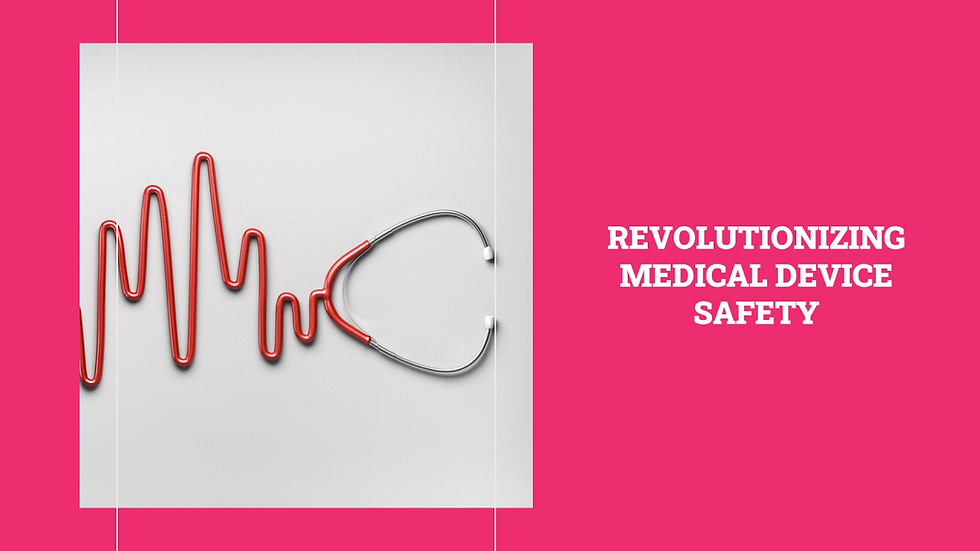
Machine learning (ML) has revolutionized various industries, and healthcare is no exception. In recent years, ML algorithms have been increasingly used to analyze large datasets and extract valuable insights that can inform decision-making and improve patient outcomes. One area where ML holds significant promise is in medical device safety databases. By leveraging ML techniques, healthcare organizations and regulatory authorities can enhance surveillance, identify emerging trends, and mitigate risks associated with medical devices. In this blog post, we'll explore the benefits of implementing ML in medical device safety databases and provide insights into how healthcare stakeholders can harness this technology effectively.
Understanding Medical Device Safety Databases:
Medical device safety databases, such as the FDA's MAUDE (Manufacturer and User Facility Device Experience), serve as repositories for reports of adverse events, malfunctions, and other incidents related to medical devices. These databases play a critical role in post-market surveillance, allowing regulatory authorities, healthcare professionals, and manufacturers to monitor device safety and performance after they are placed on the market. However, analyzing the vast amounts of data collected in these databases manually can be time-consuming and resource-intensive.
The Role of Machine Learning:
Machine learning algorithms have the potential to automate and enhance the analysis of medical device safety data in several ways:
Pattern Recognition: ML algorithms can identify patterns, trends, and anomalies in medical device safety data that may not be immediately apparent to human analysts. By analyzing large datasets, ML models can uncover associations between specific devices, adverse events, patient demographics, and other factors.
Predictive Analytics: ML techniques, such as predictive modeling, can forecast future outcomes based on historical data. By training models on past incident reports and device performance data, healthcare organizations can anticipate potential safety issues, prioritize resources, and proactively address emerging risks.
Natural Language Processing (NLP): NLP algorithms can analyze unstructured text data, such as free-text descriptions of adverse events, to extract relevant information and insights. By processing narrative reports more efficiently, NLP can improve the accuracy and completeness of data analysis in medical device safety databases.
Anomaly Detection: ML algorithms can flag unusual or unexpected patterns in medical device safety data, indicating potential safety concerns or areas warranting further investigation. By automatically detecting anomalies, healthcare organizations can identify and address device malfunctions or adverse events more promptly.
Benefits of Implementing ML in Medical Device Safety Databases:
Improved Efficiency: ML algorithms can process and analyze large volumes of data much faster than manual methods, allowing healthcare organizations to identify safety issues and trends more quickly.
Enhanced Accuracy: ML models can reduce human error and bias in data analysis by systematically analyzing large datasets and identifying patterns that may not be immediately apparent to human analysts.
Early Detection of Safety Issues: By leveraging predictive analytics and anomaly detection, ML algorithms can identify potential safety concerns before they escalate, enabling proactive intervention and risk mitigation.
Data-driven Decision-Making: ML-generated insights can inform evidence-based decision-making by healthcare organizations, regulatory authorities, and manufacturers, leading to more targeted interventions and resource allocation.
Continuous Learning and Improvement: ML models can continuously learn from new data and adapt to evolving trends and patterns in medical device safety data, ensuring that surveillance systems remain effective over time.
Challenges and Considerations:
While ML holds great promise for enhancing medical device safety surveillance, several challenges and considerations must be addressed:
Data Quality: ML models rely on high-quality, accurate, and representative data for training and analysis. Ensuring data integrity and completeness is crucial for the success of ML implementations in medical device safety databases.
Interpretability: ML models, particularly complex deep learning algorithms, can be challenging to interpret and explain. Healthcare stakeholders must prioritize transparency and interpretability to ensure that ML-generated insights are understandable and actionable.
Regulatory Compliance: Healthcare organizations must ensure that ML implementations in medical device safety databases comply with regulatory requirements, such as data privacy and security regulations and FDA guidelines for software as a medical device .
Resource Constraints: Implementing and maintaining ML systems requires significant resources, including expertise in data science, computational infrastructure, and ongoing training and validation of ML models.
Case Studies and Success Stories:
Several healthcare organizations and regulatory authorities have already begun leveraging ML in medical device safety surveillance with promising results:
FDA's Sentinel System: The FDA's Sentinel System, a national electronic surveillance system, utilizes ML algorithms to monitor the safety of medical products, including medical devices. By analyzing electronic health records and administrative claims data, the Sentinel System can rapidly detect and assess safety signals associated with medical devices.
Best Practices for Implementing ML in Medical Device Safety Databases:
Collaboration and Partnerships: Healthcare organizations should collaborate with data scientists, researchers, and technology partners to develop and implement ML solutions tailored to the specific needs and objectives of medical device safety surveillance.
Data Governance and Quality Assurance: Establishing robust data governance processes and quality assurance mechanisms is essential for ensuring the integrity, reliability, and accuracy of data used for training ML models and generating insights.
Transparency and Accountability: Healthcare stakeholders must prioritize transparency and accountability in ML implementations, ensuring that ML-generated insights are interpretable, explainable, and actionable.
Continuous Monitoring and Evaluation: ML models should be continuously monitored and evaluated to assess their performance, identify potential biases or errors, and ensure that they remain effective and reliable over time.
Conclusion:
Implementing machine learning in medical device safety databases offers significant opportunities to enhance surveillance, analysis, and decision-making in healthcare. By leveraging ML algorithms for pattern recognition, predictive analytics, NLP, and anomaly detection, healthcare organizations and regulatory authorities can identify safety issues, predict emerging trends, and mitigate risks associated with medical devices more effectively. However, addressing challenges related to data quality, interpretability, regulatory compliance, and resource constraints is essential for the successful implementation of ML in medical device safety surveillance. By adopting best practices and lessons learned from successful case studies, healthcare stakeholders can harness the power of ML to improve patient safety and advance medical device regulation and oversight.
Comentários