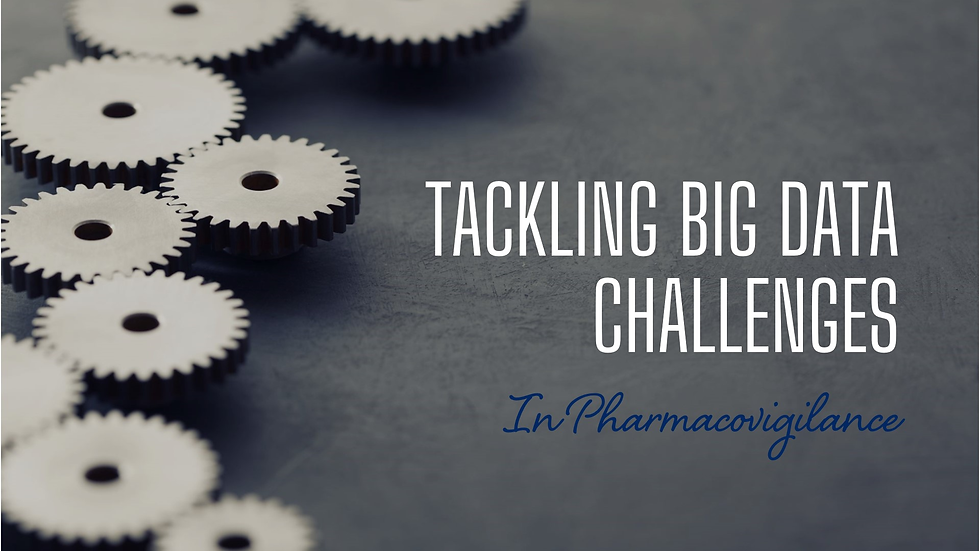
In the evolving landscape of healthcare, the integration of big data has transformed various fields, including pharmacovigilance. Pharmacovigilance, the science dedicated to the detection, assessment, understanding, and prevention of adverse effects or any other drug-related problems, relies heavily on data. The vast amounts of information generated from clinical trials, electronic health records, patient reports, and social media present both opportunities and challenges. While big data offers the potential for more accurate and timely drug safety monitoring, it also introduces complexities that must be managed effectively. This blog explores how to overcome the big data challenges in pharmacovigilance, highlighting strategies for managing data quality, integration, analysis, and security.
Understanding Big Data in Pharmacovigilance:
Big data in pharmacovigilance encompasses large volumes of diverse and complex data types, including structured data from clinical trials, unstructured data from patient reports, and real-world data from electronic health records and social media. The aim is to leverage this data to identify potential safety signals, assess the risk-benefit profile of drugs, and ensure patient safety. However, the sheer volume and variety of data present several challenges:
Data Quality and Completeness: Ensuring that the data is accurate, complete, and reliable is crucial for effective pharmacovigilance. Incomplete or erroneous data can lead to incorrect conclusions about a drug’s safety profile.
Data Integration: Combining data from various sources, such as clinical trials, health records, and social media, into a cohesive and usable format is complex. Data often comes in different formats and standards, making integration challenging.
Data Analysis: Analyzing large datasets requires advanced tools and techniques. Traditional methods may not be sufficient for handling and interpreting big data, necessitating the use of sophisticated analytics and machine learning algorithms.
Data Security and Privacy: Protecting sensitive patient information while complying with regulatory requirements is a significant concern. Ensuring data security and privacy is essential for maintaining trust and complying with legal standards.
Regulatory Compliance: Adhering to regulatory requirements for data reporting, safety monitoring, and signal detection is essential. Ensuring compliance with regulations across different jurisdictions can be challenging.
Strategies for Overcoming Big Data Challenges:
1. Ensuring Data Quality and Completeness
Data quality is the foundation of effective pharmacovigilance. To overcome challenges related to data quality and completeness:
Implement Robust Data Validation Processes: Employ validation techniques to ensure data accuracy and completeness. Automated data validation tools can help identify and correct errors or inconsistencies in real time.
Standardize Data Collection: Use standardized data collection methods and formats to ensure consistency. Adopting industry standards, such as those provided by the International Council for Harmonization (ICH) and the Common Data Model (CDM), can facilitate data integration and analysis.
Regular Data Audits: Conduct regular audits to assess data quality and address any issues promptly. Data audits help identify gaps or discrepancies and ensure that data meets the required standards.
Training and Guidelines: Provide training for data collectors and analysts to ensure they understand best practices for data entry and reporting. Clear guidelines can help minimize errors and improve data quality.
2. Integrating Data from Diverse Sources
Effective data integration is crucial for obtaining a comprehensive view of drug safety. To address integration challenges:
Adopt Data Integration Platforms: Utilize data integration platforms that can handle diverse data types and formats. These platforms can facilitate the consolidation of data from clinical trials, electronic health records, social media, and other sources.
Use Data Interoperability Standards: Implement standards for data interoperability, such as Fast Healthcare Interoperability Resources (FHIR) and Health Level Seven International (HL7). These standards enable different systems to communicate and exchange data effectively.
Develop Data Integration Frameworks: Create frameworks for integrating data that outline procedures for combining, normalizing, and transforming data. These frameworks should address data mapping, standardization, and harmonization.
Leverage Data Warehousing Solutions: Employ data warehousing solutions to store and manage large volumes of integrated data. Data warehouses can provide a centralized repository for data, making it easier to access and analyze.
3. Utilizing Advanced Data Analysis Techniques
Advanced data analysis techniques are essential for extracting meaningful insights from big data. To enhance data analysis:
Implement Machine Learning and AI: Use machine learning and artificial intelligence (AI) algorithms to analyze large datasets and identify patterns or trends. These technologies can enhance signal detection, risk assessment, and predictive modeling.
Adopt Data Visualization Tools: Utilize data visualization tools to present complex data in an understandable and actionable format. Visualization techniques, such as dashboards and heatmaps, can help identify trends and facilitate decision-making.
Employ Natural Language Processing (NLP): Implement NLP techniques to analyze unstructured data from sources like social media and patient reports. NLP can extract relevant information, identify safety signals, and improve data analysis.
Optimize Data Processing: Invest in high-performance computing resources to handle large-scale data processing. Scalable cloud computing solutions can provide the necessary infrastructure for analyzing big data efficiently.
4. Ensuring Data Security and Privacy
Data security and privacy are paramount in pharmacovigilance. To address these concerns:
Implement Data Encryption: Use encryption methods to protect sensitive patient data during storage and transmission. Encryption ensures that data is secure and accessible only to authorized individuals.
Adopt Privacy-Enhancing Technologies: Utilize privacy-enhancing technologies, such as anonymization and pseudonymization, to protect patient identities while analyzing data. These techniques reduce the risk of data breaches and maintain privacy.
Ensure Compliance with Regulations: Adhere to data protection regulations, such as the General Data Protection Regulation (GDPR) and the Health Insurance Portability and Accountability Act (HIPAA). Ensure that data handling practices comply with legal requirements and industry standards.
Conduct Security Audits: Regularly perform security audits to assess the effectiveness of data protection measures. Security audits help identify vulnerabilities and ensure that data protection practices are up to date.
5. Maintaining Regulatory Compliance
Regulatory compliance is essential for effective pharmacovigilance. To ensure compliance:
Stay Informed about Regulatory Changes: Keep up to date with changes in regulations and guidelines related to pharmacovigilance. Regularly review and update procedures to ensure compliance with current requirements.
Implement Compliance Management Systems: Use compliance management systems to track and manage regulatory requirements. These systems can help ensure that all aspects of pharmacovigilance are in line with regulatory standards.
Collaborate with Regulatory Authorities: Establish and maintain communication with regulatory authorities to stay informed about compliance expectations. Collaborative relationships can help address regulatory challenges and ensure adherence to guidelines.
Conduct Regular Training: Provide ongoing training for staff on regulatory requirements and best practices for pharmacovigilance. Training ensures that all team members are aware of their responsibilities and compliant with regulations.
Case Studies and Examples:
1. Case Study: Leveraging AI for Signal Detection
A leading pharmaceutical company implemented AI-driven analytics to enhance signal detection in their pharmacovigilance processes. By using machine learning algorithms to analyze large volumes of adverse event data, the company was able to identify safety signals more quickly and accurately. The AI system was trained to recognize patterns and trends that might indicate potential safety concerns, allowing for timely interventions and risk mitigation.
2. Example: Integration of Electronic Health Records (EHRs)
An integrated healthcare system used data warehousing solutions to combine data from electronic health records (EHRs), clinical trials, and patient-reported outcomes. By creating a centralized data repository, the organization was able to analyze comprehensive patient data and improve drug safety monitoring. The integrated system facilitated better risk assessment and enabled more effective response to emerging safety issues.
3. Case Study: Enhancing Data Privacy with Blockchain
A pharmacovigilance organization adopted blockchain technology to enhance data privacy and security. By using blockchain for data storage and transmission, the organization ensured that patient data was encrypted and immutable. The blockchain system provided a secure and transparent method for handling sensitive information, addressing privacy concerns and maintaining compliance with data protection regulations.
The Future of Big Data in Pharmacovigilance:
The future of big data in pharmacovigilance is promising, with ongoing advancements in technology and data analytics expected to further enhance drug safety monitoring. Emerging trends include:
Increased Use of AI and Machine Learning: AI and machine learning will continue to play a crucial role in analyzing big data and identifying safety signals. Advanced algorithms and predictive modeling will enable more accurate risk assessment and proactive safety monitoring.
Integration of Wearable Technology: Wearable devices will provide real-time data on patient health, contributing to more comprehensive pharmacovigilance. Integration of wearable data with other sources will enhance the ability to monitor drug safety and detect adverse effects.
Expansion of Real-World Evidence: Real-world evidence generated from diverse data sources will become increasingly important for evaluating drug safety. The integration of big data from various sources will provide a more complete picture of drug safety and effectiveness.
Advancements in Data Privacy Technologies: Innovations in data privacy technologies, such as advanced encryption and decentralized data storage, will address privacy concerns and ensure the secure handling of sensitive patient information.
Conclusion:
Overcoming big data challenges in pharmacovigilance requires a multifaceted approach that addresses data quality, integration, analysis, security, and regulatory compliance. By implementing robust data validation processes, adopting advanced analytics techniques, ensuring data security, and maintaining regulatory adherence, organizations can effectively leverage big data to enhance drug safety monitoring. As technology continues to evolve, the integration of AI, wearable technology, and real-world evidence will further advance pharmacovigilance practices, leading to improved patient safety and more effective drug development. The future of pharmacovigilance lies in harnessing the power of big data to identify and address safety concerns proactively, ultimately ensuring better outcomes for patients and more reliable therapeutic interventions
Comments