How to Implement Machine Learning in Vaccine Safety Databases
- Chaitali Gaikwad
- Jun 14, 2024
- 5 min read
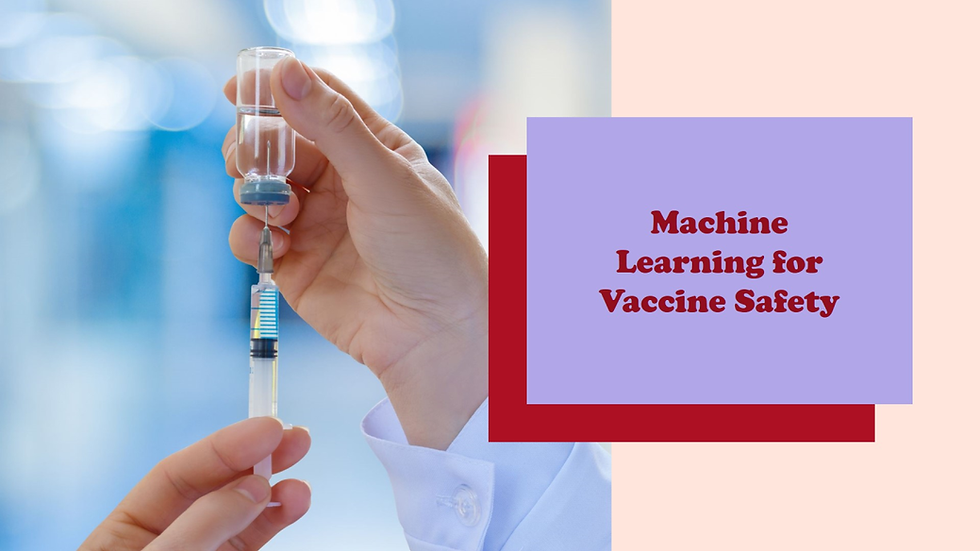
In recent years, the integration of machine learning (ML) in healthcare has revolutionized various aspects of medical data analysis and decision-making. One critical area where ML shows tremendous potential is in vaccine safety databases. These databases play a pivotal role in monitoring adverse events following immunization (AEFI) and ensuring the safety and efficacy of vaccines. This blog explores the implementation of machine learning in vaccine safety databases, detailing its applications, benefits, challenges, and future implications.
1. Introduction to Machine Learning in Healthcare:
Machine learning involves algorithms and statistical models that enable computers to learn from and make predictions or decisions based on data. In healthcare, ML algorithms are used to analyze vast amounts of medical data, identify patterns, and make predictions that can assist healthcare professionals in diagnosis, treatment planning, and public health initiatives.
2. Importance of Vaccine Safety Databases:
Vaccine safety databases are central to monitoring and assessing the safety profiles of vaccines post-licensure. They collect and analyze data on adverse events reported following immunization, providing critical insights into potential safety concerns and informing regulatory decisions and public health policies.
Key Functions:
Data Collection: Gathering adverse event reports from healthcare providers, patients, and other sources.
Surveillance: Monitoring and analyzing adverse event data to detect trends, patterns, and safety signals.
Reporting: Providing timely and accurate information to regulatory agencies, healthcare professionals, and the public.
3. Applications of Machine Learning in Vaccine Safety Databases:
Machine learning offers several applications that can enhance the functionality and effectiveness of vaccine safety databases:
a. Predictive Analytics:
Predicting Adverse Events: ML algorithms can analyze historical data to predict the likelihood of adverse events associated with specific vaccines or patient demographics.
Risk Assessment: Assessing the risk of adverse events based on factors such as age, gender, medical history, and vaccine type.
b. Pattern Recognition:
Identifying Safety Signals: ML algorithms can detect patterns or anomalies in adverse event data that may indicate potential safety concerns or emerging risks.
Cluster Analysis: Grouping similar adverse events to identify clusters that may suggest common underlying causes.
c. Natural Language Processing (NLP):
Extracting Insights from Textual Data: NLP techniques can extract valuable information from unstructured data sources, such as medical records or patient narratives, to enhance adverse event reporting and analysis.
4. Implementing Machine Learning in Vaccine Safety Databases
Implementing machine learning in vaccine safety databases requires careful planning, infrastructure development, and collaboration among healthcare professionals, data scientists, and IT experts. Here’s a step-by-step approach:
a. Define Objectives and Scope:
Identify Goals: Determine specific objectives for implementing ML, such as improving predictive capabilities or enhancing data analysis efficiency.
Scope: Define the scope of the ML implementation, including the types of data to be analyzed, the ML techniques to be used, and the intended outcomes.
b. Data Collection and Integration:
Data Sources: Identify and integrate relevant data sources, including adverse event reports, electronic health records (EHRs), vaccination registries, and demographic data.
Data Quality: Ensure data quality and consistency through data cleaning, normalization, and standardization processes.
c. Algorithm Selection and Development:
Choose ML Algorithms: Select appropriate ML algorithms based on the objectives and data characteristics. Common algorithms include decision trees, random forests, support vector machines (SVM), and neural networks.
Model Development: Develop and train ML models using historical data to predict adverse events, detect patterns, or classify safety signals.
d. Integration with Existing Systems:
Database Integration: Integrate ML models with existing vaccine safety databases and surveillance systems.
Real-Time Monitoring: Implement mechanisms for real-time monitoring and analysis of adverse event data to enable timely detection and response.
e. Validation and Evaluation:
Validation: Validate ML models using independent datasets or cross-validation techniques to assess their accuracy, sensitivity, specificity, and performance metrics.
Continuous Improvement: Continuously evaluate and refine ML models based on feedback, new data, and emerging trends in vaccine safety.
5. Benefits of Machine Learning in Vaccine Safety Databases:
Implementing ML in vaccine safety databases offers several benefits that contribute to enhanced surveillance, decision-making, and public health outcomes:
a. Early Detection of Safety Signals:
ML algorithms can identify potential safety concerns or adverse events earlier than traditional methods, allowing for timely interventions and risk mitigation strategies.
b. Improved Predictive Capabilities:
Predictive analytics enable healthcare providers and public health officials to anticipate adverse events and tailor vaccination strategies based on risk profiles.
c. Enhanced Data Analysis Efficiency:
ML automates data analysis processes, reducing the time and resources required for manual review and enabling more comprehensive surveillance across large datasets.
d. Personalized Medicine Approaches:
ML models can support personalized medicine approaches by identifying patient-specific risk factors or adverse event profiles, facilitating targeted interventions and healthcare delivery.
6. Challenges and Considerations:
While ML offers significant advantages, its implementation in vaccine safety databases also presents challenges and considerations:
a. Data Quality and Bias:
Ensuring the quality, completeness, and representativeness of data is crucial for the accuracy and reliability of ML models. Addressing biases in data sources and algorithms is essential to prevent skewed outcomes.
b. Regulatory and Ethical Considerations:
Compliance with regulatory requirements, data protection laws, and ethical standards is paramount when handling sensitive health data in vaccine safety surveillance.
c. Interpretability and Transparency:
ML models may lack interpretability, making it challenging to understand the rationale behind predictions or decisions. Ensuring transparency in model development and outcomes is critical for trust and accountability.
7. Future Directions and Innovations:
The future of ML in vaccine safety databases holds promise for continued advancements and innovations:
a. Advanced AI Techniques:
Integration of advanced AI techniques, such as deep learning and reinforcement learning, to enhance predictive capabilities and pattern recognition in adverse event data.
b. Real-Time Surveillance Systems:
Development of real-time surveillance systems that use ML to monitor vaccine safety continuously and detect safety signals as they emerge.
c. Integration with Emerging Technologies:
Integration with blockchain for secure data sharing, IoT for real-time data collection, and natural language generation (NLG) for automated reporting and communication.
8. Ethical and Societal Impact:
ML in vaccine safety databases has profound ethical and societal implications, influencing public trust in vaccines, healthcare decision-making, and equitable access to immunization:
a. Public Trust and Transparency:
Maintaining transparency in ML algorithms and outcomes is essential to foster public trust and acceptance of vaccine safety surveillance efforts.
b. Equity in Vaccine Access:
Ensuring that ML-driven insights and interventions benefit all populations equitably, addressing disparities in vaccine coverage and safety monitoring.
9. Case Studies and Success Stories:
Explore case studies and success stories where ML has been successfully implemented in vaccine safety databases, showcasing tangible benefits and lessons learned from real-world applications.
10. Conclusion:
Implementing machine learning in vaccine safety databases represents a transformative opportunity to enhance surveillance, prediction, and decision-making in public health. By leveraging ML algorithms to analyze vast datasets, detect safety signals early, and improve the efficiency of adverse event reporting, healthcare systems can strengthen vaccine safety monitoring and ensure the continued success of immunization programs worldwide.
As technology evolves and healthcare stakeholders embrace innovation, the integration of ML in vaccine safety databases will play a pivotal role in shaping the future of public health, ensuring safer vaccines and better health outcomes for global populations.
Comments