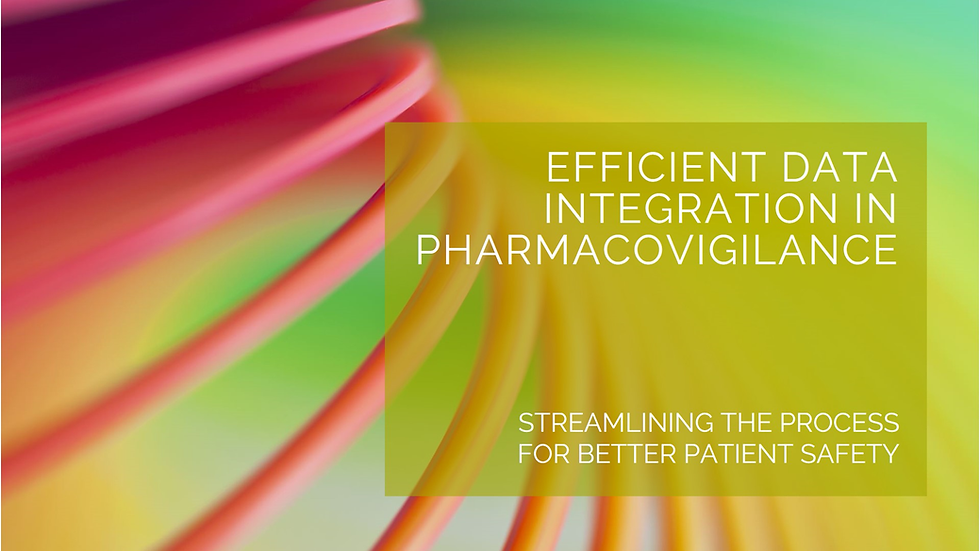
Pharmacovigilance, the science of monitoring and ensuring the safety of drugs, is a critical component of public health. As new drugs enter the market and are used in diverse populations, the need for effective pharmacovigilance systems becomes paramount. Efficient data integration is at the heart of these systems, enabling comprehensive monitoring, accurate signal detection, and timely response to adverse drug reactions (ADRs). In this blog, we will explore the strategies and best practices for implementing efficient data integration in pharmacovigilance systems, ensuring robust drug safety management.
Understanding Data Integration in Pharmacovigilance:
Data integration in pharmacovigilance refers to the process of consolidating and harmonizing data from various sources to create a unified view of drug safety information. This includes data from clinical trials, electronic health records (EHRs), patient-reported outcomes, and post-marketing surveillance. Effective integration allows for comprehensive analysis, early detection of safety signals, and informed decision-making.
The complexity of pharmacovigilance data stems from its varied sources and formats. Data integration aims to overcome these challenges by providing a coherent framework for data analysis and reporting. By integrating data efficiently, pharmacovigilance systems can enhance their ability to detect and manage potential safety issues.
Key Challenges in Data Integration for Pharmacovigilance:
Data Variety and Volume
Pharmacovigilance data comes from numerous sources, including clinical trials, EHRs, patient registries, and social media. These sources generate data in different formats—structured (e.g., databases) and unstructured (e.g., text reports). Managing this variety and volume of data can be challenging, particularly when trying to achieve a unified view.
Data Quality and Consistency
The quality of pharmacovigilance data can vary significantly. Inconsistent data formats, incomplete records, and discrepancies between sources can hinder effective integration. Ensuring data quality and consistency is crucial for reliable analysis and accurate signal detection.
Regulatory Compliance
Pharmacovigilance systems must comply with regulatory requirements and standards, such as those set by the FDA, EMA, and ICH. These regulations dictate how data should be collected, stored, and reported. Implementing data integration solutions that adhere to these requirements is essential for maintaining compliance.
Interoperability
Different data sources and systems may use incompatible data standards and protocols. Achieving interoperability between these systems is a significant challenge in data integration. Standardization of data formats and communication protocols is necessary to facilitate seamless integration.
Strategies for Efficient Data Integration in Pharmacovigilance
Develop a Data Integration Strategy
A well-defined data integration strategy is the foundation for efficient integration. This strategy should outline the objectives, scope, and approach for integrating data from various sources. Key components of the strategy include:
Data Sources Identification: Identify all relevant data sources, including clinical trial databases, EHRs, patient registries, and social media.
Data Integration Goals: Define the goals of integration, such as improving signal detection, enhancing data quality, and ensuring regulatory compliance.
Integration Framework: Establish a framework for integration, including data standards, processes, and technologies.
Implement Data Standardization
Data standardization involves converting data into a consistent format, making it easier to integrate and analyze. Key steps in data standardization include:
Adopt Common Data Standards: Utilize standardized data formats and terminologies, such as CDISC for clinical trials and HL7 for EHRs.
Develop Data Mapping Protocols: Create mapping protocols to convert data from different formats into a unified structure.
Use Data Dictionaries: Implement data dictionaries to define and standardize data elements, ensuring consistency across sources.
Leverage Data Integration Technologies
Advanced technologies can facilitate efficient data integration. Key technologies include:
Data Warehousing: Data warehouses consolidate data from multiple sources into a central repository. This centralized approach allows for easier access, management, and analysis of integrated data.
ETL (Extract, Transform, Load) Tools: ETL tools extract data from various sources, transform it into a standardized format, and load it into a target system. These tools automate data integration processes and improve efficiency.
Data Lakes: Data lakes store large volumes of raw data in its native format. This approach provides flexibility in data integration and allows for the integration of structured and unstructured data.
Ensure Data Quality and Validation
High-quality data is essential for accurate analysis and decision-making. To ensure data quality:
Implement Data Validation Checks: Use automated validation checks to identify and correct errors, inconsistencies, and missing data.
Conduct Regular Data Audits: Perform regular audits to assess data quality and address any issues.
Use Data Cleansing Techniques: Apply data cleansing techniques to remove duplicates, correct errors, and standardize data.
Enhance Interoperability
Achieving interoperability between different systems and data sources is crucial for efficient integration. Strategies to enhance interoperability include:
Adopt Industry Standards: Use industry standards for data exchange and communication, such as XML, JSON, and FHIR.
Implement APIs (Application Programming Interfaces): APIs facilitate data exchange between systems by providing standardized interfaces for communication.
Collaborate with Stakeholders: Work with stakeholders, including regulatory agencies and technology vendors, to address interoperability challenges and promote standardization.
Utilize Advanced Analytics and AI
Advanced analytics and AI can enhance data integration by providing insights and automating processes:
Machine Learning: Use machine learning algorithms to analyze large volumes of data and identify patterns or anomalies. Machine learning can improve signal detection and prediction of potential safety issues.
Natural Language Processing (NLP): NLP can analyze unstructured data, such as free-text reports, to extract valuable information and integrate it with structured data.
Predictive Analytics: Employ predictive analytics to forecast potential safety concerns based on historical data and trends.
Ensure Regulatory Compliance
Compliance with regulatory requirements is essential for pharmacovigilance systems. To ensure compliance:
Understand Regulatory Requirements: Stay informed about relevant regulations and guidelines, such as those from the FDA, EMA, and ICH.
Implement Compliance Frameworks: Develop and implement frameworks to ensure that data integration processes adhere to regulatory standards.
Conduct Compliance Reviews: Regularly review and update integration practices to ensure ongoing compliance with evolving regulations.
Facilitate User Training and Adoption
Effective data integration requires users to understand and adopt new systems and processes. To facilitate adoption:
Provide Training: Offer training programs for users to familiarize them with new integration tools and processes.
Develop User Manuals: Create user manuals and documentation to guide users through the integration process.
Support Change Management: Implement change management strategies to address any resistance to new systems and ensure smooth transitions.
Real-World Examples of Efficient Data Integration:
FDA Sentinel Initiative
The FDA Sentinel Initiative uses a distributed data network to monitor drug safety. The system integrates data from various sources, including claims databases and EHRs, to provide a comprehensive view of drug safety. By utilizing advanced analytics and data standardization, the initiative enhances signal detection and improves the overall effectiveness of pharmacovigilance.
European Medicines Agency (EMA) EHR Data Integration
The EMA integrates EHR data from multiple sources to enhance pharmacovigilance efforts. By adopting standardized data formats and leveraging data warehousing technologies, the EMA provides a unified view of drug safety information. This integration supports timely detection of safety signals and facilitates informed decision-making.
IBM Watson for Drug Safety
IBM Watson uses AI and machine learning to analyze large volumes of pharmacovigilance data. The system integrates data from clinical trials, EHRs, and patient reports to identify potential safety issues. By employing advanced analytics and natural language processing, Watson enhances signal detection and supports proactive safety management.
Conclusion
Implementing efficient data integration in pharmacovigilance systems is crucial for ensuring drug safety and protecting public health. By developing a comprehensive data integration strategy, standardizing data formats, leveraging advanced technologies, and ensuring regulatory compliance, organizations can enhance their pharmacovigilance efforts. Addressing challenges such as data variety, quality, and interoperability is essential for achieving a unified view of drug safety information. As technology continues to advance, adopting innovative solutions and best practices will further enhance the efficiency and effectiveness of data integration in pharmacovigilance systems, ultimately leading to better safety outcomes for patients.
コメント