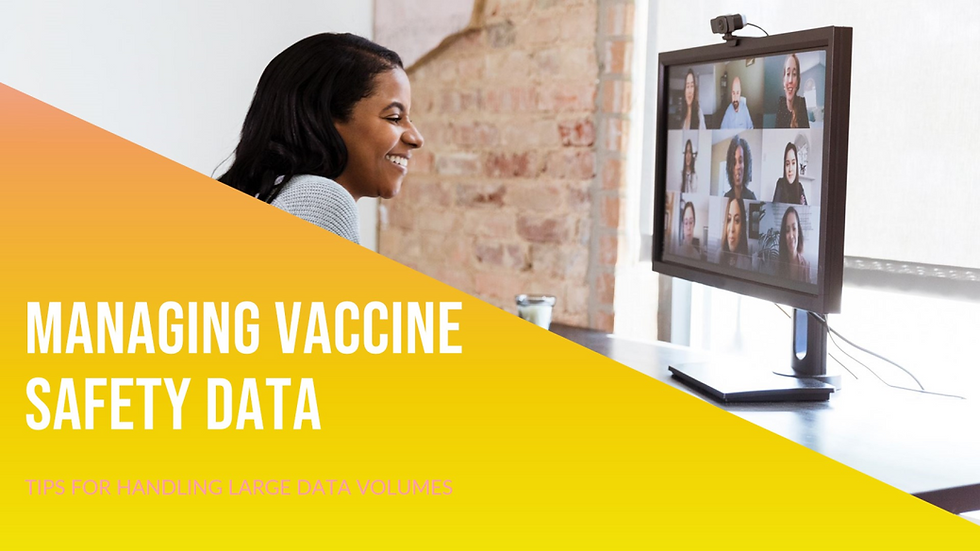
Managing data volume in vaccine safety databases is crucial for ensuring efficient data collection, storage, analysis, and utilization. This blog explores the challenges posed by large data volumes in vaccine safety databases, strategies to manage them effectively, technological advancements, and future directions.
Understanding the Challenges of Data Volume in Vaccine Safety Databases:
Vaccine safety databases manage vast amounts of data from various sources, including:
Clinical Trials: Data from vaccine clinical trials, including participant demographics, adverse events, and efficacy outcomes.
Pharmacovigilance Systems: Reports of adverse events following immunization (AEFI) submitted by healthcare providers, patients, and vaccine manufacturers.
Electronic Health Records (EHRs): Patient health information, vaccination histories, and medical records stored in electronic formats.
Real-World Data: Data from population-based studies, registries, surveillance systems, and social media platforms.
The volume, velocity, and variety of data generated present challenges in data management, processing, and analysis within vaccine safety databases.
Strategies for Managing Data Volume in Vaccine Safety Databases:
Effective management of data volume in vaccine safety databases involves implementing strategies to optimize data collection, storage, integration, and analysis:
1. Data Collection and Standardization
Define Data Standards: Establish standardized data collection protocols and formats to ensure consistency and interoperability across diverse data sources.
Automated Data Capture: Implement automated data capture mechanisms to streamline data entry, reduce errors, and enhance efficiency in data collection processes.
2. Data Storage and Infrastructure
Scalable Storage Solutions: Deploy scalable storage solutions, such as cloud-based platforms and distributed databases, to accommodate large volumes of data and facilitate seamless expansion.
Data Compression and Archiving: Utilize data compression techniques and archival strategies to optimize storage space and improve accessibility to historical data.
3. Data Integration and Interoperability
Integration Platforms: Implement data integration platforms and interoperability standards to consolidate data from disparate sources into a unified repository.
APIs and Data Pipelines: Develop application programming interfaces (APIs) and data pipelines to facilitate seamless data exchange and integration between different healthcare systems and databases.
4. Data Processing and Analysis
Parallel Processing: Utilize parallel processing techniques and distributed computing frameworks, such as Apache Hadoop and Spark, to accelerate data processing and analysis tasks.
Advanced Analytics: Implement advanced analytics tools, including machine learning algorithms and natural language processing (NLP), to derive actionable insights from large-scale datasets.
5. Data Governance and Quality Assurance
Data Governance Frameworks: Establish data governance frameworks to define roles, responsibilities, and policies for data management, privacy, and security.
Quality Assurance Measures: Implement rigorous quality assurance measures, including data validation, cleansing, and deduplication, to ensure data accuracy and reliability.
6. Data Security and Privacy
Data Encryption: Implement encryption protocols and secure transmission mechanisms to protect sensitive health information and maintain patient confidentiality.
Access Control: Enforce strict access control policies and authentication mechanisms to regulate data access and prevent unauthorized use or breaches.
Technological Advancements in Managing Data Volume:
Recent technological advancements offer innovative solutions to address the challenges of managing data volume in vaccine safety databases:
1. Cloud Computing
Scalability: Cloud computing platforms, such as Amazon Web Services (AWS) and Microsoft Azure, offer scalable storage and computing resources to handle large volumes of data.
Flexibility: Cloud-based solutions enable remote access, real-time data processing, and collaboration among geographically dispersed teams involved in vaccine safety surveillance.
2. Big Data Analytics
Real-Time Analytics: Big data analytics tools, like Apache Spark and Elasticsearch, support real-time data ingestion, processing, and visualization for timely insights into vaccine safety trends.
Predictive Modeling: Machine learning and predictive analytics algorithms enhance the detection of safety signals and forecast adverse events based on historical data patterns.
3. Artificial Intelligence (AI)
Automated Insights: AI-powered data analytics platforms automate data analysis tasks, identify patterns, and generate actionable insights to support decision-making in vaccine safety monitoring.
Natural Language Processing (NLP): NLP techniques analyze unstructured data, such as medical literature and social media posts, to extract relevant information and monitor public perceptions of vaccine safety.
4. Blockchain Technology
Data Integrity: Blockchain-based systems ensure data integrity and transparency by providing immutable records of vaccine safety data, enhancing trust and accountability in vaccine safety databases.
Decentralized Data Management: Decentralized blockchain networks facilitate secure data sharing and collaboration among stakeholders while protecting patient privacy and confidentiality.
Future Directions in Managing Data Volume:
Future directions in managing data volume in vaccine safety databases focus on leveraging emerging technologies and adopting innovative strategies:
1. Data Interoperability Standards
Standardization: Global adoption of interoperability standards, such as HL7 FHIR (Fast Healthcare Interoperability Resources), promotes seamless data exchange and integration across healthcare systems and vaccine safety databases.
Semantic Interoperability: Enhancing semantic interoperability facilitates meaningful data exchange by standardizing terminologies and ontologies used in vaccine safety surveillance.
2. Real-Time Surveillance Systems
Continuous Monitoring: Develop real-time surveillance systems that use AI and machine learning to monitor vaccine safety signals, detect anomalies, and respond promptly to emerging risks.
Predictive Analytics: Implement predictive modeling techniques to anticipate future vaccine safety trends and proactively manage public health interventions.
3. Patient-Centered Data Initiatives
Patient Engagement: Engage patients as active participants in vaccine safety surveillance through patient-reported outcomes, mobile health apps, and wearable devices to enhance data collection and monitoring.
Personalized Medicine: Integrate personalized medicine approaches, including genomic data and patient phenotypes, into vaccine safety databases to tailor immunization strategies and improve healthcare outcomes.
4. Ethical and Regulatory Frameworks
Ethical Guidelines: Develop ethical frameworks and regulatory guidelines to govern the responsible use of AI, big data analytics, and blockchain technologies in vaccine safety surveillance.
Data Privacy: Strengthen data privacy protections, consent mechanisms, and security protocols to safeguard patient information and uphold confidentiality in vaccine safety databases.
Conclusion:
Effectively managing data volume in vaccine safety databases is essential for optimizing data-driven decision-making, enhancing public health surveillance, and ensuring the safety and effectiveness of vaccines. By implementing strategies to streamline data collection, storage, integration, and analysis, healthcare organizations and public health authorities can leverage technological advancements to address the challenges posed by large-scale data management. Future innovations in cloud computing, big data analytics, AI, and blockchain technology offer promising opportunities to advance vaccine safety surveillance and protect global health outcomes. Embracing interoperability standards, patient-centered initiatives, and ethical guidelines will pave the way for a more integrated and responsive approach to managing data volume in vaccine safety databases.
Comments