How to Optimize Case Processing with AI in Medical Device Safety
- Chaitali Gaikwad
- Jun 5, 2024
- 6 min read
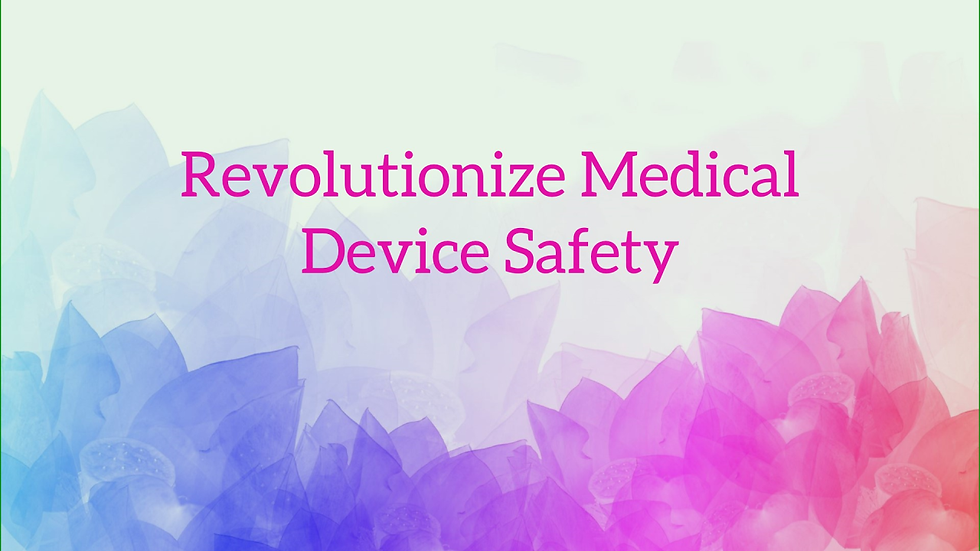
In the rapidly evolving landscape of healthcare, ensuring the safety of medical devices is of paramount importance. Medical device safety encompasses a broad spectrum of activities, from monitoring adverse events to ensuring compliance with regulatory standards. Traditional methods of case processing in medical device safety can be labor-intensive, time-consuming, and prone to human error. However, the integration of artificial intelligence (AI) offers promising solutions to optimize these processes, enhancing efficiency, accuracy, and overall safety outcomes.
Understanding Case Processing in Medical Device Safety:
Case processing in medical device safety involves the systematic collection, analysis, and reporting of adverse events related to medical devices. This process includes:
Adverse Event Reporting: Collecting data on incidents where medical devices may have caused harm to patients.
Signal Detection: Identifying patterns or trends in the data that may indicate a potential safety issue.
Risk Assessment: Evaluating the severity and likelihood of identified risks.
Regulatory Compliance: Ensuring that all reporting and risk assessment activities comply with regulatory requirements.
Traditional methods rely heavily on manual processes, which can be slow and inefficient. AI offers the potential to transform these processes by automating routine tasks, improving data accuracy, and enabling more sophisticated analysis.
The Role of AI in Optimizing Case Processing:
AI, particularly machine learning (ML) and natural language processing (NLP), can significantly enhance various aspects of case processing in medical device safety. Here’s how AI can optimize each step:
1. Automated Adverse Event Reporting
Traditionally, adverse event reporting involves manually reviewing and entering data from various sources, such as patient reports, clinical studies, and healthcare provider feedback. This process is not only time-consuming but also prone to errors and inconsistencies.
AI-Powered Automation: AI can automate the extraction and entry of data from various sources. NLP algorithms can scan text from medical records, incident reports, and other documents to identify relevant information. Machine learning models can classify and prioritize these reports based on predefined criteria, ensuring that the most critical cases are addressed promptly.
Case Study: The FDA has explored the use of AI to automate the processing of adverse event reports. AI algorithms have been developed to scan large volumes of reports, extracting relevant information and identifying potential safety signals more quickly than human analysts.
2. Enhanced Signal Detection
Signal detection involves identifying patterns in adverse event data that may indicate a potential safety issue. Traditional methods often rely on basic statistical techniques, which can miss subtle or emerging trends.
Advanced Analytics with AI: AI can apply more sophisticated analytical techniques to detect signals. Machine learning algorithms can analyze large datasets to identify complex patterns and correlations that may not be apparent with traditional methods. These models can also be continuously updated with new data, improving their accuracy over time.
3. Improved Risk Assessment
Once potential safety signals are identified, they must be assessed to determine the level of risk they pose. This involves evaluating the severity of the adverse events and the likelihood of their occurrence.
Risk Stratification with AI: AI can enhance risk assessment by providing more accurate and granular stratification of risks. Machine learning models can analyze multiple factors, such as patient demographics, device characteristics, and clinical outcomes, to assess risk levels. AI can also simulate various scenarios to predict the potential impact of identified risks.
Case Study: AI-driven risk assessment tools have been developed to evaluate the safety of implantable devices. These tools use machine learning algorithms to analyze patient data and predict the likelihood of adverse events. This enables healthcare providers to make more informed decisions about device selection and patient management.
4. Ensuring Regulatory Compliance
Regulatory compliance is a critical aspect of medical device safety. It involves ensuring that all adverse event reporting and risk assessment activities meet the requirements set by regulatory bodies, such as the FDA or the European Medicines Agency (EMA).
Compliance Automation with AI: AI can streamline regulatory compliance by automating the generation of compliance reports and ensuring that all required information is accurately captured. NLP can be used to extract relevant data from adverse event reports and clinical studies, while machine learning models can ensure that this data meets regulatory standards.
Case Study: AI tools have been developed to assist in the preparation of regulatory submissions. These tools can automatically generate reports that meet the specific requirements of different regulatory bodies, reducing the time and effort required for compliance.
Benefits of AI in Case Processing for Medical Device Safety:
The integration of AI into case processing for medical device safety offers several key benefits:
1. Increased Efficiency
By automating routine tasks, AI can significantly reduce the time and effort required for case processing. This allows healthcare professionals to focus on more complex and value-added activities, such as risk assessment and decision-making.
2. Enhanced Accuracy
AI algorithms can analyze data with a high degree of accuracy, reducing the risk of errors and inconsistencies. This leads to more reliable adverse event reporting, signal detection, and risk assessment.
3. Real-Time Monitoring
AI enables real-time monitoring of adverse events and safety signals. This allows for the early detection of potential safety issues, enabling prompt intervention and mitigation.
4. Better Resource Allocation
By prioritizing adverse event reports based on their severity and likelihood, AI can help allocate resources more effectively. This ensures that the most critical cases receive the attention they need.
5. Improved Regulatory Compliance
AI can streamline the generation of compliance reports and ensure that all required information is accurately captured. This reduces the burden of regulatory compliance and minimizes the risk of non-compliance.
Challenges and Considerations:
While the benefits of AI in case processing for medical device safety are clear, there are also several challenges and considerations to address:
1. Data Quality and Availability
AI algorithms require large amounts of high-quality data to function effectively. Ensuring that accurate and comprehensive data is available can be a challenge, particularly in cases where data is fragmented across different systems and sources.
2. Algorithm Transparency and Bias
Ensuring the transparency and explain ability of AI algorithms is crucial, particularly in healthcare where decisions can have significant consequences. It is also important to address potential biases in the data and algorithms to ensure fair and equitable outcomes.
3. Integration with Existing Systems
Integrating AI technologies with existing healthcare systems can be complex and require significant infrastructure upgrades. Ensuring compatibility and seamless integration is essential for the successful implementation of AI-driven case processing.
4. Regulatory and Ethical Issues
The deployment of AI in medical device safety is subject to regulatory scrutiny. Ensuring that AI-powered tools meet regulatory standards and receive necessary approvals can be a complex and time-consuming process. Ethical considerations, such as maintaining patient trust and ensuring the responsible use of AI, also play a critical role.
Future Prospects:
The future of AI in optimizing case processing for medical device safety is promising, with ongoing advancements likely to bring even greater benefits. Here are some potential developments to watch for:
1. Integration with Big Data Analytics
The integration of AI with big data analytics can enable the analysis of vast amounts of data from multiple sources, providing more comprehensive insights into medical device safety. This can enhance signal detection, risk assessment, and overall safety outcomes.
2. Development of Predictive Models
The development of predictive models using AI can enable proactive risk management. These models can predict potential adverse events before they occur, allowing for preventive measures to be taken.
3. Enhanced Collaboration and Data Sharing
AI can facilitate enhanced collaboration and data sharing among healthcare providers, regulatory bodies, and manufacturers. This can lead to more comprehensive and accurate safety assessments, improving overall patient outcomes.
4. Continuous Learning and Improvement
AI algorithms can be continuously updated with new data and insights, improving their accuracy and effectiveness over time. This ensures that case processing for medical device safety remains up-to-date and relevant in an ever-evolving healthcare landscape.
Conclusion:
AI has the potential to revolutionize case processing for medical device safety, offering significant improvements in efficiency, accuracy, and overall safety outcomes. By automating routine tasks, enhancing data analysis, and enabling real-time monitoring, AI can transform how adverse events are reported, signals are detected, and risks are assessed. While there are challenges to address, the future prospects of AI in this field are promising, paving the way for a safer and more efficient healthcare system. As technology continues to advance, the integration of AI into medical device safety will likely become even more seamless, leading to better patient outcomes and a more robust healthcare infrastructure.
Comments