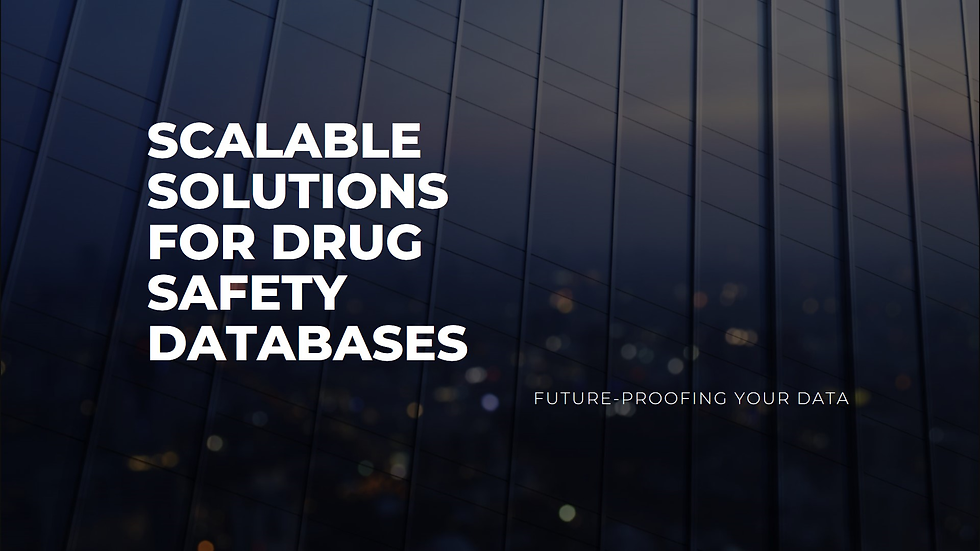
In the fast-evolving world of pharmaceuticals, the demand for effective drug safety measures has never been higher. As the complexity of drug development increases, so does the need for robust systems to monitor, analyze, and act upon safety data. Drug safety databases play a critical role in this process, acting as repositories for adverse event reports, clinical trial data, and other critical safety information. However, as these databases grow in size and complexity, they face numerous challenges that can impede their effectiveness. Future-proofing these databases with scalable solutions is essential to ensure that they can handle the increasing demands placed upon them.
The Growing Importance of Drug Safety Databases:
Drug safety databases are central to the pharmacovigilance process, which involves the detection, assessment, understanding, and prevention of adverse effects or any other drug-related problems. These databases store vast amounts of data from various sources, including clinical trials, post-marketing surveillance, and spontaneous reports from healthcare professionals and patients.
With the increasing volume of data being generated, the challenge is not just about storing this information but also ensuring that it can be accessed, analyzed, and acted upon in a timely manner. Inefficient or outdated databases can lead to delays in identifying potential safety issues, which can have serious consequences for patient safety and regulatory compliance.
Challenges Faced by Traditional Drug Safety Databases:
Traditional drug safety databases were often designed with specific, limited purposes in mind. As a result, they may not be equipped to handle the scale and complexity of modern pharmacovigilance needs. Some of the key challenges faced by these databases include:
Data Volume and Variety: The amount of safety data being generated has grown exponentially, driven by the increasing number of clinical trials, expanded post-marketing surveillance, and the rise of real-world evidence. This data is also highly varied, encompassing structured data (e.g., clinical trial results) and unstructured data (e.g., social media posts, medical records).
Scalability: Many traditional databases struggle to scale effectively, leading to performance issues as the volume of data grows. This can result in slow query times, difficulty in handling peak loads, and challenges in integrating new data sources.
Interoperability: Drug safety databases often need to integrate with other systems, such as electronic health records (EHRs), regulatory databases, and data from international partners. Ensuring interoperability between these diverse systems can be complex and time-consuming.
Data Quality and Consistency: Maintaining high data quality is critical for accurate safety assessments. However, data quality issues, such as incomplete or inconsistent records, can undermine the reliability of safety analyses.
Compliance and Security: Drug safety databases must comply with stringent regulatory requirements, such as those set by the FDA, EMA, and other regulatory bodies. Ensuring data security and privacy is also paramount, especially given the sensitive nature of the information being handled.
The Need for Scalable Solutions:
Given these challenges, it is clear that future-proofing drug safety databases requires scalable solutions that can adapt to the evolving needs of the industry. Scalability in this context refers to the ability of a system to handle increasing amounts of data, users, and complexity without compromising performance or functionality.
Key Components of a Scalable Drug Safety Database:
To future-proof drug safety databases, organizations should focus on the following key components:
Cloud-Based Infrastructure: Moving to a cloud-based infrastructure can provide the scalability needed to handle large volumes of data. Cloud platforms offer on-demand resources, allowing databases to scale up or down as needed. This flexibility can help manage peak loads, such as when a new drug is launched or when a safety signal is detected that requires rapid analysis.
Data Integration and Interoperability: A scalable drug safety database should be capable of integrating data from multiple sources, including EHRs, regulatory databases, and patient-reported outcomes. Utilizing standardized data formats and interoperability frameworks, such as the International Organization for Standardization (ISO) standards or Health Level Seven International (HL7), can facilitate seamless data exchange.
Advanced Analytics and Artificial Intelligence: Incorporating advanced analytics and AI can enhance the ability to detect and analyze safety signals. Machine learning algorithms can be trained to identify patterns in large datasets, enabling the early detection of potential adverse events. Natural language processing (NLP) can also be used to extract valuable insights from unstructured data sources, such as social media and medical literature.
Data Quality Management: Implementing robust data quality management practices is essential for ensuring the accuracy and consistency of safety data. This includes automated data cleansing processes, real-time validation, and standardized data entry protocols. Ensuring high data quality will enhance the reliability of safety assessments and regulatory submissions.
Security and Compliance: A future-proof drug safety database must be designed with security and compliance in mind. This includes implementing encryption, access controls, and audit trails to protect sensitive data. Additionally, the database should be regularly updated to comply with evolving regulatory requirements, such as those related to data privacy (e.g., GDPR) and pharmacovigilance (e.g., the ICH E2E guidelines).
The Role of Artificial Intelligence and Machine Learning:
Artificial intelligence (AI) and machine learning (ML) are increasingly being recognized as transformative technologies in the field of drug safety. These technologies can help future-proof drug safety databases by enabling more efficient data analysis and improving the accuracy of safety signal detection.
AI for Signal Detection
One of the most critical tasks in pharmacovigilance is signal detection—the process of identifying new, rare, or unexpected adverse events associated with a drug. Traditionally, this has been a labor-intensive process, requiring manual review of large datasets. However, AI can automate and enhance this process by:
Analyzing Large Datasets: AI algorithms can quickly analyze vast amounts of data from multiple sources, identifying patterns and correlations that might be missed by human analysts.
Prioritizing Signals: AI can help prioritize signals based on factors such as severity, frequency, and patient demographics, allowing pharmacovigilance teams to focus on the most critical issues first.
Reducing False Positives: By continuously learning from new data, AI systems can refine their detection capabilities, reducing the number of false positives and improving the overall accuracy of signal detection.
Machine Learning for Predictive Analytics:
Machine learning, a subset of AI, is particularly well-suited for predictive analytics in drug safety. By analyzing historical data, ML models can identify trends and predict potential safety issues before they become apparent. This can enable proactive risk management and help prevent adverse events.
For example, ML models can be used to predict which patient populations are most likely to experience adverse reactions to a particular drug, allowing for more targeted post-marketing surveillance. Additionally, ML can be used to assess the potential impact of safety signals, helping regulatory agencies and pharmaceutical companies make more informed decisions about drug safety.
The Importance of Data Governance:
As drug safety databases become more complex and incorporate more advanced technologies, the importance of data governance cannot be overstated. Data governance refers to the overall management of data availability, usability, integrity, and security in an organization. In the context of drug safety databases, strong data governance practices are essential for ensuring that data is accurate, consistent, and compliant with regulatory requirements.
Key Data Governance Practices:
To future-proof drug safety databases, organizations should implement the following data governance practices:
Data Stewardship: Assigning data stewards who are responsible for overseeing data quality, consistency, and compliance within the database.
Data Cataloging: Creating a data catalog that documents the sources, formats, and definitions of all data within the database, making it easier to manage and analyze.
Data Privacy and Security: Implementing strong data privacy and security measures, including encryption, access controls, and regular security audits.
Compliance Monitoring: Regularly monitoring and auditing the database to ensure compliance with regulatory requirements and industry best practices.
The Future of Drug Safety Databases
The future of drug safety databases lies in their ability to adapt to the rapidly changing landscape of pharmacovigilance. As new technologies emerge and the volume of safety data continues to grow, databases must be able to scale and evolve to meet these challenges. By adopting scalable solutions, such as cloud-based infrastructure, AI-driven analytics, and robust data governance practices, organizations can future-proof their drug safety databases and ensure that they remain effective tools for protecting patient safety.
Conclusion:
Future-proofing drug safety databases is a critical step in ensuring the ongoing effectiveness of pharmacovigilance efforts. As the pharmaceutical industry continues to evolve, the demands placed on these databases will only increase. By implementing scalable solutions that address the challenges of data volume, interoperability, data quality, and security, organizations can build drug safety databases that are capable of meeting the needs of today and tomorrow. Furthermore, the integration of AI and machine learning technologies can significantly enhance the ability to detect and respond to safety signals, ultimately leading to better patient outcomes and greater regulatory compliance. The journey to future-proof drug safety databases is not without its challenges, but with the right strategies and technologies in place, it is a journey well worth undertaking.
Comments