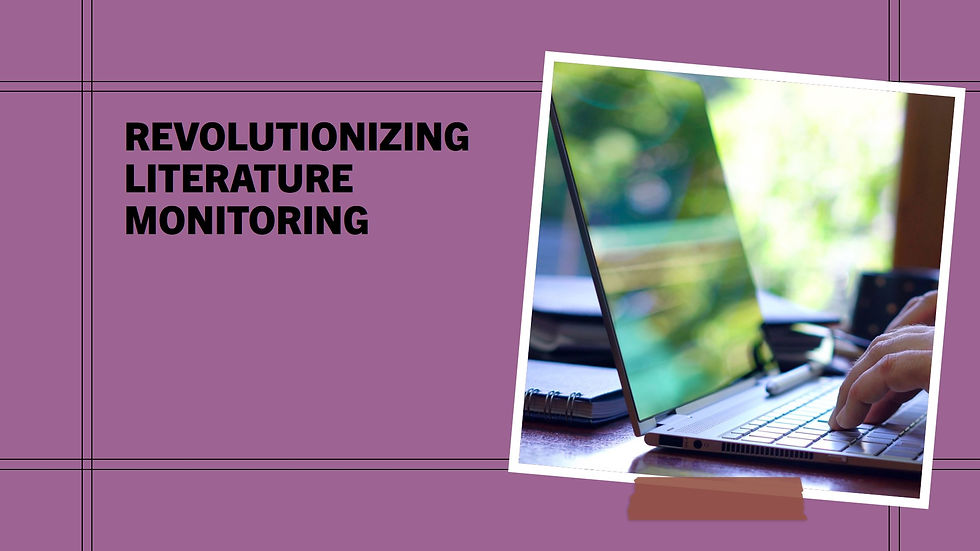
In an era of rapid advancements in healthcare, pharmaceuticals, and biotechnology, the ability to stay updated with the latest research is crucial. Literature monitoring, which involves the systematic tracking of scientific publications to identify relevant information, has become an essential practice in various fields, including pharmacovigilance, clinical research, and healthcare quality improvement. However, the sheer volume of scientific literature being published daily can overwhelm traditional methods of literature monitoring. This is where machine learning (ML) comes into play. By automating and enhancing literature monitoring processes, machine learning significantly improves efficiency, accuracy, and the ability to extract meaningful insights. In this blog, we will explore how machine learning transforms literature monitoring and the benefits it offers across different sectors.
1. Understanding Literature Monitoring
Literature monitoring is the process of systematically searching for, evaluating, and summarizing relevant scientific literature. It involves tracking publications in academic journals, conference proceedings, and other sources to gather information on specific topics, such as drug safety, disease management, and treatment outcomes. Key objectives of literature monitoring include:
Identifying Emerging Trends: Keeping abreast of new research findings helps professionals identify emerging trends in healthcare and science.
Supporting Evidence-Based Decision-Making: Access to the latest research enables healthcare professionals and decision-makers to make informed decisions based on current evidence.
Regulatory Compliance: In the pharmaceutical industry, literature monitoring is essential for compliance with regulatory requirements related to drug safety and efficacy.
Enhancing Knowledge Management: Organizations can build a knowledge repository by continuously monitoring literature, facilitating knowledge sharing and collaboration.
2. Challenges in Traditional Literature Monitoring
Traditional literature monitoring methods are often labor-intensive and time-consuming. Some of the key challenges include:
Volume of Publications: The number of scientific articles being published is growing exponentially, making it difficult for professionals to keep up.
Resource Limitations: Manual literature monitoring requires significant human resources, including researchers and analysts, which can strain budgets and time.
Information Overload: Sifting through large volumes of literature can lead to information overload, making it challenging to identify relevant articles.
Variability in Quality: The quality and relevance of published research can vary widely, requiring careful evaluation of each article.
Data Extraction Difficulties: Extracting meaningful insights from published studies often involves complex data extraction processes that are prone to errors.
3. How Machine Learning Enhances Literature Monitoring
Machine learning offers innovative solutions to address the challenges of traditional literature monitoring. Here are several ways in which ML improves efficiency and effectiveness:
A. Automated Literature Search
Natural Language Processing (NLP): ML algorithms use NLP techniques to perform automated searches across multiple databases, including PubMed, Scopus, and Google Scholar. By understanding the context and semantics of search queries, these algorithms can retrieve relevant articles more effectively than keyword-based searches.
Smart Filtering: ML models can filter results based on predefined criteria, such as publication date, journal impact factor, and study relevance. This allows researchers to focus on high-quality studies that align with their interests.
Continuous Monitoring: Machine learning can continuously scan for new publications and alerts researchers when relevant articles are published, ensuring they never miss important information.
B. Data Extraction and Analysis
Automated Data Extraction: Machine learning algorithms can automatically extract critical data points from research articles, including study outcomes, sample sizes, and adverse events. This reduces the time spent on manual data extraction and minimizes human errors.
Text Classification: ML models can categorize literature into predefined topics or themes, enabling researchers to quickly locate studies relevant to their specific areas of interest.
Sentiment Analysis: Machine learning can analyze the tone and sentiment of literature related to specific treatments or interventions, providing insights into public perception and potential concerns.
C. Enhanced Signal Detection
Pattern Recognition: Machine learning algorithms can identify patterns and correlations within large datasets, helping researchers detect safety signals or emerging trends that may not be apparent through traditional analysis.
Predictive Analytics: ML models can use historical data to develop predictive models that estimate future trends or outcomes based on current literature. This helps researchers anticipate potential risks or benefits associated with specific treatments.
D. Synthesis and Reporting
Automated Synthesis: Machine learning can assist in synthesizing findings from multiple studies, generating concise summaries that highlight key insights. This allows researchers to gain a comprehensive understanding of a topic without reading each individual study.
Dynamic Reporting: ML-powered tools can create dynamic reports that update in real time as new literature is published. This ensures that stakeholders have access to the most current information related to their areas of interest.
4. Benefits of Machine Learning in Literature Monitoring
The integration of machine learning into literature monitoring processes offers several significant benefits:
A. Increased Efficiency
Machine learning automates various aspects of literature monitoring, significantly reducing the time and effort required to stay updated on relevant research. Researchers can focus their attention on analysis and interpretation rather than manual data collection.
B. Improved Accuracy
ML algorithms can process large volumes of data with a high degree of accuracy. By minimizing human errors in data extraction and analysis, machine learning enhances the reliability of findings and conclusions drawn from literature monitoring.
C. Enhanced Insights
Machine learning can uncover hidden patterns and correlations within the data that may not be readily apparent to human reviewers. This leads to a deeper understanding of research trends and safety signals, ultimately improving decision-making.
D. Scalability
As the volume of scientific literature continues to grow, machine learning offers a scalable solution for literature monitoring. ML systems can handle increasing amounts of data without compromising efficiency, making them ideal for monitoring multiple topics or therapeutic areas simultaneously.
5. Real-World Applications of Machine Learning in Literature Monitoring
Several organizations and institutions have successfully implemented machine learning in their literature monitoring processes:
A. Pharmaceutical Companies
Many pharmaceutical companies are utilizing machine learning to enhance their literature monitoring capabilities. For instance, a global pharmaceutical company developed an ML-based system that automatically scans and analyzes publications related to drug safety. By automating data extraction and signal detection, the company improved its efficiency in identifying safety signals and regulatory compliance issues.
B. Research Institutions
Research institutions are also leveraging machine learning to monitor literature for systematic reviews and meta-analyses. By using NLP algorithms, researchers can quickly identify relevant studies and extract data, streamlining the process of synthesizing evidence for clinical guidelines.
C. Public Health Organizations
Public health organizations are increasingly using machine learning to monitor literature related to infectious diseases, vaccine safety, and health interventions. By analyzing trends in published studies, these organizations can inform public health policies and responses to emerging health threats.
6. Challenges and Considerations in Implementing Machine Learning
While machine learning offers significant advantages in literature monitoring, several challenges must be considered:
A. Data Quality and Availability
The effectiveness of machine learning algorithms relies on the quality and availability of data. Inconsistent or low-quality data can lead to inaccurate predictions or insights. Ensuring access to reliable and comprehensive datasets is crucial for successful implementation.
B. Algorithm Bias
Machine learning algorithms can be susceptible to bias based on the data they are trained on. If training data is unrepresentative or contains biases, it may lead to skewed results. It is essential to evaluate and mitigate bias in ML models to ensure fair and accurate outcomes.
C. Interpretation of Results
While machine learning can generate findings and insights, human interpretation is critical for contextualizing and validating these results. Professionals must possess the expertise to evaluate ML-generated findings and make informed decisions based on the available evidence.
D. Integration with Existing Workflows
Integrating machine learning into existing literature monitoring workflows may require changes in processes, tools, and personnel. Organizations must be prepared to invest time and resources in training staff and adapting workflows to maximize the benefits of ML.
7. Future Trends in Machine Learning and Literature Monitoring
As technology continues to evolve, several trends are likely to shape the future of machine learning in literature monitoring:
A. Advanced Natural Language Processing
Future developments in NLP will enhance the ability of machine learning algorithms to understand and analyze complex scientific texts. Improved NLP capabilities will allow for more nuanced searches, better data extraction, and more accurate sentiment analysis.
B. Integration of AI and Human Expertise
The future of literature monitoring will likely involve a collaborative approach that combines machine learning capabilities with human expertise. While ML can handle large-scale data processing, professionals can provide context, judgment, and critical thinking to interpret findings.
C. Real-Time Literature Monitoring
As machine learning technologies advance, real-time literature monitoring will become increasingly feasible. Organizations will be able to receive immediate alerts and insights based on new publications, enabling proactive decision-making.
D. Greater Focus on Patient-Centric Approaches
As healthcare shifts toward a more patient-centric model, machine learning will play a pivotal role in understanding patient experiences and outcomes. ML algorithms can analyze patient-reported outcomes and adverse events to provide valuable insights into treatment safety and effectiveness.
Conclusion:
Machine learning is transforming literature monitoring by automating processes, enhancing data extraction and analysis, and uncovering meaningful insights. By addressing the challenges of traditional literature monitoring methods, machine learning improves efficiency, accuracy, and the ability to stay updated on relevant research.
As organizations across the healthcare and pharmaceutical sectors embrace machine learning, they can enhance their literature monitoring capabilities, support evidence-based decision-making, and ultimately improve patient care. By leveraging the power of machine learning, professionals can navigate the ever-expanding landscape of scientific literature, ensuring that they remain informed and equipped to respond to emerging trends and safety signals in their respective fields.
Comments