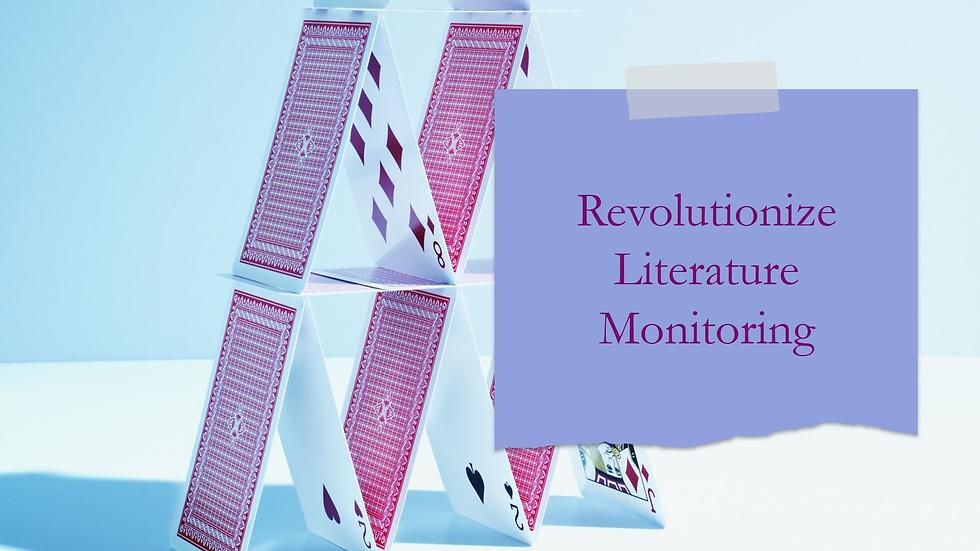
In today’s fast-paced world, the ability to efficiently monitor and analyze literature is critical for researchers, professionals, and organizations alike. With an ever-increasing volume of research publications, articles, and reports emerging every day, keeping track of the relevant literature can be overwhelming. However, advancements in artificial intelligence (AI) and machine learning (ML) provide innovative solutions for enhancing literature monitoring. This blog explores how AI and ML can be utilized to improve literature monitoring processes, making them more efficient and effective.
Understanding Literature Monitoring:
Literature monitoring involves systematically tracking and analyzing published works in a particular field or subject area. It is essential for researchers to stay updated on new findings, methodologies, and trends that can impact their work. Traditional literature monitoring methods often involve manual searches through databases, journals, and publications, which can be time-consuming and prone to oversight.
The Challenges of Traditional Literature Monitoring:
While traditional literature monitoring methods are invaluable, they come with several challenges:
Information Overload: With thousands of research papers published daily, it is challenging for researchers to keep up with relevant literature.
Time-Consuming: Manual searches and reviews are labor-intensive and require significant time and effort.
Inconsistent Quality: The quality of literature can vary, making it difficult to determine which sources are credible and relevant.
Lack of Customization: Traditional monitoring methods may not offer personalized content tailored to specific research interests or needs.
Difficulty in Extraction and Analysis: Extracting and analyzing relevant data from a multitude of sources can be daunting and prone to errors.
The Role of AI and ML in Literature Monitoring:
AI and ML technologies offer solutions to the challenges of traditional literature monitoring. By automating data collection, analysis, and retrieval processes, these technologies can enhance the efficiency and effectiveness of literature monitoring. Here’s how AI and ML can improve the literature monitoring landscape:
1. Automated Data Collection
One of the significant advantages of AI is its ability to automate data collection from various sources. Machine learning algorithms can be programmed to scan databases, journals, and online repositories for newly published literature based on specific keywords or topics of interest.
Web Scraping: AI tools can perform web scraping to gather data from multiple sources, including academic journals, preprint servers, and institutional repositories.
RSS Feeds and Alerts: AI can be used to set up alerts and RSS feeds that notify researchers of new publications relevant to their interests, saving time and ensuring they don't miss critical updates.
2. Natural Language Processing (NLP)
Natural Language Processing, a branch of AI, enables machines to understand and interpret human language. NLP can significantly enhance literature monitoring by analyzing and categorizing large volumes of text.
Content Summarization: NLP algorithms can summarize lengthy articles, extracting key points, findings, and conclusions. This allows researchers to quickly assess the relevance of a publication without reading the entire text.
Sentiment Analysis: AI can analyze the sentiment of literature, identifying positive, negative, or neutral tones. This can be particularly useful in fields like social sciences, where researchers may want to gauge public opinion on specific topics.
3. Enhanced Search and Retrieval
Traditional search methods often rely on keyword matching, which can lead to irrelevant results. AI and ML can improve search capabilities through more advanced techniques.
Semantic Search: AI-driven semantic search algorithms understand the context and meaning behind queries, providing more accurate and relevant results. This allows researchers to discover literature they may not have found using traditional keyword searches.
Recommendation Systems: Machine learning algorithms can analyze user behavior and preferences to recommend relevant articles and publications. This personalized approach enhances the likelihood of discovering valuable literature.
4. Data Analysis and Visualization
AI and ML can analyze large datasets quickly and effectively, allowing researchers to gain insights from literature that would be impossible to achieve manually.
Trend Analysis: ML algorithms can identify trends and patterns in research topics over time. This can help researchers understand emerging areas of interest and align their work accordingly.
Data Visualization: AI tools can create visual representations of data, such as graphs and charts, making it easier for researchers to interpret complex information and share findings with others.
5. Quality Assessment
Assessing the quality and credibility of literature is crucial for researchers. AI can help streamline this process through various methods.
Citation Analysis: AI can analyze citation patterns to determine the influence and relevance of specific articles. Highly cited papers may indicate significant contributions to a field.
Peer Review Automation: AI can assist in the peer review process by identifying potential reviewers based on their expertise and previous publications. This ensures a more efficient and thorough review process.
6. Integration with Existing Tools
AI and ML technologies can be integrated with existing literature monitoring tools and platforms to enhance their functionality.
Reference Management Software: Integrating AI into reference management tools can improve citation accuracy and streamline the process of managing bibliographies.
Collaborative Platforms: AI can enhance collaborative platforms, enabling researchers to share findings, discuss literature, and track group interests more effectively.
Practical Applications of AI and ML in Literature Monitoring:
Several organizations and platforms are leveraging AI and ML to enhance literature monitoring. Here are some practical applications:
1. Research Databases
Many research databases are incorporating AI technologies to improve search and retrieval processes. For example, platforms like PubMed and Scopus use machine learning algorithms to enhance their search capabilities and recommend relevant literature to users.
2. Content Aggregators
AI-powered content aggregators automatically collect and organize research articles from various sources. Tools like ResearchGate and Google Scholar utilize AI to suggest relevant articles based on user profiles and interests.
3. Literature Review Platforms
Platforms like Lit maps and EndNote offer AI-driven literature review capabilities, enabling researchers to visualize connections between articles, track citations, and discover related literature efficiently.
4. Text Mining Tools
Text mining tools utilize NLP to extract valuable information from vast amounts of text. Tools like VOS viewer and NVivo can help researchers analyze qualitative data from literature, revealing themes, patterns, and insights.
Challenges and Considerations
While AI and ML offer significant advantages for literature monitoring, there are also challenges and considerations to keep in mind:
Data Privacy and Security: Researchers must be mindful of data privacy concerns when utilizing AI tools that collect and analyze literature. Ensuring compliance with data protection regulations is essential.
Quality of Algorithms: The effectiveness of AI and ML tools depends on the quality of the algorithms used. Researchers should choose reputable tools that provide reliable results.
Interpretation of Results: AI-generated insights may require careful interpretation. Researchers should critically evaluate the output of AI tools to ensure they align with their research objectives.
Continuous Learning: AI and ML technologies are continuously evolving. Researchers should stay updated on advancements and best practices to maximize the benefits of these tools.
Future Trends in Literature Monitoring with AI and ML:
As technology continues to advance, the future of literature monitoring will likely see even more innovative applications of AI and ML. Here are some potential trends to watch for:
Increased Automation: Expect further automation of literature monitoring processes, allowing researchers to focus more on analysis and interpretation rather than data collection.
Enhanced Collaboration: AI-driven collaborative platforms will enable researchers to share insights, discuss findings, and work together more effectively across disciplines.
Personalized Research Experiences: AI will continue to enhance personalized research experiences, tailoring content and recommendations to individual user preferences and needs.
Integration of Big Data: The integration of big data analytics with literature monitoring will enable researchers to uncover deeper insights and connections across various fields.
Ethical AI Practices: As AI technologies advance, there will be a growing emphasis on ethical AI practices, ensuring that algorithms are fair, transparent, and accountable.
Conclusion:
AI and ML technologies have the potential to revolutionize literature monitoring by addressing the challenges of traditional methods and enhancing efficiency and effectiveness. By automating data collection, improving search capabilities, and providing insightful analysis, these technologies empower researchers to stay informed and make informed decisions based on the latest literature. As advancements continue, embracing AI and ML in literature monitoring will become increasingly essential for researchers and professionals seeking to navigate the complexities of the ever-evolving knowledge landscape. With the right tools and strategies, researchers can enhance their literature monitoring processes and unlock new opportunities for discovery and innovation.
Comments