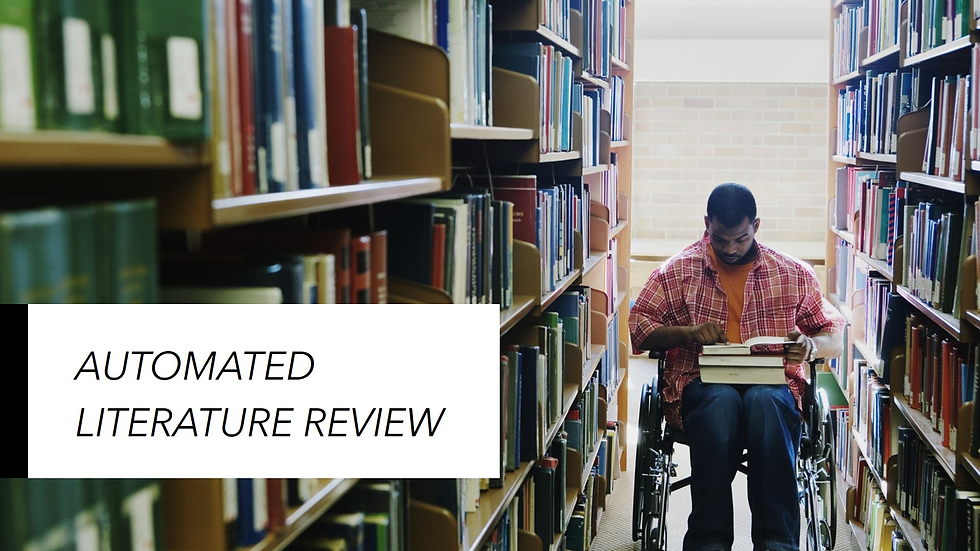
Pharmacovigilance plays a vital role in ensuring drug safety by monitoring adverse events reported in clinical studies, scientific literature, and regulatory databases. Literature reviews are a key component of pharmacovigilance, providing critical information on drug-related risks, interactions, and side effects. However, traditional manual literature reviews are time-consuming and resource-intensive. Automated literature review systems offer a more efficient alternative by leveraging artificial intelligence (AI), natural language processing (NLP), and machine learning (ML) technologies. In this blog, we’ll discuss how to implement automated literature review in pharmacovigilance to ensure effective drug safety monitoring and regulatory compliance.
1. Understanding Automated Literature Review
Automated literature review refers to the use of AI-powered systems that can search, extract, and analyze relevant content from a wide range of scientific publications, journals, and databases. These systems enable pharmacovigilance teams to streamline their processes by reducing manual effort, improving the speed of data retrieval, and enhancing the accuracy of adverse event (AE) detection.
By implementing automation, organizations can ensure continuous monitoring, improve compliance with regulatory requirements, and reduce the likelihood of human error.
2. Key Benefits of Automation in Literature Review
2.1 Faster Identification of Adverse Events
Automated systems can scan thousands of articles and reports in real-time, identifying relevant studies much faster than traditional methods. This enables early detection of potential safety signals.
2.2 Improved Accuracy and Compliance
AI-powered tools reduce the risk of human oversight and ensure consistency by applying standardized search criteria. Automation helps meet regulatory deadlines and requirements from agencies such as the FDA and EMA.
2.3 Scalability and Efficiency
As scientific literature grows exponentially, manual review processes struggle to keep up. Automated tools can efficiently manage large datasets, enabling pharmacovigilance teams to monitor multiple drugs simultaneously.
2.4 Resource Optimization
By automating repetitive tasks, organizations can free up human resources for more strategic activities, such as signal evaluation and regulatory reporting.
3. Steps to Implement Automated Literature Review in Pharmacovigilance
Implementing an automated literature review requires a strategic approach, from selecting the right tools to integrating them with existing workflows. Below is a step-by-step guide.
Step 1: Define Objectives and Scope
Start by identifying your goals and the scope of your literature review. This includes:
Target drugs and therapeutic areas: Which drugs or treatment areas require monitoring?
Regulatory requirements: Define the compliance requirements and reporting deadlines for relevant authorities.
Sources of literature: Determine which databases (e.g., PubMed, Embase) and journals will be monitored.
A clear understanding of these factors helps in selecting the appropriate tools and defining search criteria.
Step 2: Choose the Right Automation Tools
Selecting the right tools is crucial for successful implementation. Look for solutions that offer:
AI-powered search capabilities: Tools that can automatically identify keywords, synonyms, and medical terms.
NLP for text analysis: The ability to extract key insights from unstructured text.
Customizable alerts: Automated notifications for new articles matching specific criteria.
Integration with databases: Compatibility with regulatory and scientific databases like PubMed, Embase, and Medline.
Example Tools:
DistillerSR: An AI-driven platform for literature review automation.
Covidence: A tool for systematic reviews with automated search features.
Embase and PubMed integrations: Many tools offer direct access to these databases for automated searches.
Step 3: Integrate Automation Tools with Workflows
To ensure smooth implementation, integrate automated literature review tools with your existing pharmacovigilance systems. This allows seamless sharing of data across departments.
Workflow Integration: Define roles and responsibilities for teams handling automated alerts, literature assessments, and signal management.
API Connectivity: Use APIs to connect automated tools with internal databases and case management systems.
Compliance Workflow: Ensure automated processes align with internal compliance protocols and reporting structures.
Step 4: Develop Search Strategies and Filters
Create a search strategy tailored to the specific drugs or therapeutic areas you are monitoring. A robust search strategy includes:
Keywords and Synonyms: Include drug names, active ingredients, and possible adverse events.
Boolean Operators: Use AND, OR, and NOT to refine searches.
Time Filters: Set time ranges to ensure the review covers the relevant period.
Language Filters: If needed, include multi-language support to capture global literature.
Regularly refine and update search strategies based on new regulatory requirements and emerging trends.
Step 5: Validate and Train the AI Model
AI models require training to ensure they accurately identify relevant literature. This involves:
Pilot Testing: Run initial tests with a sample dataset to validate the system’s performance.
Review False Positives and Negatives: Analyze incorrectly identified articles and adjust search criteria accordingly.
Continuous Learning: Use machine learning algorithms that improve with feedback from reviewers.
Involve subject matter experts (SMEs) to validate the model’s output and provide domain-specific insights.
Step 6: Monitor and Evaluate Performance
After implementation, regularly monitor the system’s performance to ensure it meets expectations. Key metrics to track include:
Accuracy: Measure the number of relevant articles identified versus missed ones.
Processing Speed: Track how quickly the system scans and retrieves relevant literature.
Alert Effectiveness: Assess whether notifications are timely and actionable.
Compliance Metrics: Ensure the system supports timely reporting and regulatory submissions.
Use performance insights to make continuous improvements and ensure the system remains aligned with pharmacovigilance goals.
4. Overcoming Challenges in Automated Literature Review
Implementing automated literature reviews may present some challenges:
4.1 Data Quality and Integration Issues
Inconsistent data formats or incomplete integration with existing systems can limit the effectiveness of automation. Organizations must ensure data standardization and seamless integration.
4.2 False Positives and Missed Signals
AI models may generate false positives or miss relevant articles. Regular model validation and retraining are essential to mitigate these issues.
4.3 Regulatory Adaptation
Regulatory requirements may evolve, requiring adjustments in search strategies and reporting workflows. Organizations need to stay updated on regulatory changes and adapt their systems accordingly.
5. Best Practices for Successful Implementation
Here are some best practices to ensure a successful automated literature review implementation:
Collaborate Across Teams: Involve pharmacovigilance, IT, and compliance teams throughout the process.
Start with a Pilot Project: Test the system with a small set of drugs or therapeutic areas before scaling up.
Train the AI Continuously: Provide feedback loops to improve the AI model over time.
Monitor Regulatory Updates: Stay informed about regulatory changes to keep the system compliant.
Document Processes: Maintain clear documentation of search strategies, workflows, and performance metrics for audits.
6. The Future of Automated Literature Review in Pharmacovigilance
The future of automated literature review lies in enhanced AI capabilities, such as predictive analytics and real-time monitoring. Emerging trends include:
Predictive Signal Detection: AI models will predict potential risks based on trends in scientific literature.
Real-Time Monitoring: Continuous monitoring of literature sources for real-time safety updates.
AI-Augmented Human Review: Combining AI and human expertise for more accurate and efficient reviews.
Integration with Wearable Data: Future systems may incorporate data from wearable devices to track drug safety outcomes more comprehensively.
Conclusion
Implementing automated literature review in pharmacovigilance is essential for managing the growing volume of scientific data while ensuring compliance and patient safety. By following a strategic approach—defining objectives, selecting the right tools, integrating workflows, and monitoring performance—organizations can successfully transition to automated processes. While challenges such as data quality and regulatory adaptation exist, continuous improvements and AI advancements will enhance the effectiveness of automated literature reviews.
The future of pharmacovigilance lies in AI-driven solutions that enable faster, more accurate, and proactive drug safety monitoring, empowering organizations to stay ahead in a rapidly evolving healthcare landscape.
Comments