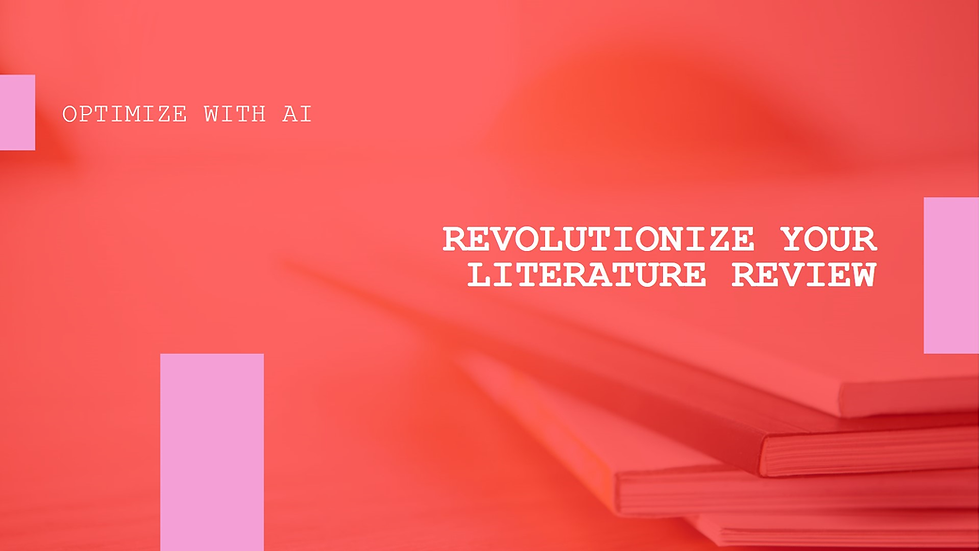
In the realm of academic research and scientific inquiry, literature reviews are fundamental. They provide a comprehensive overview of existing research, identify gaps, and help frame new studies. Traditionally, conducting a literature review has been a time-consuming and labor-intensive process, often involving manual searches through databases, sifting through numerous articles, and synthesizing information. However, with advancements in artificial intelligence (AI), the process of literature review is undergoing a significant transformation. This blog explores how AI can optimize literature reviews, making them more efficient, accurate, and insightful.
The Traditional Literature Review Process:
Before diving into how AI optimizes literature reviews, it’s important to understand the traditional process:
Defining the Research Question: Researchers start by clearly defining their research question or hypothesis. This step guides the focus of the literature review.
Searching Databases: Researchers perform searches across various academic databases (e.g., PubMed, Google Scholar, Web of Science) using keywords related to their topic.
Screening and Selection: Out of the search results, researchers manually screen and select relevant articles based on titles, abstracts, and keywords.
Reading and Analyzing: Selected articles are read in detail to extract key information, such as findings, methodologies, and theoretical frameworks.
Synthesizing Information: Researchers synthesize the information gathered to identify trends, gaps, and areas for further research.
Writing the Review: The final step involves writing a coherent and structured review that summarizes the findings and provides a critical analysis of the literature.
This traditional approach, while effective, has its limitations, especially when dealing with vast amounts of data and rapidly evolving fields.
AI-Driven Optimization of Literature Reviews:
AI technologies, particularly machine learning and natural language processing (NLP), offer powerful tools to enhance various stages of the literature review process. Here’s how AI can optimize each step:
1. Enhanced Search Capabilities
AI can significantly improve the efficiency and effectiveness of literature searches:
Semantic Search: Unlike traditional keyword-based searches, AI-driven semantic search understands the context and meaning behind search queries. This results in more relevant and accurate search results.
Automated Database Searches: AI tools can simultaneously search multiple databases and academic repositories, consolidating results in a unified platform. This reduces the time spent manually searching each database.
Personalized Recommendations: AI algorithms can analyze a researcher’s past work and search behavior to recommend relevant articles and studies tailored to their specific research interests.
2. Efficient Screening and Selection
AI accelerates the process of screening and selecting relevant literature:
Abstract and Title Screening: AI-powered tools can quickly scan titles and abstracts to identify articles that are most likely to be relevant, reducing the need for manual screening.
Automatic Categorization: Machine learning algorithms can categorize articles based on predefined criteria, such as study type, methodology, or subject matter, making it easier to sort and prioritize research.
Duplicate Detection: AI systems can identify and remove duplicate articles from search results, ensuring that researchers only review unique sources.
3. Advanced Reading and Analysis
AI enhances the process of reading and analyzing articles:
Text Extraction: NLP tools can extract key information from full-text articles, such as research findings, methodologies, and conclusions, without requiring manual extraction.
Sentiment Analysis: AI can perform sentiment analysis to assess the tone and bias of research papers, helping researchers understand the perspective of different studies.
Automated Summarization: AI-driven summarization tools can generate concise summaries of lengthy articles, making it easier for researchers to grasp the main points quickly.
4. Insightful Synthesis
AI facilitates a more effective synthesis of literature:
Trend Analysis: Machine learning algorithms can analyze large volumes of text to identify emerging trends, patterns, and correlations within the literature.
Gap Identification: AI tools can highlight gaps in the existing research by comparing new findings with previously published studies, helping researchers pinpoint areas for further investigation.
Visualization Tools: AI-powered visualization tools can create charts, graphs, and network diagrams that illustrate relationships between different studies, methodologies, and findings.
5. Streamlined Writing and Documentation
AI tools can assist in the writing and documentation phase:
Automated Citation Management: AI-driven citation management tools can automatically generate and format citations in various styles, such as APA, MLA, or Chicago.
Writing Assistance: AI writing assistants can help with drafting sections of the review, suggesting improvements, and ensuring coherence and consistency throughout the document.
Plagiarism Detection: AI-powered plagiarism detection tools can check the review for any unintentional similarities with existing literature, ensuring originality and academic integrity.
Practical Examples and Tools:
To illustrate the impact of AI on literature reviews, let’s explore some practical examples and tools:
1. AI-Powered Literature Review Platforms
Iris.ai: Iris.ai uses AI to assist researchers in mapping out the existing literature related to their research question. It provides semantic search capabilities and automatically categorizes articles based on their relevance.
Semantic Scholar: Semantic Scholar employs machine learning algorithms to enhance search accuracy and recommend relevant papers. It also provides citation graphs and influential papers related to the topic of interest.
Sage Research Methods: This platform utilizes AI to suggest relevant literature and methods for specific research questions. It also offers tools for data visualization and analysis.
2. Natural Language Processing Tools
PubMed’s NLP Tools: PubMed integrates NLP techniques to improve search results and article recommendations. It helps researchers find relevant studies more efficiently.
Text Mining Tools: Tools like Voyant Tools and Leximancer use text mining and NLP techniques to analyze and visualize large volumes of text, aiding in the synthesis of literature.
3. Citation and Writing Tools
Zotero: Zotero is a reference management tool that uses AI to organize and cite research sources. It helps researchers manage citations and generate bibliographies.
Grammarly: Grammarly’s AI-powered writing assistant helps researchers with grammar, style, and coherence in their literature review drafts.
Challenges and Considerations:
While AI offers numerous benefits, there are challenges and considerations to keep in mind:
Data Quality: The effectiveness of AI tools depends on the quality and comprehensiveness of the data they are trained on. Inaccurate or incomplete data can lead to suboptimal results.
Algorithm Bias: AI algorithms can inherit biases from the training data, which may affect the relevance and accuracy of search results and recommendations. Continuous monitoring and refinement are necessary to address these biases.
Human Oversight: AI tools should complement, not replace, human expertise. Researchers should critically evaluate AI-generated results and ensure that they align with their research objectives.
Ethical and Privacy Concerns: Using AI in literature reviews raises ethical and privacy considerations, such as ensuring data security and protecting intellectual property rights.
Future Directions:
The future of AI in literature review holds exciting possibilities:
Integration with Emerging Technologies: Combining AI with technologies like blockchain for data integrity and augmented reality for interactive visualizations could further enhance the literature review process.
Personalized AI Tools: Future AI tools may offer even more personalized recommendations and insights, tailored to individual researchers’ needs and preferences.
Enhanced Collaboration: AI-driven platforms could facilitate collaboration among researchers by integrating findings from multiple sources and fostering interdisciplinary research.
Conclusion:
AI is transforming the literature review process by enhancing search capabilities, streamlining screening and selection, advancing analysis, and facilitating insightful synthesis. By leveraging machine learning and natural language processing, researchers can conduct more efficient and accurate literature reviews, ultimately leading to better-informed research outcomes. While challenges remain, the integration of AI into literature reviews promises to revolutionize academic research, making it more accessible, insightful, and impactful. As AI technologies continue to evolve, their role in literature reviews will undoubtedly become even more profound, driving innovation and excellence in research.
Comments