How to Conduct Effective Data Audits in Vaccine Safety Databases
- Chaitali Gaikwad
- Jul 9, 2024
- 3 min read
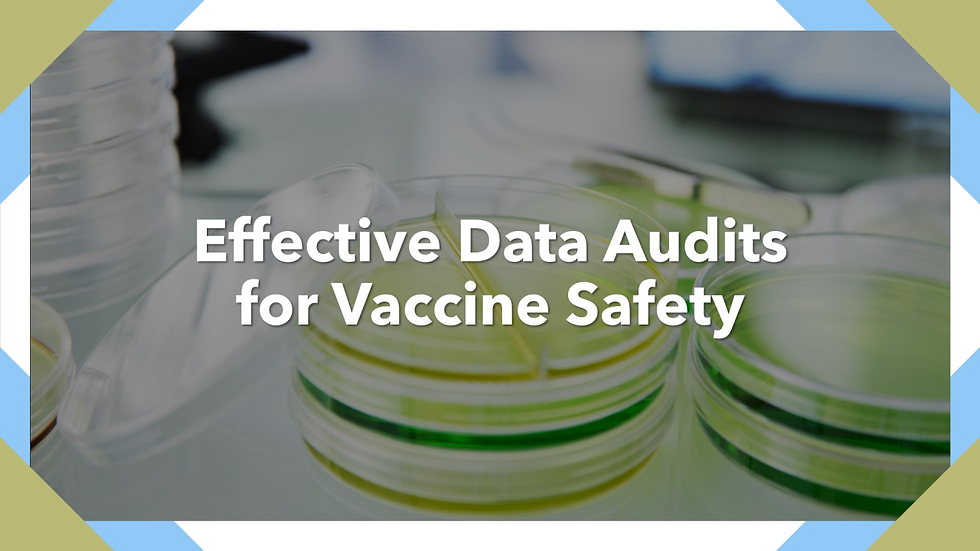
Vaccine safety databases are pivotal in monitoring and ensuring the safety of immunization programs worldwide. Conducting effective data audits is crucial to maintaining data integrity, reliability, and compliance with regulatory standards. This blog explores the importance of data audits in vaccine safety databases, key steps in conducting audits, challenges, best practices, and future directions.
Introduction to Vaccine Safety Databases:
Vaccine safety databases collect and analyze information on adverse events following immunization (AEFI) from various sources, including healthcare providers, vaccine manufacturers, and public health agencies. These databases play a critical role in:
Monitoring Safety: Detecting and investigating potential adverse reactions to vaccines.
Supporting Public Health Decision-making: Providing evidence for vaccine licensure, recommendations, and policy formulation.
Enhancing Public Confidence: Communicating transparently about vaccine safety to healthcare professionals and the public.
Importance of Data Audits in Vaccine Safety:
Data audits ensure the accuracy, completeness, and reliability of information in vaccine safety databases:
Quality Assurance: Verifying data integrity and adherence to data standards and protocols.
Regulatory Compliance: Meeting regulatory requirements for pharmacovigilance and safety reporting.
Continuous Improvement: Identifying areas for process enhancement and data quality enhancement.
Key Steps in Conducting Data Audits:
1. Establish Audit Objectives and Scope
Define the purpose, goals, and scope of the data audit:
Audit Criteria: Determine the criteria for evaluating data quality, completeness, and compliance with regulatory standards.
Scope Definition: Identify the timeframe, data sources, and specific variables to be audited.
2. Data Collection and Preparation
Gather relevant data from vaccine safety databases and prepare for auditing:
Data Extraction: Extract datasets based on audit criteria, ensuring inclusion of necessary variables and data fields.
Data Cleansing: Cleanse data to remove duplicates, errors, and inconsistencies that may affect audit outcomes.
3. Data Quality Assessment
Evaluate data quality and completeness using standardized metrics:
Accuracy: Verify the correctness of data entries against original sources and documentation.
Completeness: Assess the presence of required data fields and information for each reported adverse event.
Consistency: Ensure data consistency across different reporting periods and sources.
4. Compliance Verification
Ensure compliance with regulatory requirements and organizational protocols:
Regulatory Standards: Verify adherence to reporting guidelines and standards (e.g., ICH E2B, MedDRA coding).
Internal Protocols: Assess compliance with internal procedures for data collection, entry, and reporting.
Challenges in Conducting Data Audits:
1. Data Complexity and Variability
Heterogeneous Data Sources: Integrating data from diverse reporting systems and healthcare settings.
Data Standardization: Addressing differences in data formats, terminology, and coding practices.
2. Resource Constraints
Time and Personnel: Allocating sufficient resources for conducting thorough audits and data validation processes.
Technological Infrastructure: Ensuring access to robust IT systems and tools for data extraction and analysis.
3. Regulatory and Ethical Considerations
Data Privacy: Safeguarding patient confidentiality and complying with data protection regulations (e.g., GDPR, HIPAA).
Ethical Guidelines: Adhering to ethical standards in handling sensitive healthcare information and adverse event reporting.
Best Practices for Effective Data Audits:
1. Continuous Monitoring and Surveillance
Implement ongoing monitoring and surveillance mechanisms:
Real-time Alerts: Setting up alerts for data anomalies and potential safety signals.
Automated Checks: Using automated tools to conduct routine data quality checks and audits.
2. Stakeholder Engagement and Collaboration
Involve stakeholders in the auditing process:
Multidisciplinary Teams: Collaborate with healthcare providers, epidemiologists, data scientists, and regulatory experts.
Training and Education: Provide training on data auditing protocols and standards for consistent reporting practices.
3. Documentation and Reporting
Document audit findings and recommendations for continuous improvement:
Audit Reports: Prepare detailed reports outlining audit methodologies, findings, and corrective actions.
Feedback Loop: Share audit results with stakeholders to implement corrective measures and enhance data quality.
Future Directions and Innovations:
1. Advanced Analytical Techniques
AI and Machine Learning: Deploy AI-driven algorithms for predictive analytics and anomaly detection in vaccine safety data.
Natural Language Processing (NLP): Use NLP to analyze unstructured data sources (e.g., narrative reports) for enhanced data insights.
2. Global Collaboration and Data Sharing
Interoperability: Enhance data interoperability and standardization across global vaccine safety databases.
International Networks: Establish collaborative networks for sharing vaccine safety data and insights globally.
3. Patient-Centered Auditing
Patient Reporting Systems: Integrate patient-reported outcomes and adverse events to enrich vaccine safety data.
Public Engagement: Engage patients and the public in reporting vaccine-related adverse events to enhance surveillance and safety monitoring.
Conclusion:
Effective data audits are essential for ensuring the accuracy, reliability, and compliance of vaccine safety databases. By implementing systematic auditing processes, healthcare organizations can enhance data quality, meet regulatory requirements, and support evidence-based decision-making in vaccine safety monitoring. Overcoming challenges through advanced technologies, stakeholder collaboration, and continuous improvement efforts will drive advancements in vaccine safety auditing practices and contribute to public health outcomes globally.
Comments