How to Use AI and ML for Effective Literature Monitoring
- Chaitali Gaikwad
- Oct 15, 2024
- 5 min read
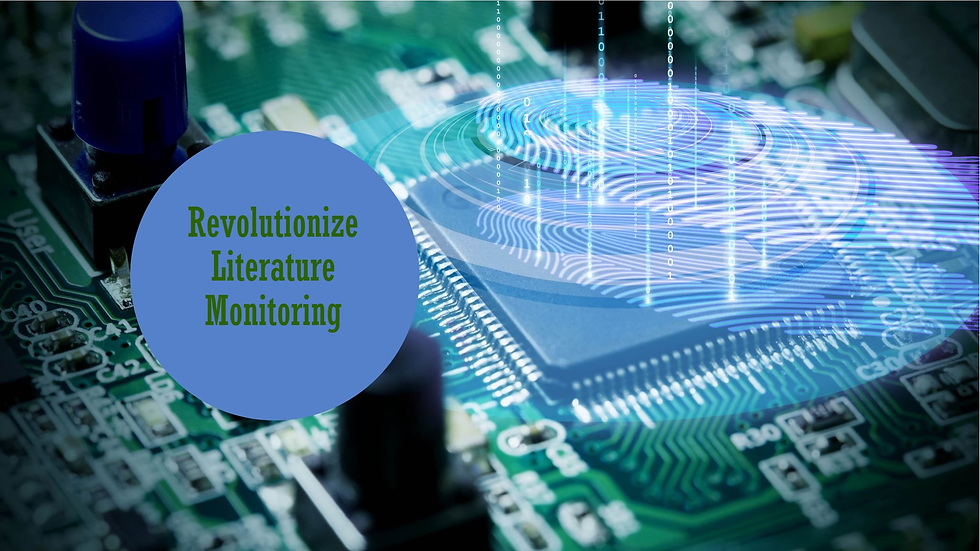
In today’s fast-paced world, the volume of scientific literature is expanding at an unprecedented rate. For organizations, especially in the fields of healthcare, pharmaceuticals, and research, keeping up with this vast influx of information is both a challenge and a necessity. Effective literature monitoring is crucial for ensuring compliance, enhancing knowledge management, and improving decision-making. Traditional methods of literature review can be time-consuming and prone to human error, leading to missed information or delays in critical findings. This is where Artificial Intelligence (AI) and Machine Learning (ML) come into play, revolutionizing the way literature monitoring is approached.
Understanding Literature Monitoring
Literature monitoring involves systematically tracking and analyzing published research, studies, articles, and other relevant materials. The goal is to identify important trends, advancements, and potential risks associated with various topics, especially in fields like pharmacovigilance, clinical research, and public health.
Key components of literature monitoring include:
Data Collection: Gathering information from various sources, including academic journals, medical databases, and conference proceedings.
Data Analysis: Evaluating the collected data to identify patterns, trends, and insights.
Reporting: Summarizing the findings for stakeholders to inform decision-making processes.
The Role of AI and ML in Literature Monitoring
AI and ML technologies can significantly enhance literature monitoring by automating data collection, improving analysis accuracy, and providing actionable insights. Here’s how organizations can leverage these technologies effectively:
1. Automating Data Collection
One of the most time-consuming aspects of literature monitoring is the manual collection of relevant studies and articles. AI can streamline this process through automated data scraping and content aggregation.
Web Scraping: AI algorithms can be trained to scrape data from various online sources, including research databases, journals, and news articles. This process involves using Natural Language Processing (NLP) techniques to identify relevant content based on predefined keywords and topics.
Content Aggregation: AI tools can aggregate content from multiple sources, providing a centralized repository for easy access. By utilizing APIs from academic databases like PubMed, Scopus, or Web of Science, organizations can ensure they have the latest studies at their fingertips.
2. Enhanced Search Capabilities
Traditional search methods often rely on keyword matching, which may miss relevant articles that use different terminologies or phrasing. AI and ML can enhance search capabilities through:
Semantic Search: Unlike traditional keyword searches, semantic search uses contextual understanding to identify relevant content. AI algorithms can interpret the meaning behind queries, returning results that are semantically related even if the exact keywords are not present.
Topic Modeling: Machine learning techniques can be employed to analyze vast amounts of literature and identify key topics and themes. By clustering similar documents together, organizations can gain a clearer understanding of emerging trends in their field.
3. Automated Abstracting and Summarization
Reading through hundreds of articles to identify relevant information is a daunting task. AI can assist with this through:
Automated Abstract Generation: AI models can be trained to generate concise abstracts summarizing the key findings of research articles. This allows researchers to quickly assess whether an article is relevant to their needs.
Content Summarization: Advanced ML algorithms can provide summaries of entire documents, highlighting essential points and findings. This capability saves time and ensures that no critical information is overlooked.
4. Risk Identification and Signal Detection
In fields such as pharmacovigilance, identifying potential risks associated with drugs or treatments is paramount. AI and ML can enhance this process by:
Adverse Event Detection: Machine learning algorithms can analyze literature for reports of adverse events or side effects associated with specific drugs. By cross-referencing this information with existing databases, organizations can detect potential safety signals early.
Trend Analysis: ML models can be used to analyze historical data and identify trends in adverse events over time. This proactive approach enables organizations to address safety concerns before they escalate.
5. Predictive Analytics
AI and ML can also play a crucial role in predictive analytics, helping organizations forecast future trends and potential issues based on existing data.
Predictive Modeling: By training machine learning models on historical literature data, organizations can predict potential risks or trends. For instance, if a particular drug has been linked to adverse events in recent studies, predictive models can assess the likelihood of similar reports emerging in the future.
Scenario Analysis: Organizations can use AI to run “what-if” scenarios based on literature findings. This capability allows stakeholders to evaluate potential outcomes and make informed decisions regarding risk management and product development.
6. Improved Collaboration and Knowledge Sharing
AI and ML facilitate better collaboration and knowledge sharing among teams.
Knowledge Management Systems: AI-powered systems can organize and categorize literature findings, making it easy for team members to access relevant information. This organization enhances collaboration by ensuring everyone is on the same page regarding current research.
Collaborative Tools: AI-driven platforms can support collaboration among researchers by allowing them to share insights, annotations, and findings in real time. This fosters a culture of continuous learning and innovation within organizations.
7. Tailored Reporting and Visualization
The ability to visualize data is crucial for effective decision-making. AI can enhance reporting through:
Data Visualization: Machine learning algorithms can generate intuitive visual representations of literature data, such as charts, graphs, and dashboards. These visualizations help stakeholders quickly grasp trends and insights.
Customizable Reports: AI can automate the generation of tailored reports based on user preferences. By allowing stakeholders to specify the type of information they need, organizations can ensure they receive relevant updates without sifting through irrelevant data.
Best Practices for Implementing AI and ML in Literature Monitoring
To maximize the benefits of AI and ML for literature monitoring, organizations should consider the following best practices:
Define Clear Objectives: Establish clear goals for literature monitoring. Determine what specific insights or data are most valuable to your organization.
Invest in Quality Data: The effectiveness of AI and ML relies heavily on the quality of the data used for training. Ensure that your data sources are credible and up-to-date.
Choose the Right Tools: Evaluate various AI and ML tools available in the market. Select platforms that align with your organization's needs and integrate seamlessly with existing systems.
Train Your Team: Provide training for your team on using AI and ML tools effectively. Empower them to leverage these technologies for enhanced literature monitoring.
Continuously Evaluate and Optimize: Regularly assess the performance of your AI and ML systems. Continuously refine your algorithms and processes to ensure they meet evolving needs.
Ensure Compliance: In regulated industries, it’s essential to ensure that your literature monitoring practices comply with relevant regulations and guidelines. AI tools should be implemented in a manner that adheres to these standards.
Conclusion:
The integration of AI and ML into literature monitoring represents a significant advancement in how organizations manage information. By automating data collection, enhancing analysis, and providing actionable insights, these technologies empower businesses to stay ahead in an ever-changing landscape. As the volume of scientific literature continues to grow, embracing AI and ML will be essential for organizations looking to maintain compliance, enhance knowledge management, and ultimately improve patient safety and outcomes.
In a world where information is power, leveraging AI and ML for effective literature monitoring is not just an option—it’s a necessity. Organizations that embrace these technologies will not only streamline their processes but also position themselves as leaders in their fields, driving innovation and ensuring the highest standards of safety and efficacy in their products and services.
Comments