How to Conduct Efficient Data Management in Drug Safety Databases
- Chaitali Gaikwad
- Aug 22, 2024
- 6 min read
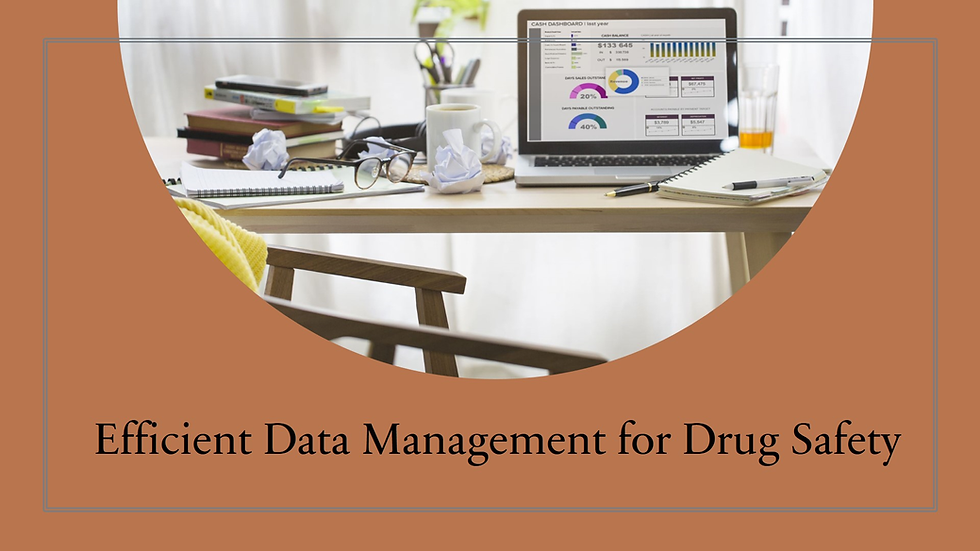
Data management in drug safety databases is a critical aspect of pharmacovigilance, ensuring that information related to adverse drug reactions (ADRs) is accurately captured, stored, and analyzed. Efficient data management not only helps pharmaceutical companies meet regulatory requirements but also plays a vital role in safeguarding public health. In this blog, we will explore the strategies and best practices for conducting efficient data management in drug safety databases.
Understanding the Importance of Data Management in Drug Safety:
Before delving into the specifics of efficient data management, it is essential to understand why it is so crucial in the context of drug safety. Drug safety databases, often referred to as pharmacovigilance databases, are repositories that store information about ADRs, safety signals, and other relevant data related to pharmaceutical products. These databases are used by pharmaceutical companies, regulatory authorities, and healthcare professionals to monitor the safety profile of drugs in real-time.
Efficient data management in these databases ensures that:
Regulatory Compliance: Regulatory agencies like the FDA, EMA, and WHO have stringent requirements for data collection, reporting, and analysis in drug safety. Non-compliance can lead to severe penalties and delays in drug approvals.
Timely Detection of Safety Signals: Well-organized and accessible data enables the early detection of potential safety signals, allowing for timely intervention and risk mitigation.
Accurate Risk-Benefit Analysis: Data integrity is paramount when assessing the risks and benefits of a drug. Any inaccuracies can lead to flawed conclusions, potentially endangering patient safety.
Effective Communication: Clear and concise data management facilitates communication between different stakeholders, including regulatory bodies, healthcare providers, and patients.
Key Challenges in Data Management for Drug Safety Managing data in drug safety databases comes with its own set of challenges. These challenges need to be addressed to ensure efficient and effective data management:
1. Data Volume and Complexity
The sheer volume of data generated in pharmacovigilance is enormous. ADR reports, clinical trial data, literature references, and real-world evidence (RWE) all contribute to a complex dataset. Managing this volume while maintaining accuracy and accessibility is a significant challenge.
2. Data Quality and Consistency
Ensuring data quality is a constant struggle. Inconsistent data entry, duplicate records, and incomplete information can all compromise the integrity of the database. Data quality issues can lead to incorrect analysis and delayed safety signals.
3. Regulatory Compliance
Different regions have varying regulatory requirements for data submission and reporting. Staying compliant with these requirements while managing a global pharmacovigilance database is a complex task that requires constant monitoring and updating.
4. Data Security and Privacy
With the increasing amount of sensitive patient information being stored in drug safety databases, data security and privacy have become paramount. Ensuring that data is protected against breaches while still being accessible to authorized users is a delicate balance.
5. Integration of Diverse Data Sources
Pharmacovigilance data comes from a variety of sources, including clinical trials, spontaneous reports, and electronic health records (EHRs). Integrating these diverse data sources into a single, coherent database is a significant challenge.
Strategies for Efficient Data Management in Drug Safety Databases
To overcome these challenges, pharmaceutical companies and regulatory authorities must adopt a strategic approach to data management. The following strategies can help ensure efficient and effective data management in drug safety databases:
1. Implementing a Robust Data Governance Framework
A strong data governance framework is the foundation of efficient data management. This framework should include clear policies and procedures for data entry, validation, and maintenance. It should also define roles and responsibilities for data management tasks to ensure accountability.
Key components of a data governance framework include:
Data Standards: Establishing standardized data formats and terminologies helps ensure consistency across the database. For example, using MedDRA (Medical Dictionary for Regulatory Activities) for coding ADRs ensures that data is categorized consistently.
Data Stewardship: Appointing data stewards who are responsible for overseeing data quality and consistency can help maintain the integrity of the database.
Data Quality Metrics: Implementing metrics to measure data quality, such as completeness, accuracy, and timeliness, allows for ongoing monitoring and improvement.
2. Leveraging Automation and AI
Automation and artificial intelligence (AI) can significantly enhance the efficiency of data management in drug safety databases. Automation can streamline repetitive tasks such as data entry, validation, and reporting, reducing the risk of human error and speeding up processes.
AI, particularly machine learning, can be used to:
Signal Detection: AI algorithms can analyze large datasets to identify potential safety signals that may not be immediately apparent to human analysts.
Data Cleaning: Machine learning models can identify and correct inconsistencies in the data, improving overall data quality.
Natural Language Processing (NLP): NLP can be used to extract relevant information from unstructured data sources such as medical literature and social media, integrating it into the drug safety database.
3. Ensuring Data Security and Privacy
Given the sensitive nature of the data stored in drug safety databases, robust security measures are essential. These measures should include:
Encryption: All data should be encrypted both at rest and in transit to protect against unauthorized access.
Access Control: Implementing strict access controls ensures that only authorized users can access the database. Role-based access control (RBAC) can be used to restrict access based on the user’s role within the organization.
Audit Trails: Maintaining detailed audit trails allows for tracking all data access and modifications, providing accountability and transparency.
Compliance with Data Privacy Regulations: Ensuring compliance with data privacy regulations such as GDPR and HIPAA is essential for protecting patient information and avoiding legal repercussions.
4. Integrating Data from Diverse Sources
To create a comprehensive drug safety database, data from various sources must be integrated seamlessly. This can be achieved through:
Data Interoperability Standards: Implementing interoperability standards such as HL7 (Health Level 7) ensures that data from different sources can be integrated into a single database without losing context or meaning.
Data Mapping: Mapping data from different sources to a common schema allows for consistent data entry and retrieval.
Data Harmonization: Harmonizing data from different sources involves reconciling discrepancies and ensuring that all data is consistent and comparable.
5. Continuous Monitoring and Improvement
Data management in drug safety databases is not a one-time task but an ongoing process. Continuous monitoring and improvement are essential for maintaining data quality and ensuring the efficiency of the database. This can be achieved through:
Regular Audits: Conducting regular audits of the database helps identify and address any issues related to data quality or regulatory compliance.
User Training: Providing ongoing training to users of the database ensures that they are aware of best practices for data entry and management.
Feedback Mechanisms: Implementing feedback mechanisms allows users to report any issues they encounter, enabling continuous improvement of the database.
6. Optimizing Database Architecture
The architecture of the drug safety database plays a crucial role in its efficiency. Optimizing the database architecture involves:
Scalability: Ensuring that the database can handle the growing volume of data as more ADR reports are collected and analyzed.
Performance: Optimizing the performance of the database to ensure that data retrieval and analysis are conducted quickly and efficiently.
Redundancy: Implementing redundancy measures such as data backups and failover systems ensures that data is not lost in the event of a system failure.
7. Collaborating with Regulatory Authorities
Close collaboration with regulatory authorities is essential for ensuring that the drug safety database meets all regulatory requirements. This collaboration can involve:
Regular Updates: Keeping the database up to date with the latest regulatory guidelines and requirements.
Data Sharing: Establishing mechanisms for securely sharing data with regulatory authorities to facilitate timely reporting and analysis.
Regulatory Audits: Preparing for and participating in regulatory audits to demonstrate compliance with data management standards.
Case Study: Efficient Data Management in Action:
To illustrate the impact of efficient data management in drug safety databases, let’s consider a hypothetical case study.
A pharmaceutical company, Pharma X, was struggling with data management in its drug safety database. The database was plagued with issues such as inconsistent data entry, duplicate records, and delays in signal detection. These issues not only posed a risk to patient safety but also put the company at risk of non-compliance with regulatory requirements.
Pharma X decided to overhaul its data management processes by implementing the strategies outlined above. They established a robust data governance framework, standardized data entry procedures, and appointed data stewards to oversee data quality. They also invested in automation and AI technologies to streamline data entry and signal detection.
Additionally, Pharma X implemented strict data security measures, including encryption and access controls, to protect patient information. They also worked closely with regulatory authorities to ensure compliance with all relevant guidelines.
As a result of these efforts, Pharma X saw significant improvements in the efficiency of its drug safety database. Data quality issues were reduced, signal detection times were shortened, and the company was able to maintain compliance with regulatory requirements. Most importantly, these improvements contributed to better patient safety outcomes.
Conclusion:
Efficient data management in drug safety databases is essential for ensuring regulatory compliance, detecting safety signals, and protecting patient health. By implementing a robust data governance framework, leveraging automation and AI, ensuring data security and privacy, integrating diverse data sources, and continuously monitoring and improving data management processes, pharmaceutical companies can achieve efficient and effective data management in their drug safety databases. This not only helps them meet regulatory requirements but also plays a critical role in safeguarding public health and ensuring the safety of pharmaceutical products.
Comments