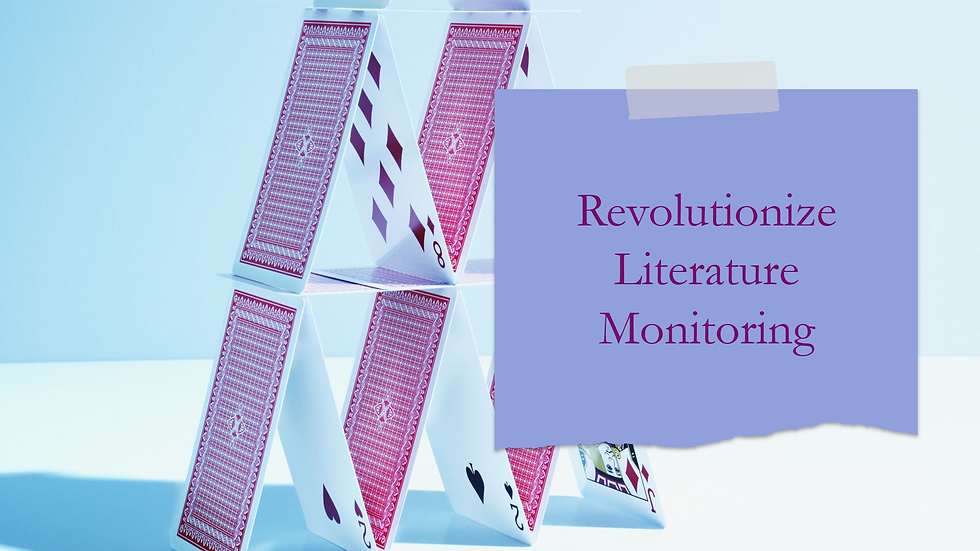
In an era of information overload, organizations across industries face the challenge of sifting through vast volumes of scientific literature to extract relevant insights. In healthcare, pharmacovigilance, and regulatory affairs, literature monitoring plays a crucial role in ensuring drug safety, compliance, and the identification of adverse events. With thousands of new articles published daily, traditional manual methods of literature review are no longer sustainable or efficient. Fortunately, Artificial Intelligence (AI) and Machine Learning (ML) have emerged as transformative tools for enhancing literature monitoring, providing solutions that are faster, more accurate, and scalable.
AI and ML technologies can streamline the process of literature review by automating the identification, extraction, and analysis of relevant information from large volumes of unstructured text. These technologies not only reduce the time spent on manual review but also improve the precision and consistency of data extraction. In this blog, we will explore how AI and ML can be used to enhance literature monitoring, focusing on key benefits such as automation, natural language processing (NLP), predictive analytics, and integration with existing systems.
The Need for Enhanced Literature Monitoring
In industries like pharmacovigilance, regulatory compliance, and clinical research, staying up to date with the latest literature is essential for ensuring product safety and compliance. Literature monitoring helps organizations:
Identify potential safety signals and adverse events.
Stay informed about new studies, trials, and drug efficacy reports.
Maintain regulatory compliance by tracking relevant publications.
Respond to inquiries and safety concerns in a timely manner.
However, the sheer volume of scientific publications, journals, and reports makes manual monitoring increasingly difficult. Human reviewers face challenges like information overload, inconsistencies in data interpretation, and the risk of missing critical information. AI and ML address these challenges by offering automated, intelligent solutions that can process and analyze vast amounts of data with precision.
How AI and ML Enhance Literature Monitoring
1. Automation of Data Extraction
One of the primary benefits of AI in literature monitoring is the ability to automate the extraction of relevant information from unstructured text. Traditional literature review methods require human reviewers to manually read through articles and extract key data points, such as adverse event reports, patient demographics, or clinical trial results. This process is time-consuming and prone to human error.
AI-powered algorithms can automatically scan and extract relevant information from scientific articles, abstracts, and clinical trial reports. These algorithms are trained to recognize specific keywords, phrases, and patterns that are relevant to the organization’s needs. Once the data is extracted, it can be categorized and stored for further analysis.
Key Benefits:
Faster Data Extraction: AI systems can process large volumes of text in a fraction of the time it would take a human reviewer, significantly reducing the time required for literature review.
Consistency: AI ensures consistent extraction of data across all documents, reducing variability and human error.
Scalability: AI systems can easily scale to handle increasing volumes of literature, making them ideal for organizations dealing with large datasets.
2. Natural Language Processing (NLP) for Text Understanding
Natural Language Processing (NLP) is a subset of AI that focuses on enabling computers to understand and interpret human language. In literature monitoring, NLP plays a critical role in processing unstructured text, such as research articles, clinical trial reports, and case studies. NLP algorithms can identify relevant concepts, entities, and relationships within the text, making it easier to extract meaningful insights.
NLP techniques such as named entity recognition (NER) and sentiment analysis can be applied to identify specific entities like drug names, adverse events, and patient demographics. This allows organizations to quickly pinpoint relevant sections of a document and focus their review efforts on the most critical information.
Key Benefits:
Efficient Information Retrieval: NLP can search through entire databases of literature to find relevant articles based on specific search criteria, reducing the need for manual searching.
Contextual Understanding: NLP algorithms can interpret the context of a document, ensuring that the extracted information is accurate and relevant to the specific use case.
Improved Accuracy: By understanding the language used in scientific literature, NLP improves the accuracy of data extraction and reduces the risk of misinterpretation.
3. AI-Driven Predictive Analytics
In addition to automating data extraction, AI and ML can be used to predict trends and identify potential safety signals based on the information contained in the literature. Predictive analytics models can analyze patterns in scientific articles and clinical trial reports to identify emerging risks or trends that may not be immediately apparent through manual review.
For example, in pharmacovigilance, predictive analytics can help identify patterns in adverse event reporting across multiple studies, enabling organizations to take proactive steps to mitigate potential safety risks. These models can also predict future trends in drug efficacy, side effects, or patient outcomes based on historical data.
Key Benefits:
Proactive Risk Management: Predictive analytics allows organizations to identify potential safety concerns before they escalate, enabling proactive risk management and response.
Data-Driven Decision Making: By providing insights into emerging trends, AI-driven analytics support data-driven decision-making processes, improving the overall quality of safety monitoring.
Continuous Learning: AI models can continuously learn from new data, improving their predictive capabilities over time and ensuring that they stay up to date with the latest literature.
4. Integration with Existing Systems
One of the key challenges in adopting AI and ML for literature monitoring is ensuring that these technologies can seamlessly integrate with existing systems and workflows. AI-powered literature monitoring tools can be integrated with existing databases, document management systems, and regulatory compliance platforms, allowing organizations to streamline their workflows and reduce duplication of effort.
By integrating AI and ML into their literature monitoring systems, organizations can automate the entire process, from data extraction to analysis and reporting. This integration ensures that all relevant literature is captured and analyzed in real-time, reducing the risk of missing critical information.
Key Benefits:
Streamlined Workflows: Integration with existing systems ensures that AI-powered tools can be easily adopted without disrupting current workflows.
Real-Time Monitoring: By integrating AI with real-time data sources, organizations can continuously monitor the latest literature and receive alerts when new relevant publications are identified.
Improved Collaboration: AI-powered tools can be integrated with collaboration platforms, enabling teams to share insights and work together more effectively.
Key Considerations for Implementing AI and ML in Literature Monitoring
While AI and ML offer numerous benefits for enhancing literature monitoring, organizations must consider several factors when implementing these technologies. These considerations include data quality, algorithm training, regulatory compliance, and ethical concerns.
1. Data Quality and Availability
The effectiveness of AI and ML models depends heavily on the quality and availability of data. Organizations must ensure that the literature they are using to train AI algorithms is comprehensive, accurate, and up to date. Incomplete or biased data can lead to inaccurate results and missed safety signals.
2. Algorithm Training and Validation
AI algorithms must be trained on large datasets to accurately identify relevant information and patterns. Organizations should invest in proper training and validation processes to ensure that their AI models are reliable and accurate. This may involve collaboration with subject matter experts to refine the algorithms and validate their outputs.
3. Regulatory Compliance
In industries like pharmacovigilance and healthcare, organizations must ensure that their AI-powered literature monitoring systems comply with regulatory requirements. This includes adherence to guidelines set forth by regulatory bodies such as the U.S. Food and Drug Administration (FDA) and the European Medicines Agency (EMA). AI systems must be transparent, explainable, and capable of generating audit trails to ensure compliance with regulatory standards.
4. Ethical Considerations
As AI and ML become more prevalent in literature monitoring, organizations must consider the ethical implications of using these technologies. This includes ensuring that AI models are not biased, that patient privacy is protected, and that the use of AI does not lead to job displacement or reduced accountability.
Future Trends in AI and ML for Literature Monitoring
The future of literature monitoring is likely to be shaped by ongoing advancements in AI and ML. As these technologies continue to evolve, we can expect to see several key trends emerge, including:
Increased Use of Deep Learning: Deep learning models, a subset of ML, are expected to play a larger role in literature monitoring by providing even more accurate and nuanced insights from unstructured text.
AI-Powered Knowledge Graphs: Knowledge graphs that map relationships between different entities (e.g., drugs, diseases, and adverse events) can help organizations visualize and understand complex relationships in the literature.
Automation of Regulatory Reporting: AI and ML will increasingly be used to automate the generation of regulatory reports based on the information extracted from literature, reducing the time and effort required for compliance reporting.
Conclusion:
AI and ML have revolutionized literature monitoring by automating data extraction, improving accuracy, and enabling predictive insights. As the volume of scientific literature continues to grow, these technologies offer organizations a scalable, efficient solution for staying on top of the latest developments. By leveraging AI and ML, organizations can enhance their literature monitoring processes, reduce the risk of missing critical information, and ensure compliance with regulatory requirements.
The integration of AI into literature monitoring represents a significant step forward, providing faster, more accurate, and proactive ways to manage the ever-growing body of scientific knowledge.
Comentarios