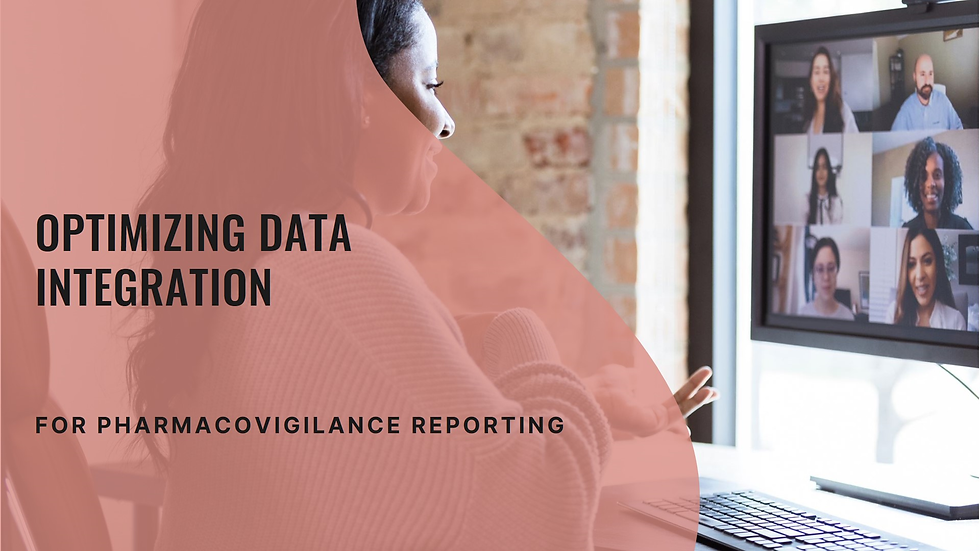
Pharmacovigilance, the science of monitoring the safety of pharmaceutical products, plays a crucial role in ensuring patient safety by detecting, assessing, and preventing adverse drug reactions (ADRs). As the pharmaceutical industry grows and evolves, the volume and complexity of data involved in pharmacovigilance have increased significantly. Efficient data integration is key to optimizing pharmacovigilance reporting, enabling timely and accurate safety assessments, regulatory compliance, and ultimately, protecting public health.
In this blog, we will explore the importance of data integration in pharmacovigilance, the challenges faced, and practical strategies to optimize data integration for more effective pharmacovigilance reporting.
The Importance of Data Integration in Pharmacovigilance
Pharmacovigilance relies on the collection and analysis of data from a variety of sources, including clinical trials, post-marketing surveillance, electronic health records (EHRs), social media, and spontaneous reporting systems. Data integration is the process of combining data from these diverse sources into a unified system that allows for comprehensive analysis.
Effective data integration enables pharmacovigilance teams to:
Enhance Signal Detection: By integrating data from multiple sources, patterns that might indicate potential safety signals can be identified more accurately and quickly.
Improve Decision-Making: A holistic view of all available data supports better-informed decisions regarding drug safety and risk management.
Ensure Regulatory Compliance: Regulatory authorities, such as the FDA and EMA, require timely and accurate pharmacovigilance reporting. Integrated data systems ensure that reports meet regulatory standards and deadlines.
Reduce Redundancy and Errors: Automated data integration reduces the need for manual data entry and the risk of human error, ensuring that pharmacovigilance reports are both accurate and consistent.
Challenges in Data Integration for Pharmacovigilance
While data integration is essential, it presents several challenges that need to be addressed for successful implementation:
Data Silos: Data is often stored in disparate systems across different departments, making it difficult to access and integrate. This fragmentation can lead to incomplete or delayed safety assessments.
Data Quality and Consistency: Data from different sources may vary in quality, format, and structure. Inconsistent or poor-quality data can compromise the accuracy of pharmacovigilance reporting.
Regulatory and Compliance Requirements: Different regions have varying regulatory requirements for pharmacovigilance reporting. Ensuring that integrated data meets all necessary compliance standards can be complex and time-consuming.
Interoperability Issues: The lack of standardization in data formats and protocols can hinder the seamless exchange of information between systems, making integration difficult.
Data Privacy and Security: Integrating data from multiple sources raises concerns about the privacy and security of sensitive patient information. Compliance with data protection regulations, such as GDPR, is essential.
Strategies to Optimize Data Integration for Pharmacovigilance
To overcome these challenges and optimize data integration for pharmacovigilance reporting, organizations can adopt the following strategies:
1. Implement a Centralized Data Repository
A centralized data repository is a single, unified database that consolidates data from various sources into one location. This approach eliminates data silos and provides a comprehensive view of all relevant information. The repository should be designed to support easy access, retrieval, and analysis of data by pharmacovigilance teams.
When implementing a centralized repository, consider the following best practices:
Data Standardization: Ensure that all incoming data is standardized to a common format. This may involve converting data from different formats into a unified structure that supports consistent analysis.
Scalability: Design the repository to accommodate the growing volume of data as new sources and types of data are integrated.
Security Measures: Implement robust security protocols to protect sensitive data and comply with regulatory requirements for data privacy.
2. Adopt Advanced Data Integration Technologies
Advanced data integration technologies can streamline the process of combining data from multiple sources, improving efficiency and accuracy. Some of the most effective technologies include:
ETL Tools (Extract, Transform, Load): ETL tools automate the process of extracting data from various sources, transforming it into a consistent format, and loading it into the centralized repository. These tools reduce the need for manual data handling and minimize the risk of errors.
Application Programming Interfaces (APIs): APIs facilitate the seamless exchange of data between different systems, enabling real-time data integration. By using APIs, pharmacovigilance teams can access up-to-date information from multiple sources, improving the timeliness of safety assessments.
Cloud-Based Solutions: Cloud-based data integration platforms offer scalability, flexibility, and enhanced collaboration capabilities. They enable organizations to integrate data from global sources without the limitations of on-premise infrastructure.
3. Leverage Artificial Intelligence and Machine Learning
Artificial intelligence (AI) and machine learning (ML) technologies can significantly enhance data integration and analysis in pharmacovigilance. These technologies can process large volumes of data quickly and identify patterns that may indicate potential safety signals.
Automated Signal Detection: Machine learning algorithms can analyze integrated data to detect safety signals more rapidly and accurately than traditional methods. These algorithms can continuously learn and improve, increasing their effectiveness over time.
Natural Language Processing (NLP): NLP can be used to extract relevant information from unstructured data sources, such as medical records, social media, and scientific literature. Integrating this information with structured data provides a more comprehensive view of drug safety.
Predictive Analytics: AI-powered predictive analytics can forecast potential safety issues based on historical data, enabling proactive risk management and decision-making.
4. Ensure Data Quality and Governance
High-quality data is essential for accurate pharmacovigilance reporting. Organizations must establish robust data governance practices to ensure that integrated data is consistent, reliable, and compliant with regulatory standards.
Data Validation: Implement automated data validation processes to detect and correct errors, inconsistencies, and duplicates in real-time.
Data Lineage: Track the origin and transformation of data throughout the integration process to ensure transparency and accountability. This is particularly important for meeting regulatory requirements.
Regular Audits: Conduct regular audits of the data integration process to identify and address any issues related to data quality, security, or compliance.
5. Foster Collaboration Between Departments
Effective data integration requires collaboration between different departments, including pharmacovigilance, IT, regulatory affairs, and data management. By fostering a collaborative culture, organizations can ensure that all stakeholders are aligned in their goals and work together to optimize data integration.
Cross-Functional Teams: Establish cross-functional teams to oversee the data integration process. These teams should include representatives from all relevant departments to ensure that everyone’s needs are met.
Training and Education: Provide ongoing training and education for all stakeholders involved in data integration. This will help them understand the importance of data integration, the tools and technologies used, and their role in the process.
Clear Communication: Establish clear lines of communication between departments to facilitate the exchange of information and resolve any issues that arise during the integration process.
6. Align with Regulatory Requirements
Regulatory compliance is a critical aspect of pharmacovigilance reporting. Organizations must ensure that their data integration processes align with the requirements of regulatory authorities in different regions.
Global Harmonization: Aim for global harmonization of data integration processes to meet the standards of multiple regulatory authorities. This may involve adopting international standards, such as the International Council for Harmonisation (ICH) guidelines.
Regulatory Updates: Stay informed about changes in regulatory requirements and update data integration processes accordingly. This will help ensure ongoing compliance and avoid potential penalties or delays in reporting.
Documentation: Maintain thorough documentation of all data integration processes, including data sources, transformation methods, and validation steps. This documentation will be essential for regulatory inspections and audits.
7. Monitor and Evaluate the Integration Process
Continuous monitoring and evaluation of the data integration process are essential to ensure its ongoing effectiveness. By regularly assessing the performance of data integration systems, organizations can identify areas for improvement and make necessary adjustments.
Key Performance Indicators (KPIs): Establish KPIs to measure the success of data integration efforts. These may include metrics related to data quality, integration speed, and the accuracy of pharmacovigilance reports.
Feedback Mechanisms: Implement feedback mechanisms to gather input from pharmacovigilance teams and other stakeholders on the effectiveness of data integration processes. Use this feedback to drive continuous improvement.
Iterative Improvements: Treat data integration as an iterative process, with regular updates and enhancements based on performance data and feedback. This approach ensures that the integration process remains aligned with organizational goals and evolving regulatory requirements.
Conclusion:
Optimizing data integration for pharmacovigilance reporting is essential for ensuring the safety of pharmaceutical products and protecting public health. By implementing a centralized data repository, adopting advanced integration technologies, leveraging AI and machine learning, ensuring data quality, fostering collaboration, aligning with regulatory requirements, and continuously monitoring and evaluating the integration process, organizations can overcome the challenges of data integration and enhance the effectiveness of their pharmacovigilance efforts.
In a rapidly evolving pharmaceutical landscape, where new data sources and regulatory requirements are constantly emerging, organizations must remain agile and proactive in their approach to data integration. By doing so, they can ensure that their pharmacovigilance systems are equipped to handle the complexities of modern drug safety monitoring and continue to deliver accurate, timely, and compliant reports that safeguard patient well-being.
Comments