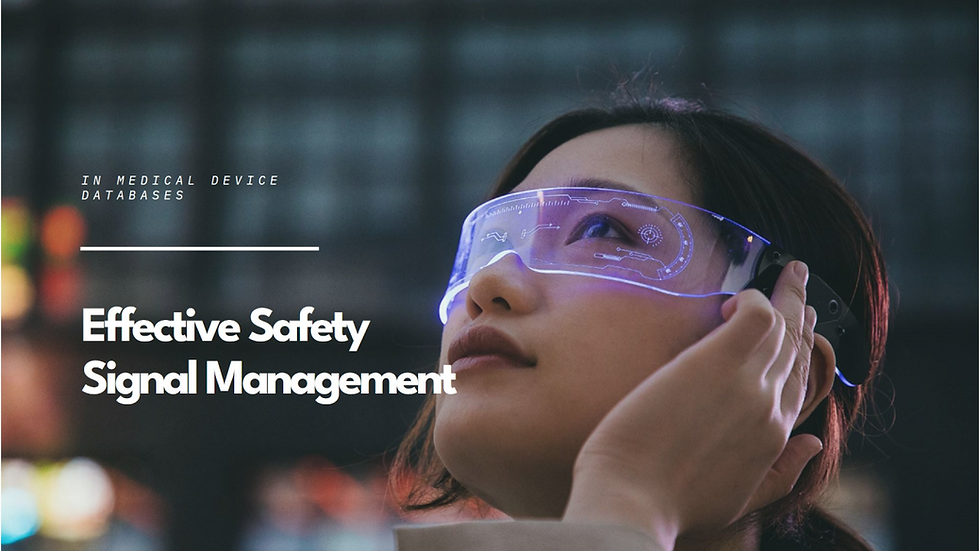
In the realm of medical devices, ensuring safety and efficacy is paramount to protecting patient health. Safety signal management plays a crucial role in identifying potential risks associated with medical devices, enabling timely intervention and regulatory action when necessary. This blog explores the principles, processes, and strategies involved in conducting effective safety signal management within medical device databases, emphasizing the importance of proactive surveillance and rapid response to emerging safety concerns.
Understanding Safety Signal Management:
What are Safety Signals?
Safety signals in the context of medical devices refer to indications or patterns in data that suggest a potential risk or safety issue associated with a device. These signals may arise from adverse event reports, post-market surveillance data, clinical studies, literature reviews, or regulatory notifications.
Objectives of Safety Signal Management:
Early Detection: Identify potential safety concerns or emerging risks associated with medical devices as early as possible.
Evaluation: Assess the clinical significance, causality, and potential impact of identified safety signals on patient safety and public health.
Action: Initiate appropriate actions, such as further investigation, risk mitigation strategies, labeling changes, or device recalls, to address identified safety signals and prevent harm to patients.
Key Components of Effective Safety Signal Management:
1. Data Collection and Integration
Adverse Event Reporting Systems (AERS): Establish robust systems for collecting adverse event reports from healthcare providers, patients, and other stakeholders. Integrate data from multiple sources, including clinical trials, post-market surveillance studies, complaint handling databases, and regulatory notifications.
Electronic Health Records (EHRs): Utilize electronic health records to capture real-world data on device usage, patient outcomes, and adverse events in clinical practice settings.
2. Signal Detection and Prioritization
Statistical Methods: Apply statistical algorithms and data mining techniques to detect signals from large datasets. Common methods include disproportionality analysis, Bayesian data mining, and time-to-event analyses.
Signal Prioritization: Prioritize signals based on factors such as severity of adverse events, frequency of reports, patient demographics, and clinical relevance. Use risk assessment tools to rank signals for further investigation.
3. Signal Evaluation and Assessment
Clinical Review: Conduct thorough clinical evaluations of identified signals to assess causality, potential mechanisms, and clinical significance. Engage clinical experts, epidemiologists, and biostatisticians in the evaluation process.
Literature Review: Perform comprehensive reviews of medical literature and relevant scientific publications to gather evidence and contextual information related to the identified safety signals.
4. Risk Communication and Decision-Making
Regulatory Reporting: Comply with regulatory requirements for reporting safety signals to health authorities, such as the FDA's Medical Device Reporting (MDR) regulations or the EU's Medical Device Vigilance System.
Stakeholder Communication: Communicate safety findings and recommendations effectively to healthcare providers, patients, regulatory agencies, and device manufacturers. Transparency and timely communication are essential for maintaining trust and facilitating informed decision-making.
5. Risk Management and Mitigation Strategies
Risk Mitigation Plans: Develop and implement risk mitigation strategies, including labeling updates, post-market surveillance studies, user training programs, and device modifications or recalls, as appropriate.
Post-Market Surveillance: Establish proactive surveillance programs to monitor the ongoing safety and performance of medical devices in real-world clinical settings. Use feedback mechanisms to capture and respond to emerging safety concerns.
Case Studies: Effective Safety Signal Management Practices:
Case Study 1: Implantable Cardioverter Defibrillators (ICDs)
Following reports of battery depletion issues in certain models of ICDs, a medical device manufacturer initiated a comprehensive safety signal management process. By analyzing adverse event reports, conducting root cause investigations, and collaborating with healthcare providers, the manufacturer identified and addressed the underlying design flaw. Regulatory notifications, device recalls, and enhanced battery monitoring protocols were implemented to mitigate risks and ensure patient safety.
Case Study 2: Orthopedic Implants
A safety signal indicating a higher-than-expected rate of implant fractures in a specific line of orthopedic implants prompted a joint investigation by regulatory agencies and device manufacturers. Through collaborative data analysis, including patient registries and clinical studies, factors contributing to the fractures were identified, such as material fatigue and surgical technique variations. Revised implant designs, surgeon training programs, and enhanced post-market surveillance measures were implemented to reduce fracture risks and improve patient outcomes.
Challenges and Considerations:
Data Quality and Integration: Ensuring the accuracy, completeness, and timeliness of data from diverse sources is critical for reliable signal detection and assessment.
Causality Assessment: Determining whether observed adverse events are directly linked to device use requires careful consideration of confounding factors and alternative explanations.
Regulatory Compliance: Adhering to regulatory requirements for safety reporting and risk management activities across different jurisdictions can be complex and resource-intensive.
Interdisciplinary Collaboration: Effective safety signal management relies on collaboration among healthcare providers, regulators, manufacturers, researchers, and patients to achieve comprehensive risk assessment and mitigation.
Future Directions and Innovations:
Advanced Analytics: Harnessing AI and machine learning algorithms for real-time signal detection, predictive analytics, and automated risk assessment in medical device safety surveillance.
Real-World Evidence (RWE): Expanding the use of real-world data sources, such as EHRs and patient registries, to enhance signal detection capabilities and support evidence-based decision-making.
Patient-Centered Safety: Incorporating patient-reported outcomes and feedback into safety signal management processes to capture patient perspectives and experiences more effectively.
Conclusion:
Effective safety signal management in medical device databases is essential for identifying and responding to potential risks promptly, ensuring patient safety, and maintaining public confidence in healthcare technologies. By implementing robust data collection, advanced analytics, rigorous evaluation processes, proactive risk communication, and collaborative risk mitigation strategies, stakeholders can enhance the safety and performance of medical devices throughout their lifecycle.
As technology evolves and regulatory frameworks adapt, ongoing efforts to strengthen safety signal management practices will drive continuous improvement in medical device safety surveillance and contribute to safer healthcare delivery globally.
Kommentare