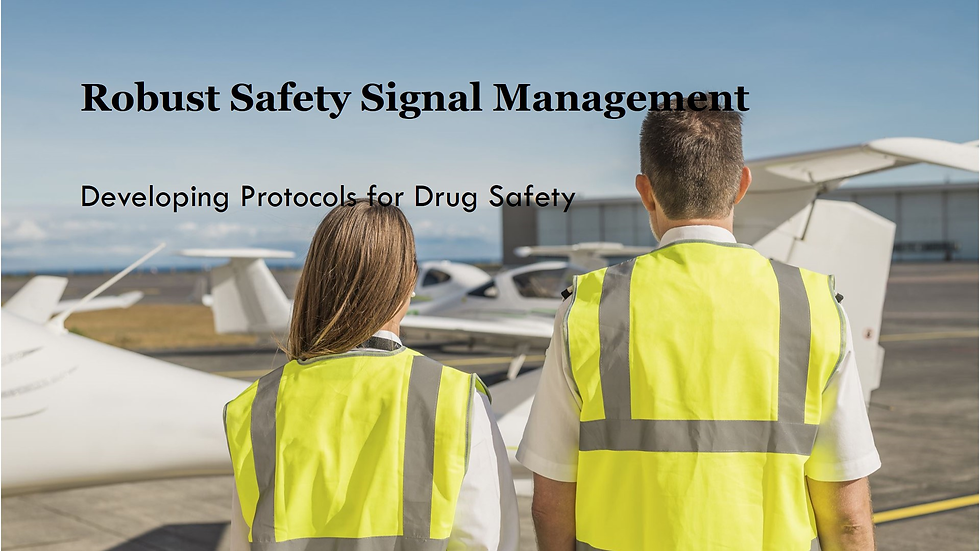
Safety signal management is a cornerstone of pharmacovigilance, responsible for identifying, assessing, and mitigating potential risks associated with pharmaceutical products. As drug safety systems grow more sophisticated and complex, the need for robust signal management protocols has never been greater. Ensuring that safety signals are detected early, evaluated accurately, and acted upon promptly is essential to protecting public health, regulatory compliance, and the reputation of pharmaceutical companies.
In this blog, we will explore the key elements and steps involved in developing robust safety signal management protocols for drug safety, emphasizing best practices for each stage, as well as the integration of modern technologies such as artificial intelligence (AI) and machine learning (ML).
1. Understanding Safety Signals
A safety signal refers to information suggesting a new or known adverse event that is potentially related to a drug and warrants further investigation. Signals can originate from a variety of sources, including spontaneous reports, clinical trials, post-marketing studies, electronic health records (EHR), and scientific literature.
Robust safety signal management protocols are essential to efficiently processing and interpreting these signals. Proper signal management ensures that potential risks are identified early and that they undergo rigorous evaluation before any regulatory or public health actions are taken.
2. Key Components of Safety Signal Management
Developing a robust safety signal management protocol requires clearly defining the core components involved in signal detection and management. Below are the essential steps:
a. Signal Detection
Signal detection is the first step in signal management, involving the identification of potential safety issues from multiple data sources. To ensure thorough and effective detection, companies should implement:
Routine monitoring of spontaneous adverse event (AE) reports: Spontaneous reporting systems like the FDA's Adverse Event Reporting System (FAERS) and the European Medicines Agency's EudraVigilance database are invaluable sources of safety information.
Data mining techniques: Automated systems, powered by statistical and AI tools, can sift through large datasets to detect patterns, associations, and emerging trends.
Literature monitoring: Continuous review of scientific publications and clinical trial data ensures that new information about a drug’s safety profile is incorporated into signal detection efforts.
Engagement with healthcare professionals and patients: Encouraging open communication channels with healthcare providers and consumers ensures that potential adverse events are reported early.
b. Signal Validation
Once a signal is detected, it must be validated to confirm whether it represents a new or previously unrecognized safety issue. The validation process typically involves:
Reviewing the clinical plausibility: Assessing whether the relationship between the drug and the adverse event makes biological and clinical sense.
Assessing the quality of the data: Evaluating the credibility of the data source, the completeness of the adverse event reports, and whether similar patterns have been reported by different sources.
Prioritization: Signals should be prioritized based on their potential impact on public health and patient safety, as well as regulatory obligations.
A robust protocol will establish clear criteria for validation, ensuring that only legitimate and meaningful signals are further investigated.
c. Signal Assessment
Signal assessment involves a comprehensive evaluation of the detected signal to determine its clinical relevance and the need for regulatory action. During this stage:
Quantitative analysis: Using advanced statistical tools, safety experts assess the frequency of adverse events compared to background rates, determining if the observed events exceed what would be expected.
Qualitative analysis: Experts review the clinical context of the signal, analyzing factors such as the drug’s mechanism of action, patient demographics, and potential risk factors that may contribute to adverse events.
Causality assessment: Evaluating whether there is a causal relationship between the drug and the adverse event based on the available evidence.
By integrating both quantitative and qualitative methods, companies can ensure that signal assessments are thorough and objective.
d. Signal Prioritization
Not all safety signals carry the same level of risk, and a key component of robust signal management protocols is the ability to prioritize signals effectively. Prioritization typically takes into account:
Severity of the adverse event: Signals involving serious adverse events, such as death or hospitalization, require immediate attention.
Frequency of occurrence: If an adverse event is occurring more frequently than expected, this could signal a widespread problem that needs to be addressed.
Regulatory implications: Some signals may trigger mandatory reporting obligations to regulatory authorities, while others may require ongoing monitoring.
Efficient prioritization ensures that resources are allocated to investigating the most critical signals first, reducing the time to detect and mitigate safety risks.
e. Signal Investigation and Action
Once a signal has been assessed and prioritized, it must be thoroughly investigated to confirm whether regulatory or safety actions are warranted. Potential actions may include:
Updating product labeling: If a signal suggests a new risk, companies may be required to update their drug’s labeling to include warnings or precautions.
Risk communication: Public health advisories or Dear Healthcare Professional letters may be issued to inform stakeholders of new safety concerns.
Regulatory submissions: Companies may need to submit updated safety data to regulatory bodies such as the FDA or EMA, which may result in safety recommendations or restrictions.
Risk management plan (RMP) updates: If a signal is confirmed as a significant risk, it may require updating a drug’s RMP or initiating risk mitigation strategies.
3. Best Practices for Developing Safety Signal Management Protocols
While every company’s signal management processes will vary depending on their size, portfolio, and regulatory environment, there are several best practices that can guide the development of robust safety signal management protocols:
a. Implement Standard Operating Procedures (SOPs)
To ensure consistency and efficiency in signal management, companies must develop clear SOPs that outline the roles, responsibilities, and processes for signal detection, validation, assessment, and investigation. These SOPs should:
Be regularly updated to reflect new regulations and best practices.
Ensure that all team members are trained in signal management processes.
Include timelines for signal detection and action, ensuring timely responses to potential safety concerns.
b. Establish Cross-functional Collaboration
Signal management is inherently multidisciplinary, requiring input from pharmacovigilance, clinical research, regulatory, medical affairs, and data science teams. To facilitate effective collaboration:
Develop cross-functional teams responsible for signal assessment and investigation.
Foster open communication between departments to ensure that safety signals are evaluated from multiple perspectives.
Engage external stakeholders, such as key opinion leaders (KOLs) and healthcare professionals, when specialized expertise is needed.
c. Use Technology to Enhance Signal Detection
Leveraging modern technology is crucial to developing a robust signal management protocol. Key technologies include:
AI and machine learning: These technologies can automate signal detection processes, reducing the time and resources needed to identify and validate signals. AI algorithms can also improve the accuracy of signal detection by identifying complex patterns and trends in large datasets.
Data mining and analytics platforms: Advanced data mining techniques enable companies to identify emerging safety concerns across vast pharmacovigilance databases in real-time.
EHR integration: Real-time integration with EHR systems can provide an additional data source for signal detection, allowing companies to monitor safety outcomes in clinical practice.
d. Ensure Regulatory Compliance
Signal management protocols must be aligned with local and international regulatory requirements, including those set by the FDA, EMA, and other health authorities. To stay compliant:
Monitor regulatory guidelines and updates regarding signal detection and reporting.
Establish clear reporting timelines and protocols for notifying regulatory agencies of new safety signals.
Ensure that signal management systems are auditable and that all signal management activities are properly documented.
4. Continuous Improvement and Risk Minimization
Signal management should not be viewed as a one-time process but rather as an ongoing effort. To ensure continuous improvement:
Regularly review and update protocols: Signal management protocols should be reviewed at least annually, ensuring they remain aligned with evolving regulations, technological advancements, and internal learnings.
Implement feedback loops: Post-implementation reviews of signal management actions can help companies assess the effectiveness of their processes and identify areas for improvement.
Proactively manage risks: By continuously monitoring drug safety data and updating risk management plans, companies can mitigate potential safety issues before they escalate.
5. The Role of AI and ML in Signal Management
Artificial intelligence and machine learning are revolutionizing pharmacovigilance, making signal detection and management more efficient and accurate. AI-driven tools can sift through massive amounts of data to uncover patterns, assess causality, and prioritize risks more effectively than traditional methods. By integrating AI into signal management protocols, companies can enhance the speed and accuracy of their safety signal evaluations, leading to faster responses to emerging drug safety risks.
Conclusion:
Developing robust safety signal management protocols for drug safety is an essential component of a comprehensive pharmacovigilance strategy. By implementing clear processes for signal detection, validation, assessment, prioritization, and action, and by leveraging modern technologies like AI and machine learning, companies can ensure that potential safety risks are identified and addressed promptly. In doing so, they protect public health, maintain regulatory compliance, and safeguard the reputation of their products. As the pharmaceutical landscape continues to evolve, robust safety signal management will remain a critical priority for ensuring drug safety in an increasingly complex and data-driven world.
Comentarios