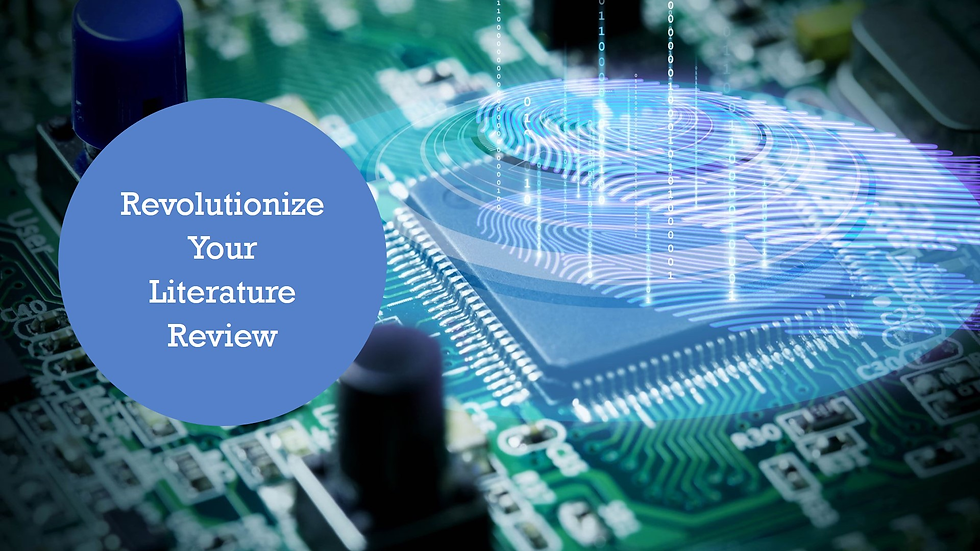
In today's data-driven world, the role of literature reviews has expanded far beyond academia. They are essential not only in scientific research but also in industries such as healthcare, pharmacovigilance, and business strategy, where access to the latest developments is critical. However, the traditional process of reviewing vast quantities of data is time-consuming and prone to human error. This is where Artificial Intelligence (AI) becomes a game-changer, offering tools to streamline, optimize, and enhance the literature review process. In this blog, we explore how to ensure an effective literature review with AI, focusing on best practices, challenges, and technologies that maximize efficiency and accuracy.
1. What is an AI-Enhanced Literature Review?
An AI-enhanced literature review uses machine learning (ML), natural language processing (NLP), and automation to assist researchers in systematically identifying, analyzing, and summarizing relevant information. It involves algorithms that can sift through large databases, extract meaningful insights, and present the most relevant research papers or documents in an organized manner.
These AI-powered systems not only accelerate the review process but also reduce the risk of missing critical data. Whether in pharmacovigilance, regulatory compliance, or academic research, AI offers a significant advantage over manual methods by automating repetitive tasks, identifying trends, and providing real-time updates.
2. Why is AI Critical for Literature Reviews?
AI technology is transforming the way literature reviews are conducted by addressing several pain points in traditional processes:
Volume of Data: Modern researchers must deal with exponentially growing data in scientific publications, journals, and reports.
Time Constraints: Manual review of large datasets requires considerable time, creating bottlenecks in workflows.
Risk of Human Bias: Subjective selection of sources or oversight can lead to incomplete reviews.
Dynamic Research Environment: In fast-moving industries like medical research, timely access to the latest data is critical for decision-making.
AI can help overcome these challenges by enabling faster, unbiased, and more accurate literature reviews.
3. Best Practices for Implementing AI in Literature Review
Implementing AI for literature review requires thoughtful planning and attention to detail. Here are some best practices to ensure effectiveness:
3.1 Define Objectives and Scope Clearly
Before deploying AI tools, it is essential to determine the specific objectives of the literature review. For example, in pharmacovigilance, the goal may be to identify adverse drug reactions or safety signals. In academia, the focus could be on identifying gaps in existing research. Defining the scope ensures that the AI system targets the right keywords, publications, and sources.
3.2 Select the Right AI Tools
Different tools cater to different types of literature reviews. Some popular AI-based tools include:
Connected Papers: Visualizes connections between research papers.
Semantic Scholar: Uses NLP to extract insights from scientific literature.
Iris.ai: Provides automated research mapping and text extraction.
Rayyan: Facilitates systematic reviews by simplifying article screening.
Evaluate tools based on your specific requirements, such as keyword search capabilities, citation analysis, or integration with databases like PubMed.
3.3 Leverage Natural Language Processing (NLP) Algorithms
NLP algorithms allow AI systems to understand human language. These algorithms are used to identify relevant keywords, categorize topics, and summarize key findings from large datasets. NLP-powered AI tools can automatically filter research papers based on relevance, providing only those that meet your criteria.
3.4 Implement Data Quality Control Mechanisms
While AI reduces human error, it still requires oversight. Implement quality control mechanisms to validate the outputs of AI models. Researchers should periodically review results to ensure that important studies are not missed and irrelevant materials are filtered out effectively.
3.5 Incorporate Human Oversight for Critical Decision-Making
AI tools can significantly reduce the workload, but human expertise is still necessary for making informed decisions, interpreting nuanced information, and finalizing the conclusions of a literature review. A collaborative approach—where researchers work alongside AI systems—ensures a comprehensive review.
4. Key Features of Effective AI-Powered Literature Review Systems
To ensure the AI system performs effectively, certain features must be prioritized during implementation. These include:
4.1 Automation of Routine Tasks
AI can automate repetitive tasks such as keyword searches, metadata extraction, and bibliography management. This saves time and enables researchers to focus on analysis and interpretation.
4.2 Advanced Search Capabilities
Effective literature review tools should support advanced search queries, including Boolean operators (AND, OR, NOT), phrase matching, and wildcard searches. This allows for more refined searches to yield the most relevant data.
4.3 Real-Time Updates
AI tools can be configured to monitor publications continuously and provide real-time alerts on new developments. This feature is particularly useful in fast-paced industries like pharmacovigilance, where the latest research may affect regulatory actions.
4.4 Summarization and Clustering Algorithms
Summarization algorithms condense lengthy research articles into key points, making it easier for researchers to understand the core message. Similarly, clustering algorithms group related studies, helping researchers identify themes and trends across multiple papers.
4.5 Integration with Databases and Citation Management Tools
The AI system should seamlessly integrate with existing databases (e.g., PubMed, IEEE Xplore) and citation management software (e.g., Mendeley, EndNote). This ensures a smooth workflow from literature search to reference management.
5. Challenges and Limitations of AI in Literature Reviews
Despite the many advantages of AI, there are also challenges and limitations to consider:
5.1 Limited Contextual Understanding
AI systems may struggle with understanding the context or nuance of some research topics, leading to incorrect categorization or exclusion of relevant papers.
5.2 Data Quality Issues
AI models depend on the quality of the datasets they are trained on. Poorly indexed databases or incomplete metadata can reduce the effectiveness of AI tools.
5.3 Bias in Algorithms
AI models are not immune to bias. If the underlying data used to train the algorithms is biased, the results of the literature review may also reflect those biases.
5.4 Learning Curve for Users
Researchers may need time to become familiar with AI tools. Proper training and onboarding are essential to ensure smooth adoption.
5.5 Cost and Resource Constraints
While AI-powered tools can save time in the long run, there may be initial costs associated with purchasing software, training staff, and integrating AI into existing workflows.
6. Applications of AI-Enhanced Literature Review in Different Fields
AI-enhanced literature reviews are transforming multiple industries by providing insights and improving efficiency. Some examples include:
6.1 Healthcare and Pharmacovigilance
In pharmacovigilance, AI tools help identify adverse drug reactions from the latest publications, ensuring timely updates to safety profiles. Automated screening reduces the chances of missing critical reports that could affect patient safety.
6.2 Business and Market Research
AI tools allow businesses to stay ahead of industry trends by monitoring the latest research and market reports. They assist in gathering competitive intelligence and identifying emerging opportunities.
6.3 Academia and Scientific Research
AI supports academic researchers by automating the process of identifying literature gaps, organizing citations, and summarizing large volumes of text, thus accelerating research timelines.
6.4 Regulatory Compliance and Risk Management
AI tools play a crucial role in helping organizations maintain compliance with regulatory standards. They assist in monitoring new guidelines and ensuring timely responses to regulatory changes.
7. Future Trends in AI-Powered Literature Reviews
The future of AI in literature review lies in further advancements in NLP, machine learning, and automation. Some anticipated trends include:
AI-Driven Systematic Reviews: Complete automation of systematic reviews with minimal human intervention.
Voice-Activated Literature Search: Hands-free search capabilities using natural language commands.
Personalized Literature Recommendations: AI systems providing tailored recommendations based on a researcher’s specific interests.
Improved Sentiment Analysis: Advanced sentiment analysis to detect subtle shifts in research trends and public perception.
8. Conclusion
AI is transforming the way literature reviews are conducted by improving speed, accuracy, and efficiency. By automating repetitive tasks, providing real-time updates, and offering advanced search capabilities, AI ensures that researchers and professionals across industries can stay ahead of the curve. However, the effective use of AI also requires careful planning, quality control, and human oversight. When implemented correctly, AI-powered literature reviews can significantly enhance decision-making, reduce workload, and uncover insights that would otherwise remain hidden.
As AI continues to evolve, it promises even more sophisticated tools and applications that will further revolutionize the way literature reviews are performed. Embracing this technology is not just an option—it’s a necessity for staying competitive and informed in today’s fast-paced world.
Comments