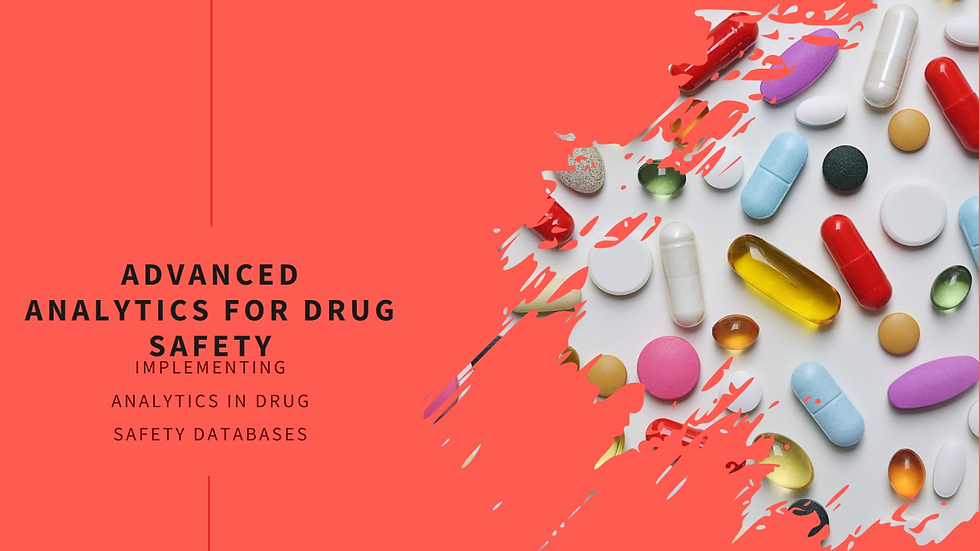
Drug safety databases play a pivotal role in monitoring adverse drug reactions (ADRs), evaluating drug efficacy, and ensuring patient safety throughout the drug development lifecycle. Advanced analytics, including machine learning (ML), natural language processing (NLP), and predictive modeling, empower pharmaceutical companies to extract valuable insights from vast datasets to inform regulatory decisions and improve healthcare outcomes.
Importance of Advanced Analytics in Pharmacovigilance:
Advanced analytics in drug safety databases are essential for:
Early Detection of Safety Signals: Identifying potential ADRs and safety concerns early to facilitate timely interventions and risk mitigation strategies.
Enhanced Signal Detection: Analyzing large volumes of structured and unstructured data to detect subtle patterns and signals that may indicate previously unrecognized ADRs.
Predictive Risk Assessment: Predicting patient-specific risks and identifying populations at higher risk of adverse events based on demographic, clinical, and genetic data.
Implementation Strategies for Advanced Analytics:
Implementing advanced analytics requires a strategic approach to leverage data effectively and derive actionable insights for drug safety monitoring and regulatory reporting.
1. Data Integration and Standardization
Integrate diverse data sources, including clinical trials, electronic health records (EHRs), spontaneous reports, and social media, to create comprehensive datasets for analysis. Standardize data formats and terminologies to ensure consistency and compatibility across different sources.
Example: Unified Data Platforms
Implement unified data platforms that consolidate structured and unstructured data from internal and external sources. These platforms facilitate seamless data integration, preparation, and analysis for advanced analytics.
2. Selection of Analytical Tools and Technologies
Choose appropriate analytical tools and technologies, such as machine learning algorithms, NLP engines, and data visualization tools, based on the complexity and objectives of the analysis. Leverage cloud-based solutions for scalability and computational power.
Example: AI-Powered Signal Detection
Deploy AI-driven signal detection algorithms to automatically analyze adverse event reports, identify potential safety signals, and prioritize signals for further investigation based on statistical significance and clinical relevance.
3. Development of Predictive Models
Develop predictive models using historical data to forecast future trends in drug safety, predict patient outcomes, and optimize risk management strategies. Train models on large datasets to improve accuracy and reliability of predictions.
Example: Predictive Risk Scores
Create predictive risk scores using ML models to assess the likelihood of adverse events in patient populations exposed to specific drugs. These scores inform personalized healthcare interventions and treatment decisions.
4. Implementation of Real-Time Monitoring Systems
Establish real-time monitoring systems that continuously analyze incoming data streams, such as electronic health records and social media feeds, to detect and respond promptly to emerging safety concerns and ADRs.
Example: Real-Time Surveillance Platforms
Deploy real-time surveillance platforms equipped with anomaly detection algorithms to monitor changes in ADR patterns and medication usage trends. Automated alerts notify healthcare professionals and regulators of potential safety issues.
5. Collaboration and Training
Foster collaboration between data scientists, pharmacovigilance experts, regulatory affairs professionals, and healthcare providers to interpret analytical findings and translate insights into actionable recommendations. Provide training on advanced analytics tools and methodologies to enhance team capabilities.
Example: Cross-Functional Workshops
Organize cross-functional workshops and training sessions to educate stakeholders on the use of advanced analytics in drug safety. Case studies and interactive simulations demonstrate the application of analytics in real-world scenarios.
Benefits of Implementing Advanced Analytics:
1. Improved Signal Detection Efficiency
Advanced analytics enhance signal detection efficiency by automating data analysis processes, reducing manual review efforts, and detecting ADRs that may not be apparent through traditional methods.
2. Enhanced Patient Safety and Risk Management
Early identification of safety signals and predictive risk assessment improve patient safety by enabling timely interventions, risk communication, and regulatory actions to mitigate potential harm.
3. Evidence-Based Decision-Making
Data-driven insights from advanced analytics support evidence-based decision-making in drug development, regulatory submissions, and post-marketing surveillance. Regulatory agencies use analytics to evaluate drug safety profiles and make informed approval decisions.
4. Operational Efficiency and Cost Savings
Streamlined data workflows and automated analytics reduce operational costs associated with manual data entry, review processes, and regulatory compliance. Efficient resource allocation optimizes pharmacovigilance activities and accelerates time-to-market for new drugs.
5. Continuous Improvement and Innovation
Continuous monitoring and analysis of drug safety data foster continuous improvement in pharmacovigilance practices, regulatory strategies, and healthcare innovation. Feedback loops enable iterative refinement of predictive models and analytical methodologies.
Challenges in Implementing Advanced Analytics:
1. Data Quality and Completeness
Ensuring data quality, completeness, and accuracy across disparate data sources pose challenges in implementing advanced analytics. Addressing data inconsistencies and missing values is critical to deriving reliable insights.
2. Regulatory Compliance and Validation
Meeting regulatory requirements for data integrity, privacy protection, and analytical validation is essential for gaining acceptance of advanced analytics in drug safety surveillance and regulatory submissions.
3. Integration with Existing Systems
Integrating advanced analytics platforms with existing IT infrastructure and regulatory reporting systems requires interoperability, scalability, and compatibility with legacy systems.
Future Trends in Advanced Analytics for Drug Safety:
1. Expansion of AI Applications
AI applications, including deep learning and reinforcement learning, will advance predictive modeling capabilities for drug safety, enabling more accurate risk assessments and personalized medicine approaches.
2. Real-Time Data Analytics
Advancements in real-time data analytics will enable continuous monitoring of drug safety signals and rapid response to emerging ADRs, enhancing proactive risk management and patient safety.
3. Interdisciplinary Collaboration
Increased collaboration between data scientists, healthcare providers, regulatory agencies, and technology innovators will drive interdisciplinary approaches to integrating advanced analytics into drug safety practices.
Conclusion:
Implementing advanced analytics in drug safety databases is essential for enhancing pharmacovigilance, improving patient safety, and supporting regulatory compliance in pharmaceutical and healthcare industries. By leveraging AI, ML, and predictive modeling, companies can streamline signal detection, optimize risk management strategies, and accelerate drug development timelines. Overcoming challenges in data integration, regulatory validation, and organizational readiness is crucial for maximizing the benefits of advanced analytics in drug safety surveillance. With continued innovation and collaboration, advanced analytics will shape the future of pharmacovigilance, driving continuous improvement in drug safety monitoring and healthcare outcomes globally.
Comments