How to Implement AI in Literature Review for Compliance
- Chaitali Gaikwad
- Oct 15, 2024
- 6 min read
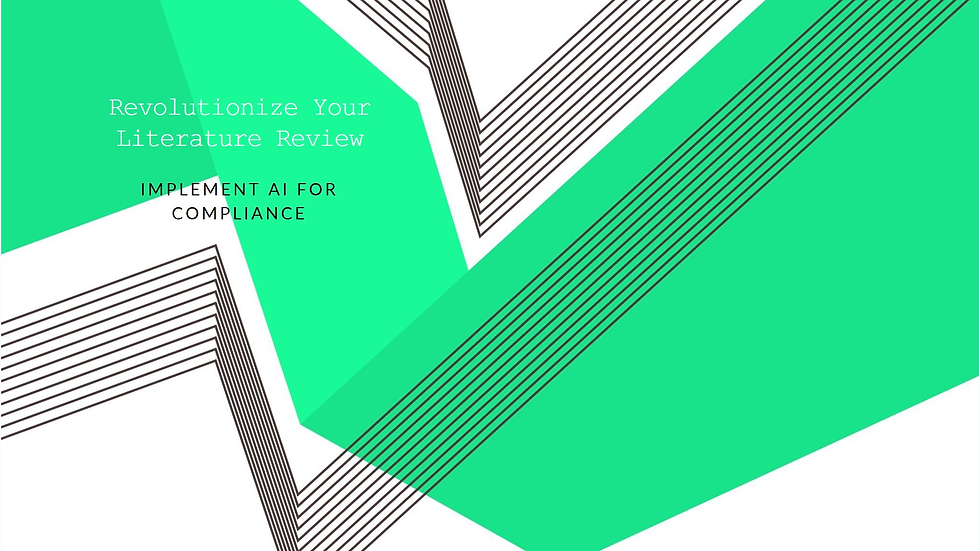
In the rapidly evolving landscape of regulatory compliance, organizations in fields such as pharmaceuticals, healthcare, and biotechnology face increasing pressure to stay updated with a deluge of scientific literature. The challenge lies in efficiently and effectively reviewing vast amounts of information to ensure compliance with regulations while identifying potential risks and advancements. Traditional literature review methods can be time-consuming, labor-intensive, and prone to human error, making the integration of Artificial Intelligence (AI) a crucial strategy for organizations aiming to enhance their compliance processes. This blog explores how to implement AI in literature review for compliance, highlighting key strategies, technologies, and best practices.
Understanding the Importance of Literature Review for Compliance
Literature reviews are systematic evaluations of published research that provide essential insights into trends, safety concerns, and the efficacy of drugs and treatments. In regulated industries, thorough literature reviews are vital for:
Risk Assessment: Identifying potential risks associated with products, treatments, or procedures.
Regulatory Compliance: Ensuring adherence to guidelines set by regulatory bodies such as the FDA, EMA, and others.
Signal Detection: Monitoring adverse events and safety signals related to drugs and medical devices.
Knowledge Management: Keeping teams informed about the latest research findings and innovations.
Given the ever-increasing volume of scientific literature, leveraging AI technologies can streamline the literature review process, improve accuracy, and enhance compliance.
Key Benefits of Implementing AI in Literature Review
Before delving into the implementation strategies, it’s important to recognize the benefits that AI brings to literature reviews for compliance:
Efficiency: AI can automate repetitive tasks, significantly reducing the time required for literature reviews.
Enhanced Accuracy: AI algorithms can minimize human error, ensuring that relevant studies and articles are not overlooked.
Comprehensive Coverage: AI can analyze vast datasets quickly, allowing organizations to stay abreast of the latest research across multiple sources.
Improved Decision-Making: By providing actionable insights from analyzed data, AI enables organizations to make informed decisions swiftly.
Steps to Implement AI in Literature Review for Compliance
1. Define Objectives and Scope
Before implementing AI, organizations must clearly define the objectives of the literature review. Consider the following questions:
What specific compliance requirements must be addressed?
Which areas of research are most relevant to your organization?
What outcomes do you aim to achieve through the literature review?
By establishing clear objectives, organizations can tailor their AI solutions to meet specific compliance needs.
2. Select the Right AI Tools
Various AI tools are available for literature review, each with unique features and capabilities. Key factors to consider when selecting AI tools include:
Natural Language Processing (NLP): NLP enables AI systems to understand and analyze human language. Look for tools that leverage NLP for automated data extraction and content summarization.
Machine Learning Algorithms: Machine learning can be used to identify patterns, classify documents, and detect relevant studies based on historical data.
Integration Capabilities: Ensure that the selected tools can integrate seamlessly with existing systems and databases to streamline workflows.
3. Data Collection and Management
A successful literature review requires access to a wide range of data sources. Implement AI-powered data collection strategies to ensure comprehensive coverage:
Automated Data Scraping: Use AI algorithms to scrape data from reputable academic databases, journals, and other relevant sources. This process can be automated to ensure real-time access to new studies.
Content Aggregation: AI tools can aggregate content from multiple sources, providing a centralized repository for literature. This simplifies the search process and enhances accessibility.
4. Utilize AI for Enhanced Search and Retrieval
Traditional keyword-based searches can be limiting, often resulting in irrelevant results. AI-powered search capabilities can improve the effectiveness of literature retrieval:
Semantic Search: Implement AI-driven semantic search capabilities that understand the context and intent behind queries. This approach yields more relevant results, even when keywords vary.
Topic Modeling: Use machine learning techniques to identify key topics and themes within large datasets. This enables organizations to focus on critical areas of research relevant to compliance.
5. Automate Abstracting and Summarization
Reading through extensive literature can be overwhelming. AI can assist in abstracting and summarizing key findings:
Automated Abstract Generation: AI algorithms can generate concise abstracts from articles, allowing researchers to quickly determine the relevance of a study.
Content Summarization: Advanced machine learning models can provide summaries of entire documents, highlighting essential points and findings. This capability saves time and ensures that critical information is not overlooked.
6. Risk Identification and Signal Detection
In compliance-driven industries, early detection of potential risks is paramount. AI can enhance risk identification through:
Adverse Event Detection: Machine learning algorithms can analyze literature for reports of adverse events related to specific products. By cross-referencing this information with existing databases, organizations can detect potential safety signals early.
Trend Analysis: AI models can analyze historical data to identify trends in adverse events over time, enabling organizations to proactively address safety concerns.
7. Reporting and Visualization
Clear and concise reporting is essential for compliance audits and decision-making. AI can facilitate this process by providing:
Data Visualization: AI-driven tools can generate intuitive visual representations of literature data, such as charts, graphs, and dashboards. These visualizations help stakeholders quickly grasp trends and insights.
Customizable Reports: AI can automate the generation of tailored reports based on user preferences. By allowing stakeholders to specify the type of information they need, organizations can ensure they receive relevant updates without sifting through irrelevant data.
8. Continuous Learning and Improvement
AI systems thrive on data, and continuous learning is essential for maintaining effectiveness. Organizations should regularly assess the performance of their AI tools:
Model Training: Regularly retrain machine learning models using updated literature data to ensure they remain accurate and relevant.
Feedback Loops: Implement feedback mechanisms to gather insights from users. This information can guide improvements and refinements to AI tools and processes.
Best Practices for Successful Implementation
To ensure successful implementation of AI in literature review for compliance, organizations should consider the following best practices:
Engage Stakeholders: Involve key stakeholders, including compliance officers, regulatory affairs professionals, and researchers, in the implementation process to ensure their needs are met.
Invest in Training: Provide training for team members on how to use AI tools effectively. This empowerment ensures that staff can leverage AI for enhanced literature monitoring and compliance.
Establish Clear Protocols: Develop clear protocols for literature review processes, including data collection, analysis, and reporting. This structure enhances consistency and compliance.
Monitor Regulatory Changes: Stay informed about changes in regulatory requirements that may impact literature review processes. AI tools should be updated accordingly to accommodate new compliance needs.
Maintain Data Security and Privacy: Ensure that data collection and management practices comply with relevant data protection regulations. Safeguarding sensitive information is critical for maintaining trust and compliance.
Case Studies of AI Implementation in Literature Review
Case Study 1: Pharmaceutical Company
A leading pharmaceutical company faced challenges in monitoring vast amounts of literature for compliance with regulatory guidelines. By implementing an AI-powered literature review tool, they automated data collection and analysis processes. The system utilized NLP and machine learning algorithms to identify relevant studies, summarize key findings, and flag potential safety signals. As a result, the company reduced literature review time by 60%, improved accuracy, and enhanced compliance with regulatory requirements.
Case Study 2: Healthcare Research Organization
A healthcare research organization struggled to keep up with the growing volume of research articles related to public health. By adopting AI technologies, they developed a comprehensive literature monitoring system that aggregated data from multiple sources. The AI tool enabled semantic search and automated abstract generation, allowing researchers to focus on critical areas of study. This implementation led to improved research output, better-informed decision-making, and enhanced compliance with funding agency requirements.
Conclusion:
The integration of AI in literature review processes for compliance represents a significant advancement in how organizations manage and analyze scientific literature. By automating data collection, enhancing search capabilities, and providing actionable insights, AI empowers organizations to stay ahead in an ever-changing regulatory landscape.
As the volume of literature continues to grow, embracing AI technologies will be essential for organizations looking to maintain compliance, improve decision-making, and ultimately ensure the safety and efficacy of their products and services. Implementing AI in literature review processes is not just a strategy for efficiency; it is a necessary step toward ensuring the highest standards of regulatory compliance in today’s complex and dynamic environment.
תגובות