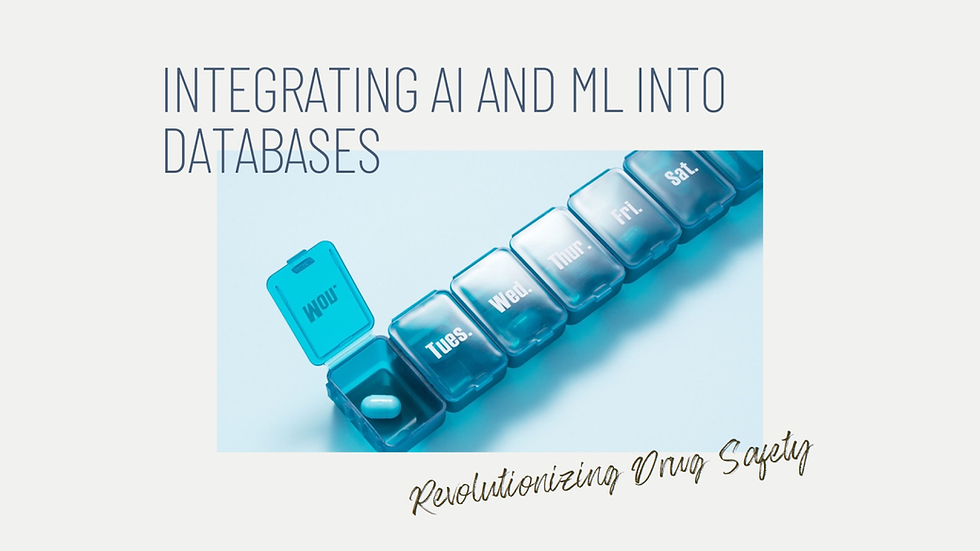
Drug safety is a critical aspect of pharmaceutical development and healthcare. Ensuring that medications are safe and effective involves rigorous monitoring and analysis of data collected from clinical trials, post-market surveillance, and real-world usage. Traditional methods of managing and analyzing drug safety data often fall short in handling the sheer volume and complexity of information. This is where Artificial Intelligence (AI) and Machine Learning (ML) come into play. By integrating AI and ML into drug safety databases, we can enhance the efficiency, accuracy, and depth of drug safety assessments. In this blog, we will explore the steps and considerations involved in this integration process.
The Importance of Drug Safety Databases:
Drug safety databases are repositories of information related to adverse drug reactions (ADRs), side effects, and other safety-related data. These databases are vital for:
Identifying ADRs: Detecting and recording adverse reactions to medications.
Regulatory Compliance: Ensuring that pharmaceutical companies comply with regulatory requirements.
Risk Management: Assessing and mitigating risks associated with drug usage.
Research and Development: Informing the development of safer drugs.
Given the importance of these databases, integrating AI and ML can significantly enhance their functionality and effectiveness.
Benefits of AI and ML in Drug Safety:
Enhanced Data Analysis
AI and ML algorithms can process vast amounts of data quickly and accurately. This capability is crucial for identifying patterns, trends, and anomalies that might be missed by traditional methods.
Improved ADR Detection
Machine learning models can be trained to recognize subtle signals in data that indicate potential ADRs. This leads to earlier detection and intervention, improving patient safety.
Automation of Routine Tasks
AI can automate routine data entry, cleaning, and analysis tasks, freeing up human resources for more complex and strategic activities.
Predictive Analytics
Predictive models can forecast potential drug safety issues before they become widespread, allowing for proactive measures.
Integration of Diverse Data Sources
AI can integrate and analyze data from various sources, such as electronic health records (EHRs), social media, and scientific literature, providing a more comprehensive view of drug safety.
Steps to Integrate AI and ML into Drug Safety Databases:
1. Define Objectives and Requirements
Before integrating AI and ML, it is essential to define clear objectives. What specific problems are you trying to solve? What are the expected outcomes? Defining these goals will guide the selection of appropriate AI and ML tools and methodologies.
2. Data Collection and Preparation
AI and ML algorithms require high-quality data to function effectively. This involves:
Data Collection: Gathering data from various sources such as clinical trials, EHRs, and pharmacovigilance reports.
Data Cleaning: Removing duplicates, correcting errors, and handling missing values.
Data Transformation: Converting data into formats suitable for analysis, such as normalizing and scaling numerical values.
3. Data Integration
Integrate data from different sources into a unified database. This may involve using ETL (Extract, Transform, Load) tools and data warehousing solutions. Ensure that the integrated data maintains its integrity and consistency.
4. Selection of AI and ML Models
Choose appropriate AI and ML models based on the defined objectives. Common models used in drug safety include:
Supervised Learning: For tasks such as classification (e.g., identifying ADRs) and regression (e.g., predicting the severity of reactions).
Unsupervised Learning: For clustering similar adverse events and identifying unknown patterns.
Natural Language Processing (NLP): For analyzing unstructured data, such as clinical notes and social media posts.
5. Model Training and Validation
Train the selected models using historical data. This involves:
Feature Selection: Identifying the most relevant variables for the model.
Training: Feeding the model with training data and adjusting parameters to minimize error.
Validation: Testing the model on a separate dataset to evaluate its performance and generalizability.
6. Deployment
Deploy the trained models into the drug safety database system. This can be done on-premises or using cloud-based solutions. Ensure that the deployment environment is scalable and secure.
7. Monitoring and Maintenance
Continuous monitoring is essential to ensure that the models perform as expected in real-world scenarios. Regularly update the models with new data to maintain their accuracy and relevance. Implement feedback loops to refine the models based on user input and new findings.
Key Considerations and Challenges:
Data Privacy and Security
Drug safety data often contains sensitive patient information. Ensuring data privacy and security is paramount. This involves complying with regulations such as GDPR and HIPAA, implementing robust encryption methods, and establishing strict access controls.
Model Interpretability
Healthcare professionals need to understand the rationale behind AI and ML predictions. Ensuring model interpretability and transparency is crucial for gaining trust and facilitating informed decision-making.
Regulatory Compliance
Regulatory bodies such as the FDA and EMA have specific guidelines for the use of AI and ML in drug safety. Ensuring compliance with these regulations is critical to avoid legal issues and ensure patient safety.
Integration with Existing Systems
Integrating AI and ML solutions with existing drug safety systems and workflows can be challenging. This requires careful planning, testing, and collaboration with IT departments and other stakeholders.
Scalability
As the volume of drug safety data grows, ensuring that AI and ML solutions can scale effectively is essential. This involves choosing scalable architectures and technologies that can handle increasing data loads and processing demands.
Case Studies
Case Study 1: NLP for ADR Detection
A pharmaceutical company integrated NLP algorithms into their drug safety database to analyze unstructured data from clinical notes and social media. The NLP models were able to identify previously unreported ADRs and provided early warnings for potential safety issues, leading to more timely interventions.
Case Study 2: Predictive Analytics for Risk Management
Another company implemented predictive analytics models to forecast the likelihood of severe ADRs based on patient demographics, medical history, and drug interactions. This allowed for proactive risk management and personalized patient care, reducing the incidence of severe adverse events.
Case Study 3: Automation of Pharmacovigilance Processes
A large-scale deployment of AI was used to automate routine pharmacovigilance tasks such as data entry, triage, and signal detection. This significantly reduced the workload on human analysts and improved the efficiency and accuracy of ADR reporting.
Future Trends:
The integration of AI and ML into drug safety databases is still evolving. Future trends may include:
Advanced Predictive Models
The development of more sophisticated predictive models that can consider a wider range of variables and provide more accurate risk assessments.
Real-Time Monitoring
AI-driven real-time monitoring systems that can provide immediate alerts for potential safety issues, enabling faster responses.
Integration with Genomic Data
Combining drug safety data with genomic information to identify genetic factors that influence drug safety and efficacy, leading to personalized medicine approaches.
AI-Driven Drug Development
Using AI to simulate and predict drug safety profiles during the early stages of drug development, reducing the need for extensive animal testing and early human trials.
Conclusion:
Integrating AI and ML into drug safety databases holds immense potential for enhancing drug safety monitoring, improving patient outcomes, and streamlining regulatory compliance. By following a structured approach to data collection, model selection, and deployment, pharmaceutical companies and healthcare organizations can leverage these technologies to transform their drug safety processes. While challenges remain, the benefits of AI and ML integration far outweigh the risks, paving the way for a safer and more efficient pharmaceutical landscape.
Comments