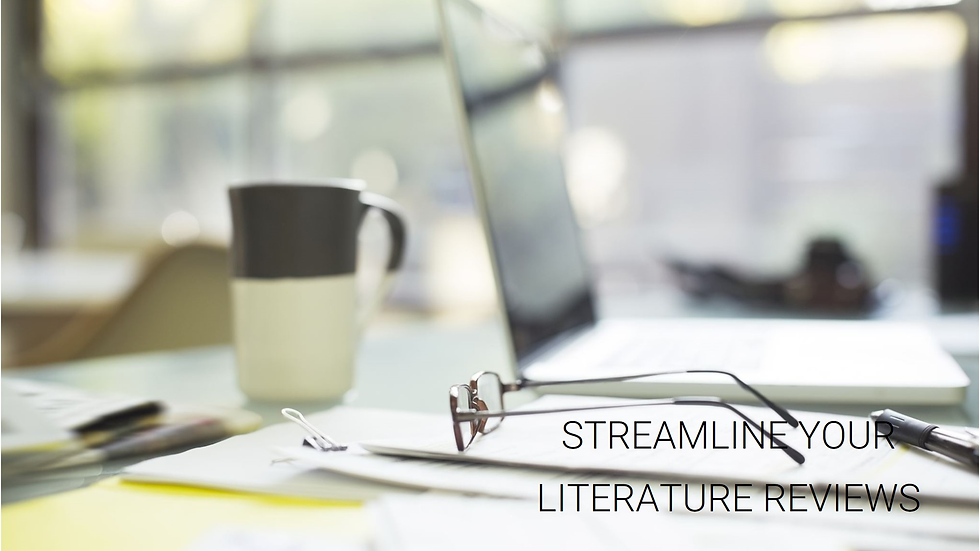
Conducting a literature review is a fundamental step in the research process, serving as the foundation for any academic or scientific inquiry. Traditionally, literature reviews have been time-consuming and labor-intensive, requiring researchers to sift through vast amounts of information to identify relevant studies, synthesize findings, and highlight gaps in the existing body of knowledge. However, recent advancements in artificial intelligence (AI) and machine learning (ML) are revolutionizing how literature reviews are conducted, making the process more efficient, accurate, and less overwhelming. This blog will explore how AI and ML can simplify literature review processes and enhance research outcomes.
Understanding Literature Reviews:
A literature review is a comprehensive survey of existing research on a specific topic or research question. It involves collecting, evaluating, and synthesizing relevant literature to provide a coherent understanding of what is already known, identify gaps in knowledge, and justify the need for further research.
The literature review process typically involves several steps:
Defining the Research Question: Clearly articulating the research question or hypothesis to guide the review.
Conducting a Comprehensive Search: Identifying relevant databases and sources to gather literature, including journal articles, books, conference papers, and reports.
Screening and Selecting Studies: Evaluating the gathered literature based on inclusion and exclusion criteria to identify studies that are most relevant to the research question.
Analyzing and Synthesizing Findings: Extracting key findings, themes, and methodologies from the selected studies to create a cohesive narrative.
Identifying Gaps and Future Directions: Highlighting areas where further research is needed to inform future studies.
The Challenges of Traditional Literature Review Processes
While literature reviews are essential for building a solid foundation for research, they can be fraught with challenges, including:
Time-Consuming: Manually searching, screening, and analyzing literature can take weeks or even months, delaying the overall research process.
Information Overload: The sheer volume of published studies can be overwhelming, making it difficult to identify relevant research and synthesize findings.
Subjectivity: The selection and evaluation of studies can be influenced by researcher bias, leading to potential gaps in the review.
Lack of Standardization: Different fields may have varying conventions for conducting literature reviews, leading to inconsistencies in methodology and reporting.
How AI and ML Can Simplify Literature Review Processes
AI and ML technologies can streamline literature review processes in several ways, addressing the challenges outlined above and enhancing research efficiency and effectiveness.
1. Automated Literature Search
AI-powered tools can automate the literature search process, quickly scanning vast databases to identify relevant studies based on predefined keywords, phrases, and research questions. These tools use natural language processing (NLP) algorithms to understand the context of search queries, making it easier to retrieve pertinent literature.
For example, AI algorithms can identify synonyms, related terms, and variations of search terms, expanding the scope of literature retrieval and ensuring that no relevant studies are overlooked. This automation significantly reduces the time researchers spend searching for literature and allows them to focus on analysis and synthesis.
2. Efficient Screening and Selection
Once the literature has been gathered, researchers must screen and select studies based on inclusion and exclusion criteria. AI and ML technologies can assist in this phase by employing algorithms that assess the relevance and quality of studies automatically.
Machine learning models can be trained to recognize patterns in relevant literature, helping researchers quickly identify studies that meet specific criteria. For instance, these algorithms can analyze abstracts, titles, and keywords to determine the likelihood of relevance, allowing researchers to prioritize studies that align with their research question.
Furthermore, AI tools can facilitate the screening of studies for quality and rigor, reducing the risk of including low-quality research in the review. This automated screening process saves time and ensures a more systematic approach to literature selection.
3. Data Extraction and Analysis
AI and ML can enhance data extraction and analysis by automating the process of identifying key findings, themes, and methodologies within selected studies. Natural language processing algorithms can analyze text, extract relevant information, and categorize findings based on predefined themes.
For example, AI tools can be used to identify the primary outcomes, sample sizes, methodologies, and conclusions of studies, allowing researchers to synthesize information more efficiently. This automation reduces the risk of human error and enables researchers to generate insights from literature quickly.
Moreover, machine learning algorithms can assist in identifying trends and patterns within the literature, helping researchers uncover connections between studies and explore new research directions.
4. Improved Synthesis and Visualization
Synthesizing findings from multiple studies can be a complex and challenging task. AI and ML technologies can facilitate this process by providing tools for data visualization and analysis. These tools can help researchers create visual representations of the literature, such as charts, graphs, and network diagrams, that illustrate key findings and relationships.
For example, machine learning algorithms can analyze citation networks to identify influential studies, emerging trends, and potential gaps in the literature. These visualizations can help researchers communicate their findings effectively and provide a clearer understanding of the state of the field.
Additionally, AI-powered platforms can assist in generating automated summaries of literature, allowing researchers to quickly grasp the key points of multiple studies without having to read each one in detail.
5. Identification of Research Gaps
AI and ML can help researchers identify gaps in the existing literature by analyzing patterns in published studies and highlighting areas where further research is needed. By examining citation patterns, co-authorship networks, and thematic trends, machine learning algorithms can uncover under-researched topics and suggest potential avenues for future investigation.
This capability is particularly valuable for researchers looking to contribute new knowledge to their field. By leveraging AI tools, researchers can ensure that their work addresses relevant and timely research questions, ultimately advancing the state of knowledge in their discipline.
6. Streamlining Collaboration
Collaboration is often a key component of the research process, and AI tools can facilitate teamwork among researchers. Many AI-powered literature review platforms offer collaborative features that allow multiple users to work together on a literature review in real time.
Researchers can share notes, highlight key findings, and discuss studies within the platform, streamlining communication and ensuring that all team members are on the same page. This collaborative approach enhances the overall quality of the literature review by incorporating diverse perspectives and expertise.
Case Studies: AI and ML in Action:
Several research institutions and organizations have already begun to integrate AI and ML technologies into their literature review processes. Here are a few case studies illustrating the successful application of these technologies:
Case Study 1: The COVID-19 Evidence Synthesis
During the COVID-19 pandemic, researchers needed to rapidly synthesize evidence from a vast number of studies on the virus, its transmission, and treatments. AI-powered platforms were developed to automate the literature review process, allowing researchers to identify relevant studies in real time and synthesize findings quickly.
These platforms utilized natural language processing to extract key data points from articles and generate automated summaries, enabling researchers to make informed decisions based on the latest evidence. This rapid synthesis of information was crucial for informing public health policies and clinical guidelines during the pandemic.
Case Study 2: Systematic Reviews in Healthcare
A research team conducted a systematic review of interventions to improve patient adherence to medication. To streamline the literature review process, they employed AI-powered tools that automated the search and screening of relevant studies.
The AI algorithms analyzed thousands of articles, identifying studies that met the inclusion criteria and extracting relevant data points. This automation significantly reduced the time spent on the literature review, allowing the researchers to focus on synthesizing findings and drawing meaningful conclusions.
Best Practices for Implementing AI and ML in Literature Reviews:
To maximize the benefits of AI and ML in literature reviews, researchers should consider the following best practices:
Define Clear Research Questions: Clearly articulate the research questions or hypotheses to guide the AI algorithms in literature retrieval and analysis.
Select Appropriate AI Tools: Choose AI-powered platforms that align with the specific needs of the literature review, considering features such as automated search, data extraction, and visualization.
Combine Human Expertise with AI: While AI can significantly enhance the literature review process, it should complement, not replace, human expertise. Researchers should remain actively involved in critical evaluation and synthesis.
Train AI Models: If using machine learning algorithms, ensure that models are trained on relevant datasets to improve accuracy and effectiveness in identifying and analyzing literature.
Maintain Ethical Considerations: Be aware of ethical considerations related to data privacy, transparency, and potential biases in AI algorithms. Ensure that AI tools are used responsibly and ethically in the research process.
Stay Updated on Advances: The field of AI and ML is rapidly evolving. Researchers should stay informed about the latest advancements and tools available for literature review processes.
Conclusion:
AI and ML are revolutionizing the literature review process, making it more efficient, accurate, and accessible for researchers across disciplines. By automating literature searches, screening and selection, data extraction, synthesis, and gap identification, these technologies are transforming the way researchers conduct literature reviews.
As the volume of research continues to grow, the integration of AI and ML into literature review processes will become increasingly essential. By leveraging these technologies, researchers can streamline their workflows, enhance collaboration, and ultimately contribute to the advancement of knowledge in their fields.
As researchers embrace AI and ML tools, they will not only simplify their literature review processes but also improve the quality of their research outcomes, paving the way for more impactful and relevant studies in the future.
Comments