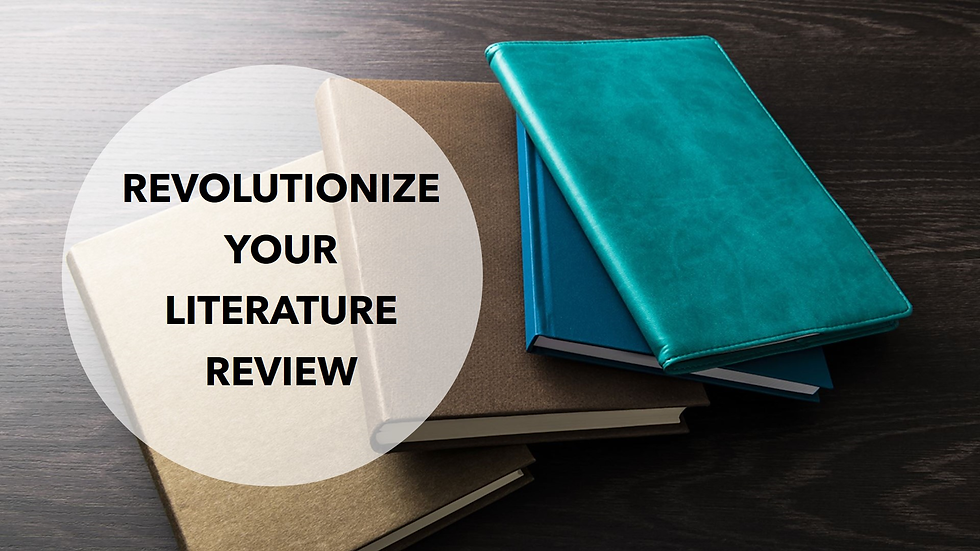
In recent years, artificial intelligence (AI) has emerged as a transformative force across various industries. Its applications in healthcare, finance, manufacturing, and education have revolutionized workflows, improving efficiency and reducing the time required for complex tasks. One such area where AI is making a significant impact is in literature reviews, particularly in scientific research and regulatory domains like pharmacovigilance, where vast amounts of data must be analyzed rapidly and accurately.
Conducting a literature review is a crucial component of research and analysis, but it often presents challenges in terms of time, effort, and accuracy. The manual process involves reviewing numerous scientific papers, reports, and other publications, which can be time-consuming and prone to human error. AI-powered tools are now being used to streamline this process, making it more efficient and ensuring comprehensive and accurate reviews.
This blog will explore how to optimize literature review processes with AI, focusing on how these advanced technologies can enhance accuracy, speed, and overall quality of research outcomes.
1. Understanding the Challenges of Traditional Literature Reviews
Before diving into AI's role, it’s essential to understand the challenges inherent in traditional literature reviews. Researchers often face issues such as:
Time-Consuming Process: Literature reviews typically require researchers to sift through large databases of academic articles, clinical studies, patents, and more. It can take weeks or months to collect and analyze all the relevant data.
Information Overload: With the growing number of publications, especially in scientific fields, researchers are overwhelmed with vast volumes of data, making it difficult to track every significant finding.
Human Error and Bias: Even skilled researchers may inadvertently miss key studies or introduce bias by focusing on certain types of publications. This can affect the quality of the final review and lead to incomplete conclusions.
Lack of Standardization: Literature reviews, when done manually, often suffer from inconsistencies in terms of how sources are selected, analyzed, and interpreted.
These challenges highlight the need for more efficient, accurate, and scalable solutions, which is where AI comes into play.
2. AI in Literature Reviews: An Overview
AI technologies, particularly natural language processing (NLP) and machine learning (ML), are increasingly being used to optimize literature reviews. AI-powered tools can automatically analyze, categorize, and extract relevant information from vast datasets, significantly reducing the time required to conduct thorough reviews.
Key AI technologies that enhance literature reviews include:
Natural Language Processing (NLP): NLP enables machines to read, understand, and derive meaning from human language. It’s critical for AI to comprehend complex scientific terminology and concepts in research articles, making it a powerful tool in literature review automation.
Machine Learning (ML): ML models can be trained on large datasets of scientific papers, learning patterns and identifying trends across various studies. These models can help researchers filter out irrelevant information and focus on critical findings.
AI-Powered Search Engines: AI-driven search engines and databases optimize how researchers search for publications, providing more accurate and relevant results. These tools can prioritize the most influential studies, saving time and improving the quality of the literature review.
3. Steps to Optimize Literature Reviews with AI
To fully leverage AI for optimizing literature reviews, researchers and organizations must follow a series of steps that integrate AI tools effectively into their workflows.
3.1. Selecting the Right AI Tool
There are several AI-based literature review tools available, each with its specific capabilities. The choice of tool depends on the type of research, volume of data, and the specific goals of the literature review. Some popular AI-driven platforms include:
Iris.ai: A comprehensive AI-based literature review tool that helps researchers find relevant academic papers quickly and summarizes them.
Rayyan: A machine learning-based tool designed to assist in systematic reviews. It aids in the selection and categorization of studies.
SciNote: A tool that uses NLP and ML to assist in the literature review process, helping researchers structure their workflows and identify relevant studies more efficiently.
Researchers should evaluate each platform’s features, such as automated citation management, ability to filter search results, and integration with existing databases like PubMed or Google Scholar.
3.2. Using AI for Data Collection
Data collection is one of the most time-consuming aspects of literature reviews. AI-powered tools can drastically speed up this process by automating the search for relevant studies. AI systems can crawl through vast databases, selecting only the most pertinent articles based on keywords, study designs, and other customizable filters.
NLP algorithms can interpret scientific abstracts, titles, and even entire papers to quickly identify the most relevant studies, allowing researchers to focus on deeper analysis rather than the initial search and retrieval process.
3.3. Automating Study Selection
Once the initial dataset is collected, the next step is to screen the studies. Traditional literature reviews often involve a manual review of abstracts and full-text articles to assess their relevance. AI can automate this process by learning from researchers’ inclusion and exclusion criteria, reducing the likelihood of overlooking important studies.
Machine learning models can analyze thousands of papers and quickly flag those that meet predefined criteria, significantly speeding up the study selection process. This automated screening ensures consistency and reduces human bias.
3.4. Extracting Key Information
After selecting relevant studies, researchers must extract data for analysis. This process involves identifying key elements such as study objectives, methodologies, results, and conclusions. AI-powered text mining tools can automatically extract this information from selected papers, organizing it in structured formats for easy comparison and synthesis.
NLP technologies can summarize the key findings of multiple studies, offering researchers a concise overview of the literature. Some AI tools also include visualization features, allowing researchers to see trends and patterns in the data.
3.5. Ensuring Comprehensive Coverage
AI tools help ensure that no critical studies are missed during the literature review process. Traditional methods often result in gaps due to limited time or human error. AI, however, can continuously scan for new publications in real-time, providing updates on relevant studies as they are published.
By using AI to track new developments, researchers can maintain comprehensive and up-to-date literature reviews, ensuring that their work reflects the latest available data.
3.6. Reducing Bias
One of the critical advantages of AI in literature reviews is its ability to minimize bias. Human reviewers may unintentionally favor certain types of studies or findings, leading to skewed results. AI systems, on the other hand, apply consistent criteria and algorithms to assess all relevant studies, ensuring an objective review process.
AI tools can also identify studies that present conflicting findings or highlight gaps in the research, allowing for a more balanced and nuanced literature review.
4. Integrating AI with Human Expertise
While AI significantly optimizes the literature review process, it should not replace human expertise. The most effective literature reviews involve collaboration between AI and human researchers. AI handles repetitive tasks like data collection and initial screening, freeing up time for researchers to focus on critical analysis and interpretation.
Human oversight ensures that AI-generated results are accurate, and human expertise is still required to draw meaningful conclusions from the data. Researchers must remain actively involved in evaluating the relevance and quality of studies, even when AI tools are used.
5. Benefits of AI-Optimized Literature Reviews
The advantages of using AI in literature reviews are numerous:
Time Efficiency: AI tools reduce the time required for search, selection, and data extraction, allowing researchers to complete reviews faster.
Increased Accuracy: AI algorithms can process vast amounts of data with high precision, ensuring that no relevant studies are missed and reducing human error.
Consistency: AI tools apply consistent criteria for study selection, reducing the likelihood of bias or inconsistency in the review process.
Scalability: AI tools allow researchers to handle large datasets, which would be unmanageable manually, and scale their literature reviews to include more comprehensive data.
Continuous Updates: AI systems can automatically scan for new studies, ensuring that literature reviews remain current and comprehensive.
6. Conclusion
Optimizing literature reviews with AI is a game-changer for researchers, particularly in fields where vast amounts of data must be processed quickly and accurately. By automating the labor-intensive tasks of data collection, study selection, and information extraction, AI allows researchers to focus on critical analysis and synthesis, leading to more reliable and comprehensive reviews.
AI’s ability to reduce bias, ensure comprehensive coverage, and integrate with human expertise makes it an indispensable tool for modern literature reviews. As AI technologies continue to evolve, their role in enhancing research workflows will only grow, offering new possibilities for scientific discovery and knowledge synthesis.
Embracing AI in literature reviews is not just about efficiency—it's about improving the quality and reliability of research in an era of information overload. Researchers who incorporate AI into their workflows are better positioned to stay ahead in their fields and contribute meaningful insights to the broader scientific community.
Kommentare