How to Implement AI in Literature Monitoring for Compliance
- Chaitali Gaikwad
- Sep 23, 2024
- 6 min read
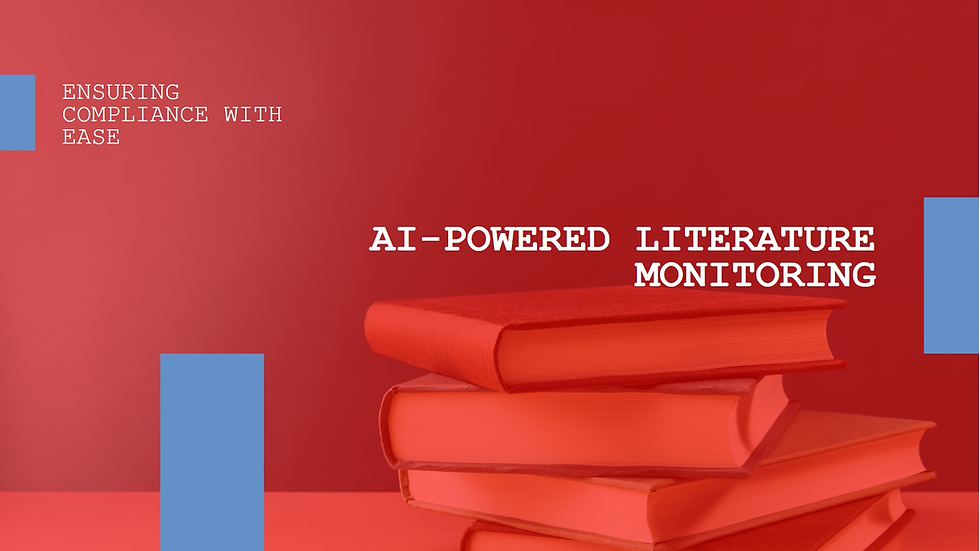
Implementing AI in literature monitoring for compliance is becoming increasingly crucial in industries like pharmaceuticals, finance, and legal services. The vast and growing volume of information available online makes it challenging for compliance teams to stay up-to-date with the latest developments. AI-powered tools can enhance the efficiency, accuracy, and scope of literature monitoring, ensuring that organizations remain compliant with regulations and industry standards. In this blog, we’ll explore the steps to implement AI in literature monitoring, the benefits it offers, and the challenges that may arise during the process.
1. Understanding the Need for AI in Literature Monitoring
Compliance is a critical function in many industries, particularly in highly regulated sectors like pharmaceuticals, finance, and healthcare. Regulatory bodies frequently update guidelines, and new research or case law can have significant implications for an organization's operations. Traditionally, compliance teams have relied on manual processes to monitor literature, which is time-consuming, prone to error, and often inefficient when dealing with large volumes of data.
AI offers a solution to these challenges by automating the process of literature monitoring. AI-powered systems can scan vast amounts of text data from multiple sources, identify relevant information, and alert compliance teams to any potential issues. This not only saves time and resources but also enhances the accuracy and comprehensiveness of compliance monitoring.
2. Key Components of an AI-Powered Literature Monitoring System
Before diving into the implementation process, it’s essential to understand the key components of an AI-powered literature monitoring system:
Natural Language Processing (NLP): NLP enables AI systems to understand and process human language. It allows the system to analyze text data, extract relevant information, and identify patterns or anomalies that may be of interest to compliance teams.
Machine Learning (ML) Algorithms: ML algorithms are used to train the AI system to recognize relevant content. Over time, the system learns from the data it processes, improving its accuracy and efficiency.
Data Integration: An effective AI-powered literature monitoring system must be able to integrate data from multiple sources, including scientific journals, legal databases, news outlets, and social media platforms.
Alerting and Reporting Mechanisms: Once the AI system identifies relevant content, it needs to alert the appropriate personnel. This could be done through automated reports, email alerts, or dashboards.
3. Steps to Implement AI in Literature Monitoring for Compliance
Step 1: Define Objectives and Requirements
The first step in implementing AI in literature monitoring is to clearly define the objectives and requirements. What specific compliance areas are you targeting? What types of literature or data sources are relevant? Defining these parameters upfront will help guide the implementation process and ensure that the AI system is tailored to meet your organization’s specific needs.
Step 2: Select the Right AI Tools and Platforms
Next, select the AI tools and platforms that will form the foundation of your literature monitoring system. There are several AI-powered platforms available that specialize in different aspects of literature monitoring. Some are designed for specific industries, such as pharmacovigilance, while others are more general-purpose. When selecting a tool, consider factors such as ease of integration with your existing systems, the quality of the AI algorithms, and the level of customization available.
Step 3: Data Collection and Integration
For the AI system to function effectively, it needs access to relevant data. This involves setting up data collection processes that pull in information from the identified sources. Data integration is a critical step, as the AI system must be able to seamlessly process and analyze data from multiple sources. This may involve using APIs to connect with external databases or setting up web scraping tools to gather information from online sources.
Step 4: Train the AI System
Once the data is collected and integrated, the next step is to train the AI system. Training involves feeding the system with large amounts of data and allowing it to learn from that data. During this process, it’s essential to provide feedback to the system to help it improve its accuracy. This could involve flagging incorrect results or refining the algorithms to better understand the context of the data.
Step 5: Implement NLP and ML Algorithms
NLP and ML algorithms are at the heart of the AI-powered literature monitoring system. Implementing these algorithms involves configuring the system to recognize relevant keywords, phrases, and patterns within the data. This might include training the system to identify specific regulatory terms or industry jargon. Over time, the system should be able to automatically identify and flag content that is relevant to your compliance needs.
Step 6: Set Up Alerting and Reporting Mechanisms
After the AI system is trained and operational, set up the mechanisms for alerting and reporting. This ensures that when the system identifies relevant content, it can promptly notify the appropriate personnel. Alerts can be customized based on the level of urgency or relevance, and reports can be generated at regular intervals to provide an overview of the monitored literature.
Step 7: Continuous Monitoring and Improvement
AI systems require continuous monitoring and improvement to remain effective. Regularly review the system’s performance and make adjustments as necessary. This could involve retraining the system with new data, refining the algorithms, or expanding the data sources. Continuous improvement is essential to ensure that the AI system remains aligned with the evolving regulatory landscape.
4. Benefits of Implementing AI in Literature Monitoring
Increased Efficiency:
One of the most significant benefits of implementing AI in literature monitoring is the increased efficiency it offers. AI systems can process vast amounts of data far more quickly than human analysts, allowing compliance teams to stay on top of new developments in real-time.
Improved Accuracy:
AI systems are less prone to human error, meaning that they can often identify relevant information with greater accuracy. This reduces the risk of non-compliance due to missed information or incorrect analysis.
Cost Savings:
By automating the literature monitoring process, organizations can reduce the need for large compliance teams, leading to significant cost savings. Additionally, the increased accuracy and efficiency of AI systems can help prevent costly compliance breaches.
Scalability:
AI-powered literature monitoring systems are highly scalable, meaning they can easily adapt to increasing volumes of data. This makes them ideal for organizations that need to monitor large amounts of information across multiple sources.
Customization:
AI systems can be customized to meet the specific needs of an organization. Whether it’s focusing on particular regulatory areas, industries, or types of literature, AI can be tailored to provide the most relevant insights.
5. Challenges in Implementing AI in Literature Monitoring
Data Quality and Availability:
One of the primary challenges in implementing AI in literature monitoring is ensuring the quality and availability of data. AI systems rely on large amounts of high-quality data to function effectively. If the data is incomplete, outdated, or biased, it can negatively impact the system’s performance.
Integration with Existing Systems:
Integrating AI-powered tools with existing systems can be complex and time-consuming. Organizations need to ensure that the AI system can seamlessly connect with other compliance tools, databases, and processes.
Regulatory Compliance:
Ironically, while AI can help with regulatory compliance, implementing AI itself must also comply with various regulations. For instance, data privacy laws like GDPR may impose restrictions on how data is collected, stored, and processed. Organizations need to ensure that their AI implementation is compliant with these regulations.
Cost of Implementation:
The initial cost of implementing AI in literature monitoring can be high, particularly for small or medium-sized organizations. However, these costs are often offset by the long-term savings in efficiency and accuracy.
Human Oversight:
While AI can automate many aspects of literature monitoring, human oversight is still necessary. Compliance professionals need to review and validate the AI system’s findings to ensure accuracy and relevance. Additionally, human expertise is required to interpret complex regulatory language and apply it to specific organizational contexts.
6. Case Studies and Real-World Examples
Pharmaceutical Industry:
In the pharmaceutical industry, AI-powered literature monitoring is used to track developments in drug safety and efficacy. Companies like Pfizer and Roche have implemented AI systems to monitor scientific journals, clinical trial data, and regulatory updates. These systems help ensure that the companies remain compliant with FDA guidelines and other regulatory requirements.
Financial Services:
In the financial services sector, AI is used to monitor changes in regulations, case law, and market trends. For example, JPMorgan Chase has implemented an AI-powered compliance monitoring system that scans legal databases, news sources, and social media for relevant information. This helps the company stay compliant with SEC regulations and avoid costly fines.
Legal Industry:
Law firms are also adopting AI for literature monitoring, particularly in areas like intellectual property law and antitrust regulation. AI systems help lawyers stay informed about changes in case law, emerging legal trends, and new legislation, allowing them to provide better advice to their clients.
7. Conclusion
Implementing AI in literature monitoring for compliance is a powerful way to enhance the efficiency, accuracy, and scope of your compliance efforts. By automating the process of monitoring vast amounts of data, AI systems can help organizations stay ahead of regulatory changes and avoid costly compliance breaches. However, successful implementation requires careful planning, the right tools, and continuous monitoring to ensure that the AI system remains effective and aligned with your organization’s needs.
As the regulatory landscape continues to evolve, AI-powered literature monitoring will become increasingly essential for organizations looking to maintain compliance and mitigate risk. By following the steps outlined in this blog, you can successfully implement AI in your literature monitoring process and reap the benefits of this cutting-edge technology.
Comments