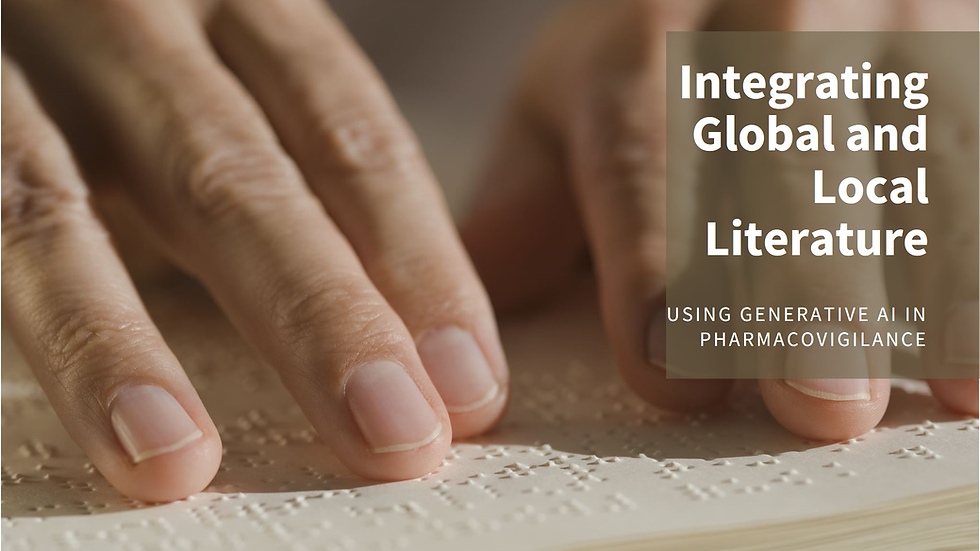
Pharmacovigilance (PV) is the science and activities related to detecting, assessing, understanding, and preventing adverse effects or other drug-related problems. Literature monitoring is a critical component of pharmacovigilance, providing valuable data on adverse drug reactions (ADRs), new safety signals, and drug efficacy. However, integrating global and local literature—a task involving diverse languages, formats, and regulatory requirements—remains a significant challenge. Generative AI has emerged as a game-changing technology in addressing these challenges, transforming the efficiency, accuracy, and scalability of literature integration in PV.
This blog delves into how generative AI can revolutionize the integration of global and local literature for pharmacovigilance, emphasizing its capabilities, benefits, and future potential.
Challenges in Integrating Global and Local Literature in Pharmacovigilance
Before understanding the role of generative AI, it is crucial to explore the key hurdles faced in integrating global and local literature:
Diversity in Data Sources:
Global literature comes from well-structured databases like PubMed, Embase, and WHO databases, while local literature is often scattered across regional journals, conference proceedings, or local newspapers.
This makes it challenging to standardize and collate data effectively.
Language Barriers:
Pharmacovigilance requires monitoring literature in multiple languages to meet regulatory requirements in different regions. Translating medical and scientific content accurately is a major obstacle.
Volume of Data:
With thousands of publications generated daily, identifying relevant articles for PV is labor-intensive and prone to human error.
Regulatory Variations:
Different countries and regions have unique pharmacovigilance reporting requirements. Integrating literature to meet these diverse requirements adds to the complexity.
Time Constraints:
Quick identification of safety signals is critical to prevent harm to patients. Manual processes for screening and analysis are time-consuming.
Generative AI, with its ability to create, synthesize, and analyze text data, provides innovative solutions to overcome these challenges.
How Generative AI Supports Literature Integration in Pharmacovigilance
Generative AI technologies, such as OpenAI's GPT models or similar large language models (LLMs), have evolved significantly in their ability to process, understand, and generate human-like text. Here's how they contribute to integrating global and local literature in PV:
1. Automated Literature Screening and Relevance Detection
Generative AI models can rapidly sift through vast quantities of global and local literature to identify articles relevant to pharmacovigilance. Using natural language processing (NLP), these models:
Extract keywords related to ADRs, product names, and therapeutic areas.
Analyze abstract and full-text articles to determine relevance.
Highlight safety signals or unusual patterns that warrant further investigation.
This automation reduces the manual burden on pharmacovigilance teams and ensures no critical information is overlooked.
2. Multilingual Translation and Analysis
A significant advantage of generative AI lies in its ability to handle multilingual content. Pharmacovigilance requires analyzing literature from diverse geographies, often written in local languages. Generative AI can:
Translate abstracts and full-text articles into the desired language while preserving context and technical accuracy.
Summarize key findings in a language understandable to pharmacovigilance experts.
For example, AI tools can integrate findings from Chinese, Russian, or Spanish journals into a centralized database, enabling seamless global-local literature integration.
3. Intelligent Data Extraction
AI models can be trained to identify and extract critical information from literature, including:
Drug names, doses, and formulations.
Adverse events, severity, and outcomes.
Patient demographics and comorbidities.
Reporter information (e.g., healthcare professionals or patients).
This capability eliminates the need for manual data entry and ensures consistency and accuracy in reporting.
4. Signal Detection and Analysis
By analyzing trends in global and local literature, generative AI can identify emerging safety signals. For example:
Global Context: Identifying ADRs reported in multiple international studies.
Local Insights: Highlighting region-specific safety concerns that might not appear in global databases.
The AI models can also correlate signals from literature with other data sources, such as Individual Case Safety Reports (ICSRs), to provide a comprehensive safety assessment.
5. Regulatory Compliance
Generative AI can adapt literature monitoring outputs to meet the unique requirements of various regulatory authorities, such as:
EMA’s EudraVigilance, FDA’s FAERS, and PMDA in Japan.
National pharmacovigilance systems in emerging markets.
AI models ensure that extracted data is structured and reported in formats compliant with these regulations.
6. Continuous Learning and Adaptation
Generative AI models are highly adaptable. They can:
Learn from user feedback to improve accuracy over time.
Incorporate new keywords, therapeutic areas, or reporting guidelines as regulations evolve.
Stay updated with the latest scientific developments and integrate newly published data seamlessly.
Benefits of Using Generative AI in Literature Integration
1. Enhanced Efficiency
AI-driven automation significantly reduces the time required for screening, translating, and analyzing literature. Teams can focus on higher-value tasks like interpreting safety signals and making regulatory submissions.
2. Improved Accuracy
Generative AI eliminates human errors associated with manual processes, such as missed articles, incomplete data extraction, or misinterpretation due to language barriers.
3. Cost-Effectiveness
Automating routine tasks reduces the operational costs of literature monitoring while improving output quality.
4. Scalability
Generative AI systems can handle increasing volumes of global and local literature without compromising performance, making them ideal for growing pharmacovigilance needs.
5. Comprehensive Insights
By integrating data from global and local sources, AI ensures that pharmacovigilance teams have a holistic view of drug safety, including regional nuances.
6. Regulatory Alignment
Generative AI simplifies compliance with diverse regulatory frameworks, reducing the risk of non-compliance penalties.
Future Outlook: Generative AI and Literature Monitoring in Pharmacovigilance
The future of generative AI in literature integration is promising, with advancements likely to include:
Enhanced Contextual Understanding: Future AI models will better understand the context of adverse event reports, enabling nuanced insights.
Integration with Real-World Evidence: Combining literature data with real-world evidence, such as electronic health records (EHRs) and social media monitoring, will provide deeper safety insights.
Adaptive Compliance: AI systems will evolve to preemptively align with new regulations, ensuring seamless compliance.
Human-AI Collaboration: Generative AI will act as an intelligent assistant, augmenting human expertise rather than replacing it.
How Crypta Helps with Literature Integration in Pharmacovigilance
Crypta, a Generative AI-powered Literature Review and Monitoring Platform, embodies the advancements discussed above. It provides comprehensive support for integrating global and local literature through:
Automated Screening and Relevance Detection: Crypta identifies and highlights relevant articles using advanced AI algorithms.
Multilingual Support: It translates and summarizes literature in multiple languages, ensuring accurate insights across regions.
Regulatory Compliance: Crypta’s outputs are aligned with global pharmacovigilance regulatory requirements.
Customizable Workflows: Tailored workflows cater to diverse industry needs and therapeutic areas.
Crypta empowers pharmacovigilance teams to stay ahead of the challenges in literature monitoring, ensuring patient safety and regulatory compliance while streamlining operations. By adopting Crypta, organizations can unlock the full potential of generative AI in their pharmacovigilance efforts.
#Pharmacovigilance #DrugSafety #LifeSciences #PharmaInnovation #PatientSafety #HealthTech #Automation #ArtificialIntelligence #RegTech #DigitalTransformation #Salvus
#Crypta #Datacreds #SignalDetection #LiteratureReview #ICSRProcessing #RegulatoryCompliance #SafetyMonitoring #FutureOfPharma #InnovativeSolutions
留言