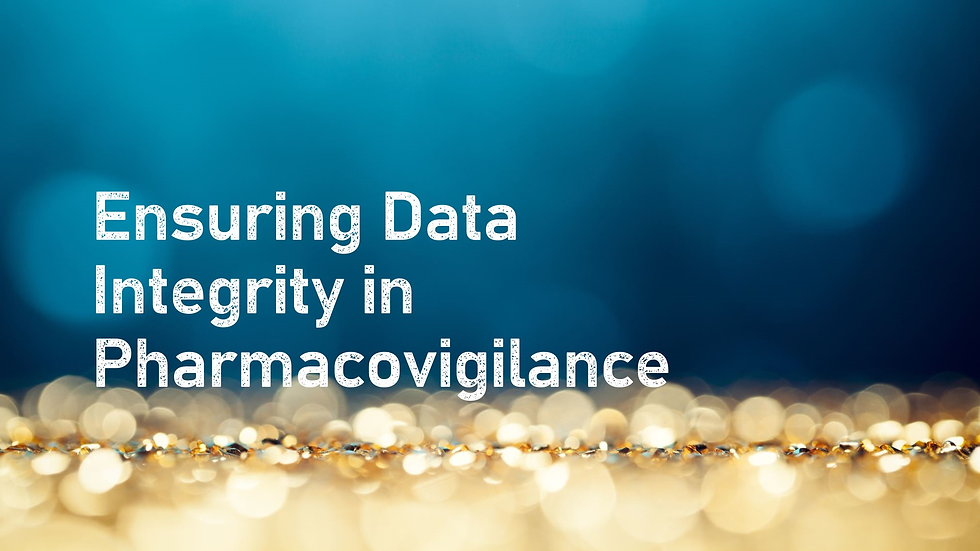
In the realm of pharmacovigilance, ensuring the integrity of data is not just a regulatory requirement but a critical component in safeguarding public health. Pharmacovigilance, the science and activities related to the detection, assessment, understanding, and prevention of adverse effects or any other drug-related problems, relies heavily on accurate and reliable data. Here’s a detailed guide on how to ensure data integrity in pharmacovigilance databases.
1. Understanding Data Integrity in Pharmacovigilance
Data integrity in pharmacovigilance refers to the accuracy, completeness, and consistency of data throughout its lifecycle. It encompasses aspects like data entry, storage, retrieval, and reporting. Maintaining data integrity is crucial because it ensures that the information used to assess the safety of pharmaceuticals is reliable, which in turn influences regulatory decisions and public health outcomes.
2. Regulatory Framework and Standards
Adherence to regulatory guidelines and standards is foundational to ensuring data integrity. Key regulatory bodies such as the FDA (Food and Drug Administration), EMA (European Medicines Agency), and ICH (International Council for Harmonization) provide comprehensive guidelines:
Good Clinical Practice (GCP): Ensures that clinical trials are conducted ethically and data is accurate.
Good Manufacturing Practice (GMP): Covers aspects related to drug production and control.
Good Pharmacovigilance Practice (GVP): Focuses specifically on the safety and efficacy of medicinal products post-marketing.
Compliance with these guidelines helps in setting up a robust framework for maintaining data integrity.
3. Implementing Robust Data Entry Protocols
Data entry is the first step in the data lifecycle and sets the stage for data integrity. Implementing stringent data entry protocols is essential:
Standardized Forms: Use standardized forms and electronic data capture (EDC) systems to reduce variability in data entry.
Training: Provide comprehensive training to personnel on data entry procedures and the importance of data accuracy.
Validation Checks: Implement real-time validation checks to identify and correct errors during data entry.
4. Data Storage and Security
Once data is entered into the system, it needs to be stored securely to prevent unauthorized access and tampering:
Access Controls: Implement role-based access controls to ensure that only authorized personnel can access sensitive data.
Data Encryption: Use encryption techniques to protect data both at rest and in transit.
Regular Backups: Schedule regular backups to prevent data loss in case of system failures or disasters.
5. Data Quality Assurance Processes
To maintain data integrity, ongoing quality assurance processes are critical:
Regular Audits: Conduct regular internal and external audits to identify and rectify potential issues.
Data Reconciliation: Periodically reconcile data across different sources to ensure consistency and accuracy.
Error Tracking: Implement systems to track and analyze data errors, which can help in identifying root causes and preventing recurrence.
6. Data Integrity in Electronic Systems
With the increasing use of electronic systems in pharmacovigilance, ensuring data integrity in these systems is paramount:
System Validation: Validate electronic systems to ensure they meet the required specifications and perform as expected.
Audit Trails: Maintain audit trails that document all changes made to the data, including the user who made the change, the date, and the reason.
Data Backups: Ensure regular backups of electronic systems and test the restore process to confirm data recovery capabilities.
7. Addressing Data Integrity Issues
Despite best efforts, data integrity issues may still arise. Addressing these issues promptly is crucial:
Root Cause Analysis: Conduct thorough investigations to identify the root cause of data integrity issues.
Corrective and Preventive Actions (CAPA): Implement corrective actions to address immediate issues and preventive actions to avoid future occurrences.
Documentation: Document all issues, investigations, and actions taken to ensure transparency and facilitate future audits.
8. Training and Culture
A culture of data integrity begins with education and training:
Ongoing Training: Provide continuous training to staff on the importance of data integrity and best practices.
Ethical Culture: Foster an organizational culture that prioritizes ethical practices and integrity in data management.
Communication: Encourage open communication about data integrity issues and promote a proactive approach to problem-solving.
9. Collaboration and Communication
Effective collaboration and communication among stakeholders can enhance data integrity:
Cross-Functional Teams: Work with cross-functional teams, including clinical, regulatory, and IT departments, to ensure comprehensive data management.
Stakeholder Engagement: Engage with regulatory authorities, industry peers, and other stakeholders to stay updated on best practices and regulatory changes.
Feedback Mechanisms: Implement feedback mechanisms to gather insights from users and improve data management practices.
10. Technology and Innovations
Embracing new technologies and innovations can help in maintaining data integrity:
Artificial Intelligence (AI): Utilize AI and machine learning to enhance data analysis, identify patterns, and detect anomalies.
Blockchain: Explore the use of blockchain technology to create immutable records and improve data transparency.
Automated Tools: Implement automated tools for data validation, monitoring, and reporting to reduce human error.
11. Case Studies and Best Practices
Examining real-world case studies and best practices can provide valuable insights into maintaining data integrity:
Case Study 1: A pharmaceutical company implemented a new EDC system with robust validation checks and access controls, resulting in a significant reduction in data entry errors and improved compliance.
Case Study 2: An organization faced data integrity issues due to inadequate training. By enhancing their training programs and fostering a culture of data integrity, they successfully addressed the issues and improved data quality.
12. Future Trends and Challenges
Looking ahead, several trends and challenges may impact data integrity in pharmacovigilance:
Increasing Data Volume: The growing volume of data from various sources, including real-world evidence, poses challenges in data management and quality control.
Regulatory Changes: Evolving regulatory requirements will necessitate continuous adaptation of data management practices.
Technological Advancements: While technology offers opportunities for enhancing data integrity, it also presents new challenges related to system integration and security.
Conclusion:
Ensuring data integrity in pharmacovigilance databases is a multifaceted process that requires adherence to regulatory standards, implementation of robust protocols, and continuous monitoring and improvement. By focusing on data entry, storage, quality assurance, and leveraging technological advancements, organizations can maintain the highest standards of data integrity. Ultimately, safeguarding data integrity is not just about compliance but is essential for protecting public health and ensuring the efficacy and safety of medicinal products.
Comments