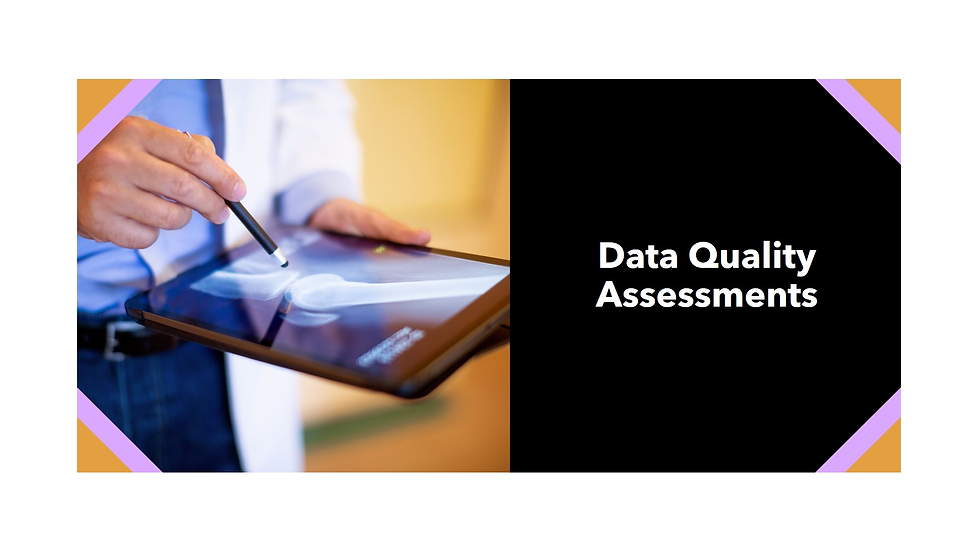
In the pharmaceutical industry, drug safety is paramount. Ensuring the efficacy and safety of medications requires rigorous monitoring and evaluation, a process that heavily relies on the quality of data within drug safety databases. These databases capture and store vast amounts of information related to adverse drug reactions (ADRs), patient safety reports, clinical trial data, and post-marketing surveillance. To maintain the integrity and reliability of this critical data, conducting regular data quality assessments is essential. This blog will explore the key steps and best practices for conducting data quality assessments in drug safety databases, highlighting the importance of accuracy, completeness, consistency, and timeliness in ensuring patient safety.
1. Understanding the Importance of Data Quality in Drug Safety
Data quality in drug safety databases directly impacts patient safety, regulatory compliance, and the credibility of pharmaceutical companies. Poor data quality can lead to incorrect conclusions, delayed safety signal detection, and potential harm to patients. Therefore, pharmaceutical companies and regulatory bodies such as the FDA (Food and Drug Administration) and EMA (European Medicines Agency) emphasize the need for robust data quality management practices.
High-quality data ensures that safety signals are detected promptly, adverse drug reactions are accurately reported, and regulatory submissions are reliable. It also helps in building trust with stakeholders, including patients, healthcare providers, and regulatory authorities. With the increasing volume and complexity of data generated from various sources, such as clinical trials, electronic health records (EHRs), and spontaneous reporting systems, the need for systematic data quality assessments has never been greater.
2. Key Dimensions of Data Quality in Drug Safety Databases
Before diving into the assessment process, it's essential to understand the key dimensions of data quality that should be evaluated. These dimensions provide a framework for identifying and addressing potential issues in drug safety databases:
Accuracy: Data should accurately reflect the real-world events they represent. Inaccurate data can lead to false conclusions about a drug's safety profile.
Completeness: All necessary data should be captured and recorded. Missing data can skew analyses and lead to underreporting of safety concerns.
Consistency: Data should be consistent across different sources and over time. Inconsistencies can create confusion and hinder the ability to draw reliable conclusions.
Timeliness: Data should be up-to-date and available when needed. Delays in data entry or processing can impact the timely detection of safety signals.
Relevance: Data should be relevant to the specific drug safety questions being addressed. Irrelevant data can clutter analyses and obscure important findings.
Accessibility: Data should be easily accessible to authorized users. Poor accessibility can delay critical safety assessments and decision-making.
3. Steps to Conduct Data Quality Assessments in Drug Safety Databases
Conducting a data quality assessment involves several steps, each aimed at evaluating different aspects of data quality and identifying areas for improvement.
Step 1: Define the Scope and Objectives
The first step in conducting a data quality assessment is to define the scope and objectives of the assessment. This involves identifying the specific drug safety databases to be assessed, the types of data to be evaluated (e.g., ADR reports, clinical trial data), and the key data quality dimensions to focus on.
Example Objective: Assess the accuracy and completeness of adverse drug reaction (ADR) reports in a post-marketing surveillance database.
The scope should also include the timeframe for the assessment, the data sources to be reviewed, and any specific regulatory requirements that need to be considered.
Step 2: Identify Data Quality Metrics
Once the scope and objectives are defined, the next step is to identify the specific data quality metrics that will be used to evaluate the data. These metrics should align with the data quality dimensions identified earlier and be tailored to the specific context of drug safety.
Accuracy Metrics: Percentage of ADR reports with correct patient demographics, correct drug names, and correct event dates.
Completeness Metrics: Percentage of ADR reports with all mandatory fields completed, percentage of missing data for key variables.
Consistency Metrics: Rate of inconsistencies between ADR reports and EHR data, rate of discrepancies in duplicate reports.
Timeliness Metrics: Average time from ADR occurrence to report entry, percentage of reports entered within regulatory timeframes.
Relevance Metrics: Percentage of reports relevant to the specific drug safety question under investigation.
Accessibility Metrics: Percentage of authorized users with access to required data, average time to retrieve data for analysis.
Step 3: Data Collection and Extraction
With the metrics defined, the next step is to collect and extract the necessary data from the drug safety databases. This may involve querying the databases to retrieve relevant records, extracting data from EHR systems, or aggregating data from multiple sources.
Data Extraction Tools: Use data extraction tools and software to automate the process and ensure consistency in data retrieval. Examples include SQL queries, ETL (Extract, Transform, Load) tools, and data warehousing solutions.
Data Sampling: In some cases, it may be necessary to use sampling techniques to assess data quality, especially when dealing with large datasets. Random sampling or stratified sampling can provide a representative view of data quality without needing to review every record.
Step 4: Data Quality Evaluation
Once the data is collected, the next step is to evaluate its quality based on the identified metrics. This involves analyzing the data to identify any issues related to accuracy, completeness, consistency, timeliness, relevance, and accessibility.
Accuracy Evaluation: Compare the data against known standards or reference datasets to identify errors or discrepancies. For example, cross-check ADR reports against EHR data to verify patient demographics and event dates.
Completeness Evaluation: Review the data to identify missing values or incomplete records. Calculate the percentage of missing data for each key variable and assess the impact on overall data quality.
Consistency Evaluation: Analyze the data for inconsistencies across different sources or over time. For example, check for discrepancies between ADR reports and duplicate entries or inconsistencies in coding practices.
Timeliness Evaluation: Assess the time taken to enter data into the database and identify any delays that could impact safety assessments. Calculate the average time from ADR occurrence to report entry and compare it against regulatory requirements.
Relevance Evaluation: Review the data to ensure it is relevant to the specific drug safety questions being addressed. Identify any irrelevant or extraneous data that may be cluttering the analysis.
Accessibility Evaluation: Evaluate the ease of access to the data for authorized users. Identify any barriers to data access, such as restrictive permissions, data silos, or technical issues.
Step 5: Identify and Prioritize Data Quality Issues
After evaluating the data, the next step is to identify and prioritize the data quality issues that need to be addressed. This involves categorizing the issues based on their impact on drug safety assessments and regulatory compliance.
High-Priority Issues: Issues that directly impact patient safety or regulatory compliance should be addressed first. For example, missing data in ADR reports or significant delays in data entry.
Medium-Priority Issues: Issues that may affect the reliability of safety assessments but are less critical than high-priority issues. For example, inconsistencies in coding practices or minor discrepancies in patient demographics.
Low-Priority Issues: Issues that have minimal impact on safety assessments and can be addressed as part of routine data quality management. For example, irrelevant data that does not affect the analysis.
Step 6: Implement Data Quality Improvements
Once the data quality issues are identified and prioritized, the next step is to implement improvements to address these issues. This may involve making changes to data collection processes, updating database management practices, or providing additional training to staff.
Process Improvements: Streamline data entry processes to reduce the likelihood of errors, implement automated data validation checks, and enhance data integration between different systems.
Technology Enhancements: Invest in advanced data quality management tools, such as data cleansing software, data governance platforms, and machine learning algorithms for anomaly detection.
Staff Training: Provide training to staff on best practices for data entry, coding, and reporting. Emphasize the importance of data quality in ensuring patient safety and regulatory compliance.
Data Governance: Establish or strengthen data governance frameworks to ensure ongoing data quality management. Define clear roles and responsibilities for data stewards, implement data quality standards, and regularly review data quality metrics.
Step 7: Monitor and Review Data Quality
Data quality assessment is not a one-time activity but an ongoing process. After implementing improvements, it's important to continuously monitor and review data quality to ensure that issues are being addressed and that the quality of data remains high.
Regular Audits: Conduct regular audits of drug safety databases to identify new data quality issues and assess the effectiveness of implemented improvements.
Continuous Monitoring: Use data quality monitoring tools to continuously track data quality metrics in real time. Set up alerts to notify relevant stakeholders of any significant changes in data quality.
Feedback Loops: Establish feedback loops with data entry staff, data stewards, and other stakeholders to continuously improve data quality practices. Encourage open communication and collaboration to identify and address data quality challenges.
Regulatory Compliance: Regularly review regulatory requirements and ensure that data quality practices align with these requirements. Update data quality assessments and improvements as needed to maintain compliance.
Conclusion:
Conducting data quality assessments in drug safety databases is a critical process that ensures the accuracy, completeness, and reliability of data used in drug safety monitoring and evaluation. By following a systematic approach that includes defining the scope and objectives, identifying data quality metrics, collecting and evaluating data, and implementing continuous improvements, pharmaceutical companies can maintain high standards of data quality. This, in turn, enhances patient safety, supports regulatory compliance, and builds trust with stakeholders.
In an era of increasing data complexity and volume, investing in robust data quality management practices is essential for the pharmaceutical industry. By prioritizing data quality, companies can better detect safety signals,
Comments