deeper insights into drug safety, enabling more accurate predictions and early detection of ADRs.
2. Integration with Real-World Data
The integration of machine learning with real-world data, such as data from EHRs, wearable devices, and social media, will enhance drug safety monitoring. This integration will enable more comprehensive and real-time analysis of drug safety, leading to better patient outcomes.
3. Personalized Pharmacovigilance
Machine learning will continue to support the development of personalized pharmacovigilance, where drug safety monitoring is tailored to individual patients. This approach will enable more precise identification of ADRs and better management of drug safety for specific patient populations.
4. Regulatory Frameworks for AI and Machine Learning
As the use of machine learning in pharmacovigilance grows, regulatory authorities will likely develop frameworks and guidelines.
Conclusion:
Machine learning is transforming drug safety monitoring by enhancing the accuracy, speed, and efficiency of detecting adverse drug reactions, predicting potential risks, and automating data analysis. By leveraging vast amounts of data from diverse sources, machine learning enables real-time monitoring and more personalized approaches to pharmacovigilance, ultimately improving patient safety and outcomes. However, the integration of this technology also presents challenges, including data quality, interpretability, regulatory considerations, and the need for robust integration with existing systems.
Comments