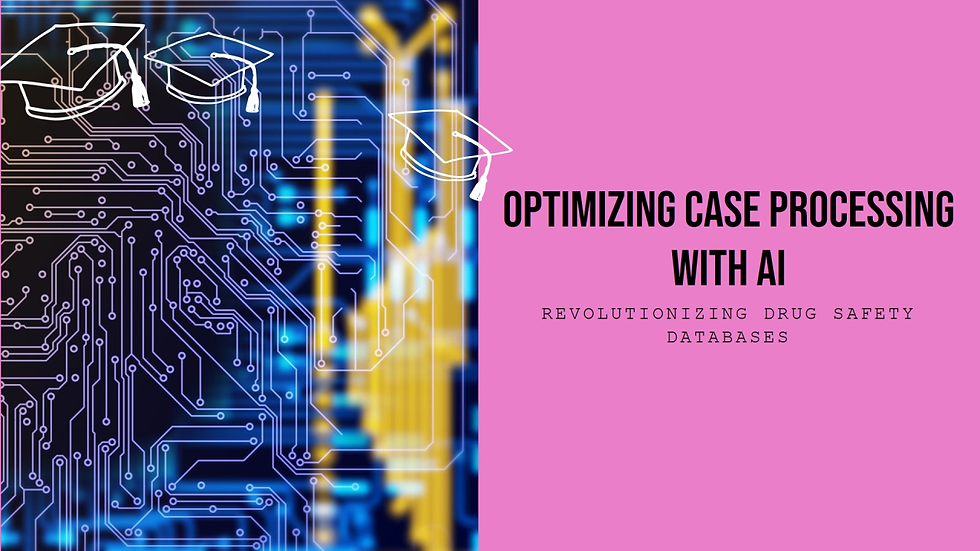
In the realm of pharmacovigilance, the efficient processing of adverse event reports is paramount for ensuring the safety of pharmaceutical products. With the ever-increasing volume of data in drug safety databases, manual case processing can be time-consuming and resource-intensive. However, Artificial Intelligence (AI) offers a solution by streamlining and optimizing case processing workflows. This blog delves into the strategies and benefits of integrating AI into case processing in drug safety databases.
Understanding Case Processing in Drug Safety:
Case processing involves the collection, assessment, and documentation of adverse event reports related to pharmaceutical products. This process is crucial for identifying potential safety concerns, assessing the causality of adverse events, and taking appropriate regulatory actions.
Traditional case processing workflows typically involve several manual steps:
Data Entry: Transcribing adverse event reports from various sources into the database.
Triage: Prioritizing cases based on severity and regulatory requirements.
Data Review: Analyzing the reported information to determine the relevance and credibility of adverse events.
Causality Assessment: Evaluating the likelihood that the pharmaceutical product caused the reported adverse event.
Reporting: Documenting the findings and submitting reports to regulatory authorities as required.
While these manual processes are essential, they can be labor-intensive, time-consuming, and prone to errors, especially as the volume of adverse event reports continues to grow.
The Role of AI in Optimizing Case Processing:
AI technologies, particularly machine learning and natural language processing (NLP), offer transformative capabilities for optimizing case processing workflows:
1. Data Extraction and Entry
AI algorithms can automate the extraction of relevant information from diverse sources, including:
Spontaneous Reporting Systems: Databases where healthcare professionals and consumers report adverse events.
Electronic Health Records (EHRs): Digital records of patients' medical histories and treatments.
Clinical Trials: Structured data from clinical studies investigating pharmaceutical products.
By automating data extraction and entry, AI reduces the burden of manual data entry, minimizes errors, and accelerates the processing of adverse event reports.
2. Triage and Prioritization:
Machine learning models can analyze the content of adverse event reports to prioritize cases based on severity and regulatory requirements. By identifying critical cases that require immediate attention, AI ensures that resources are allocated efficiently and response times are minimized.
3. Data Review and Causality Assessment:
AI-powered algorithms can assist pharmacovigilance professionals in reviewing adverse event reports and assessing the causality of reported events. These algorithms can:
Identify Relevant Information: Extract key information such as patient demographics, medical history, and concomitant medications from unstructured text data.
Flag Potential Safety Signals: Highlight patterns and anomalies in the data that may indicate emerging safety concerns.
Predict Causality: Assess the likelihood that the pharmaceutical product caused the reported adverse event based on historical data and known risk factors.
By automating data review and causality assessment, AI accelerates the identification of potential safety signals and enables more informed decision-making.
4. Reporting and Documentation:
AI can streamline the process of generating regulatory reports by automating the compilation of relevant data and generating standardized reports. By automating this process, AI ensures consistency, reduces the risk of errors, and expedites regulatory submissions.
Strategies for Implementing AI in Case Processing:
To effectively integrate AI into case processing workflows, pharmacovigilance organizations can adopt the following strategies:
1. Data Preparation and Annotation
Ensure that adverse event reports are properly prepared and annotated to facilitate AI-powered analysis. This may involve:
Standardizing Data Formats: Ensure that data from different sources are formatted consistently to enable seamless integration.
Annotating Training Data: Label adverse event reports with relevant information to train machine learning models effectively.
2. Model Development and Validation
Develop and validate machine learning models for various tasks, including data extraction, triage, data review, and causality assessment. Key steps in model development and validation include:
Feature Engineering: Identify relevant features and variables to include in the model.
Training and Testing: Train the model on labeled data and evaluate its performance on unseen data.
Validation: Validate the model's performance against established benchmarks and regulatory requirements.
3. Integration with Existing Systems
Integrate AI-powered case processing solutions seamlessly with existing pharmacovigilance systems and workflows. Ensure that AI tools can:
Import Data: Retrieve adverse event reports from databases and other sources for analysis.
Export Results: Export processed data and reports back to the pharmacovigilance database or reporting system.
Interface with Users: Provide user-friendly interfaces for pharmacovigilance professionals to interact with AI-powered tools.
4. Continuous Monitoring and Improvement
Continuously monitor the performance of AI models and update them as needed to ensure optimal performance. This may involve:
Monitoring Model Performance: Track key performance metrics such as accuracy, precision, and recall over time.
Updating Models: Incorporate new data and insights into AI models to improve their predictive capabilities.
Addressing Feedback: Solicit feedback from pharmacovigilance professionals and end-users to identify areas for improvement.
Benefits of AI in Case Processing:
The integration of AI into case processing workflows offers several benefits:
1. Increased Efficiency
AI automates manual tasks, such as data entry and review, reducing processing times and freeing up resources for higher-value activities.
2. Enhanced Accuracy
AI-powered algorithms can analyze large volumes of data with a high degree of accuracy, minimizing errors and ensuring consistency in data processing.
3. Timely Detection of Safety Signals
By automating data review and analysis, AI enables the timely detection of potential safety signals, allowing pharmacovigilance professionals to take proactive measures to protect public health.
4. Improved Regulatory Compliance
AI streamlines regulatory reporting processes, ensuring that adverse event reports are documented and submitted accurately and in compliance with regulatory requirements.
5. Scalability
AI-powered solutions can scale to handle growing volumes of adverse event reports, ensuring that pharmacovigilance systems remain robust and effective as data volumes increase.
Challenges and Considerations:
While AI offers significant benefits for optimizing case processing in drug safety databases, several challenges and considerations must be addressed:
1. Data Quality
Ensuring the quality and consistency of data is essential for the effectiveness of AI-powered case processing solutions. Poor-quality data can lead to inaccurate predictions and unreliable insights.
2. Interpretability
AI models, particularly deep learning models, can be complex and difficult to interpret. Ensuring that AI-driven insights are transparent and understandable is important for regulatory compliance and trust.
3. Regulatory Compliance
Pharmacovigilance is a highly regulated field, and AI-powered solutions must comply with regulatory requirements and guidelines. Ensuring compliance while leveraging AI capabilities is a critical consideration.
4. Ethical Use of AI
The use of AI in healthcare raises ethical considerations, including data privacy, fairness, and the potential for algorithmic bias. Ensuring ethical use of AI is essential for maintaining public trust and safeguarding patient welfare.
5. Human Oversight
While AI can automate many aspects of case processing, human oversight remains crucial for ensuring the accuracy and reliability of AI-driven insights. Pharmacovigilance professionals should remain actively involved in the case processing process and exercise judgment where necessary.
Conclusion:
In conclusion, the integration of Artificial Intelligence (AI) into case processing workflows in drug safety databases holds immense promise for optimizing pharmacovigilance practices. By automating manual tasks, enhancing accuracy, and enabling timely detection of safety signals, AI streamlines case processing and empowers pharmacovigilance professionals to protect public health more effectively.
Comments