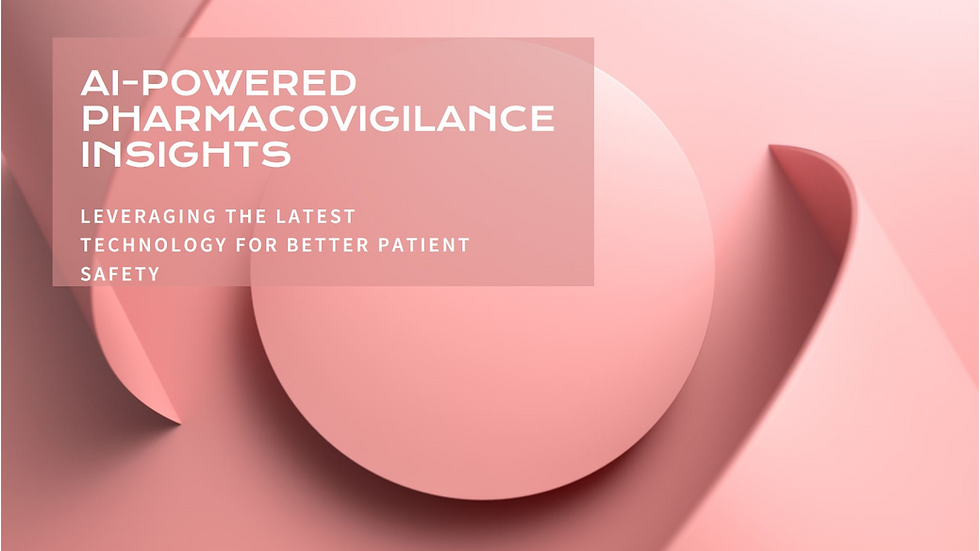
Pharmacovigilance, the science and activities relating to the detection, assessment, understanding, and prevention of adverse effects or any other drug-related problems, is crucial for ensuring the safety and efficacy of pharmaceutical products. With the exponential growth of data in the healthcare sector, traditional pharmacovigilance methods are often inadequate to manage and analyze vast amounts of information efficiently. This is where Artificial Intelligence (AI) steps in, offering advanced solutions to enhance pharmacovigilance practices. In this blog, we will explore how AI can be leveraged to gain advanced insights in pharmacovigilance, thereby improving drug safety and patient outcomes.
Understanding Pharmacovigilance and Its Challenges:
Pharmacovigilance involves several key activities, including:
Adverse Event Reporting: Collecting reports of adverse events (AEs) from healthcare providers, patients, and pharmaceutical companies.
Signal Detection: Identifying potential safety signals from the reported data.
Risk Management: Developing strategies to mitigate identified risks.
Regulatory Compliance: Ensuring adherence to regulatory requirements and guidelines.
The primary challenges in pharmacovigilance include:
Data Volume: The sheer volume of data from clinical trials, post-marketing surveillance, electronic health records (EHRs), and social media is overwhelming.
Data Variety: Data comes in various formats, including structured data, unstructured text, images, and genomic data.
Timeliness: Quickly identifying and responding to safety signals is critical to protect patients.
Accuracy: Ensuring the accuracy and reliability of data is essential for making informed decisions.
Resource Constraints: Manual review and analysis of data require significant resources and time.
AI technologies, such as machine learning (ML), natural language processing (NLP), and deep learning, offer powerful tools to address these challenges and enhance pharmacovigilance practices.
Leveraging AI for Advanced Pharmacovigilance Insights:
1. Automated Adverse Event Reporting
AI can automate the process of adverse event reporting, making it more efficient and accurate.
Natural Language Processing (NLP): NLP algorithms can process and analyze unstructured text data from various sources, including clinical notes, social media posts, and patient forums. By extracting relevant information on adverse events, NLP enhances the comprehensiveness of safety data.
Text Mining : Text mining techniques can identify patterns and trends in adverse event reports. These techniques can help in recognizing potential safety signals early, allowing for timely intervention.
2. Enhanced Signal Detection
Traditional signal detection methods often rely on statistical techniques that may miss subtle or rare signals. AI can enhance signal detection through advanced analytics.
Machine Learning Algorithms : Machine learning algorithms can analyze large datasets to identify patterns and correlations that may indicate potential safety issues. These algorithms can be trained to recognize complex relationships in the data, improving the accuracy of signal detection.
Deep Learning : Deep learning models, such as convolutional neural networks (CNNs) and recurrent neural networks (RNNs), can process high-dimensional data, such as genomic information and medical images. These models can identify intricate patterns and anomalies, enhancing the detection of safety signals.
3. Predictive Analytics
AI-powered predictive analytics can forecast potential adverse events and safety issues before they occur.
Risk Prediction Models : Machine learning models can predict the likelihood of adverse events based on patient characteristics, medication history, and genetic information. These models can help in stratifying patients by risk and tailoring treatment plans accordingly.
Time-Series Analysis : AI can analyze time-series data to identify trends and predict future safety issues. For example, time-series analysis can forecast the incidence of adverse events over time, helping in proactive risk management.
4. Real-Time Monitoring
AI enables real-time monitoring of pharmacovigilance data, allowing for prompt identification and response to safety signals.
Stream Processing : Stream processing technologies can handle continuous data streams from various sources, such as EHRs and social media. AI algorithms can analyze these streams in real time, identifying potential safety issues as they arise.
Dashboard and Visualization Tools : AI-powered dashboards and visualization tools provide real-time insights into pharmacovigilance data. These tools enable healthcare professionals and regulatory bodies to monitor safety signals and trends, facilitating quick decision-making.
5. Integration of Diverse Data Sources
AI can integrate data from diverse sources, providing a holistic view of drug safety.
Data Fusion : Data fusion techniques combine data from multiple sources, such as clinical trials, post-marketing surveillance, and patient registries. AI algorithms can process and analyze this integrated data, enhancing the understanding of drug safety.
Interoperability Standards : AI can facilitate the use of interoperability standards, such as HL7 FHIR, to integrate data from different systems. This integration ensures that safety data is comprehensive and accessible.
6. Improved Data Quality and Consistency
Ensuring high data quality and consistency is essential for accurate pharmacovigilance insights. AI can automate data validation and cleaning processes.
Data Cleaning Algorithms : AI algorithms can identify and correct errors, inconsistencies, and missing values in pharmacovigilance data. These algorithms ensure that the data is accurate and reliable.
Data Standardization : AI can standardize data from various sources, ensuring that it conforms to predefined formats and terminologies. Standardization enhances data consistency and facilitates more accurate analysis.
7. Supporting Regulatory Compliance
AI can assist in ensuring compliance with regulatory requirements and guidelines.
Automated Reporting : AI can automate the generation of regulatory reports, ensuring that they are accurate and timely. These reports include periodic safety update reports (PSURs) and risk management plans (RMPs).
Compliance Monitoring : AI-powered tools can monitor pharmacovigilance activities for compliance with regulatory standards. These tools can identify deviations and recommend corrective actions, ensuring adherence to regulations.
Case Studies: AI in Pharmacovigilance:
Case Study 1: AI-Driven Signal Detection at a Leading Pharmaceutical Company
A leading pharmaceutical company implemented AI-driven signal detection to enhance its pharmacovigilance processes. By using machine learning algorithms to analyze data from clinical trials and post-marketing surveillance, the company identified potential safety signals more quickly and accurately. This approach reduced the time needed for signal detection by 40% and improved the accuracy of adverse event identification.
Case Study 2: NLP for Adverse Event Reporting
A global healthcare organization utilized NLP to process unstructured data from electronic health records and social media. By extracting relevant information on adverse events, the organization improved the comprehensiveness of its safety data. This approach enabled the early detection of rare adverse events and enhanced the organization’s ability to respond to potential safety issues.
Case Study 3: Predictive Analytics in Pharmacovigilance
A research team developed predictive analytics models to forecast adverse events based on patient characteristics and medication history. These models helped in identifying high-risk patients and tailoring treatment plans to minimize adverse events. The implementation of predictive analytics resulted in a 30% reduction in adverse event incidence and improved patient outcomes.
Challenges and Ethical Considerations:
While AI offers significant advancements in pharmacovigilance, it also presents challenges and ethical considerations.
Data Privacy : The integration of diverse data sources raises concerns about patient privacy and data security. Ensuring that data is anonymized and securely managed is paramount.
Algorithm Bias : AI models can inherit biases present in training data, leading to skewed results. It is crucial to ensure that algorithms are trained on diverse, representative datasets to avoid disparities in healthcare outcomes.
Transparency and Explain ability : AI algorithms, particularly deep learning models, can be complex and difficult to interpret. Ensuring transparency and explain ability in AI-driven decisions is essential for trust and regulatory compliance.
Regulatory Challenges : The use of AI in pharmacovigilance must comply with regulatory standards and guidelines. Continuous collaboration between technology developers, healthcare providers, and regulatory bodies is necessary to ensure compliance and maintain public trust.
Future Directions:
The future of AI in pharmacovigilance is promising, with several advancements on the horizon.
Advanced AI Models : The development of more sophisticated AI models, such as reinforcement learning and generative adversarial networks (GANs), will enhance the accuracy and predictive capabilities of pharmacovigilance systems.
Personalized Pharmacovigilance : Personalized medicine approaches can be applied to pharmacovigilance, considering individual patient factors such as genetics and lifestyle to optimize drug safety.
Global Pharmacovigilance Networks : Creating global pharmacovigilance networks powered by AI can facilitate the sharing of data and insights across countries. This collaborative approach will enhance the monitoring of drug safety on a global scale.
Real-World Evidence Integration : Integrating real-world evidence from sources such as wearable devices and mobile health apps will provide a more comprehensive view of drug safety and effectiveness.
Conclusion:
AI is transforming pharmacovigilance by offering advanced solutions to manage and analyze vast amounts of data efficiently. By automating adverse event reporting, enhancing signal detection, enabling predictive analytics, facilitating real-time monitoring, integrating diverse data sources, improving data quality, and supporting regulatory compliance, AI significantly enhances pharmacovigilance practices.
However, it is essential to address challenges related to data privacy, algorithm bias, transparency, and regulatory compliance to fully realize the potential of AI in pharmacovigilance. As these technologies continue to evolve, they hold the promise of improving drug safety, patient outcomes, and public health worldwide.
Comments