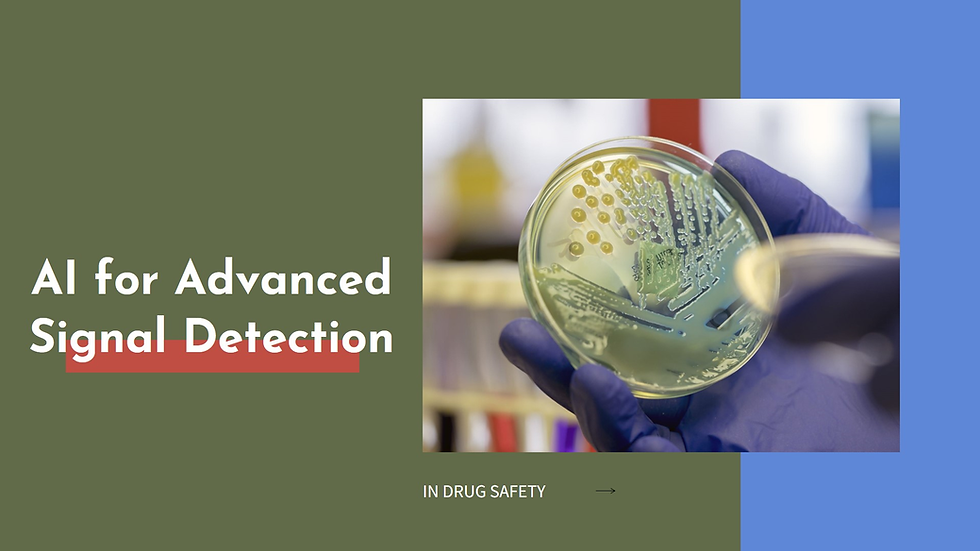
The pharmaceutical industry is undergoing a transformative shift driven by advancements in artificial intelligence (AI). One of the most significant areas where AI is making an impact is in drug safety, specifically in signal detection. Signal detection is a critical process in pharmacovigilance, where data is analyzed to identify potential safety issues associated with pharmaceutical products. Traditional methods of signal detection have limitations, including the time it takes to identify safety concerns and the potential for human error. AI, with its ability to process vast amounts of data quickly and accurately, offers a solution to these challenges. In this blog, we'll explore how AI can be used for advanced signal detection in drug safety, the benefits it brings, and practical steps for implementing AI-driven signal detection in pharmacovigilance.
Understanding Signal Detection in Drug Safety
Signal detection in drug safety involves the identification of new or unexpected adverse events associated with pharmaceutical products. These signals are derived from various data sources, including:
Spontaneous Reporting Systems: Voluntary reports of adverse drug reactions (ADRs) submitted by healthcare professionals, patients, and pharmaceutical companies.
Electronic Health Records (EHRs): Comprehensive patient data, including medication history, diagnoses, and clinical outcomes.
Clinical Trials: Data from controlled studies that provide initial safety information about a drug.
Medical Literature: Published studies and case reports that may reveal new safety concerns.
Social Media and Online Forums: User-generated content that can offer insights into patient experiences and potential ADRs.
The goal of signal detection is to identify potential safety issues as early as possible, allowing for timely interventions to protect patient safety. Traditional methods of signal detection, such as disproportionality analysis and Bayesian data mining, have been effective but are limited by their reliance on predefined thresholds and manual analysis. AI has the potential to revolutionize signal detection by automating and enhancing these processes.
The Role of AI in Advanced Signal Detection
AI encompasses a range of technologies, including machine learning (ML), natural language processing (NLP), and data mining, all of which can be applied to signal detection in drug safety. These technologies enable more sophisticated analysis of data, allowing for the identification of patterns and correlations that may not be apparent through traditional methods.
Key AI Technologies for Signal Detection
Machine Learning (ML)
ML algorithms are designed to learn from data and improve their performance over time. In the context of signal detection, ML can be used to:
Analyze Large Datasets: ML can process vast amounts of data from multiple sources, identifying patterns and correlations that may indicate potential safety signals.
Predict ADRs: Predictive models can be trained on historical data to forecast the likelihood of ADRs occurring in specific patient populations.
Automate Signal Detection: ML algorithms can automate the detection of signals, reducing the reliance on manual analysis and speeding up the process.
Natural Language Processing (NLP)
NLP is a branch of AI that focuses on the interaction between computers and human language. It enables the analysis of unstructured text data, such as medical records and social media posts. In signal detection, NLP can be used to:
Extract Relevant Information: NLP algorithms can extract key information from unstructured text, such as mentions of ADRs, patient symptoms, and drug names.
Analyze Medical Literature: NLP can automate the review of medical literature, identifying new safety signals and trends in published studies.
Monitor Social Media: NLP tools can scan social media platforms for patient-reported outcomes and experiences, providing real-time insights into potential safety concerns.
Data Mining
Data mining involves the exploration and analysis of large datasets to uncover hidden patterns and relationships. In pharmacovigilance, data mining techniques can be used to:
Identify Unusual Patterns: Data mining can detect unusual patterns in ADR reporting, which may indicate new or emerging safety signals.
Combine Data Sources: Data mining allows for the integration of data from multiple sources, providing a more comprehensive view of drug safety.
Support Regulatory Decision-Making: Data mining results can inform regulatory decisions, such as labeling changes or the issuance of safety warnings.
Benefits of Using AI for Signal Detection in Drug Safety:
The application of AI in signal detection offers several key benefits that enhance the overall effectiveness of pharmacovigilance efforts:
Improved Accuracy and Sensitivity
AI algorithms can detect subtle patterns and correlations in data that may be missed by traditional methods. This leads to improved accuracy in identifying true safety signals while reducing the number of false positives. As a result, AI-driven signal detection is more sensitive to potential risks, allowing for earlier identification and intervention.
Enhanced Efficiency
Traditional signal detection methods often involve time-consuming manual analysis. AI automates many of these processes, significantly reducing the time required to detect and evaluate signals. This increased efficiency allows pharmacovigilance teams to focus on higher-value tasks, such as risk assessment and mitigation.
Real-Time Monitoring
AI enables real-time monitoring of various data sources, including EHRs, social media, and spontaneous reporting systems. This real-time capability allows for the continuous assessment of drug safety, enabling quicker responses to emerging risks and improving overall patient safety.
Scalability
As the volume of pharmacovigilance data continues to grow, AI provides the scalability needed to manage and analyze large datasets effectively. Whether dealing with thousands of ADR reports or millions of social media posts, AI can handle the scale and complexity of modern drug safety data.
Proactive Risk Management
AI's predictive capabilities allow for proactive risk management. By identifying potential safety issues before they become widespread, pharmaceutical companies and regulatory agencies can implement preventive measures, such as additional studies or risk communication, to mitigate risks.
Practical Steps for Implementing AI in Signal Detection:
Implementing AI for advanced signal detection in drug safety requires careful planning and execution. Here are some practical steps to guide the process:
Assess Data Readiness
Before implementing AI, organizations must assess the readiness of their data. This includes evaluating the quality, completeness, and diversity of data sources. High-quality data is essential for training AI algorithms and ensuring accurate signal detection.
Data Cleaning: Clean and standardize data to remove errors, duplicates, and inconsistencies.
Data Integration: Integrate data from various sources, such as EHRs, spontaneous reporting systems, and medical literature, to create a comprehensive dataset.
Select the Right AI Tools
Choosing the right AI tools and technologies is critical for successful implementation. Organizations should consider their specific needs, available resources, and the types of data they will be analyzing.
AI Platforms: Explore AI platforms that offer ML, NLP, and data mining capabilities tailored to pharmacovigilance.
Customization: Ensure that AI tools can be customized to fit the unique requirements of your signal detection processes.
Develop and Train AI Models
Once the appropriate AI tools have been selected, the next step is to develop and train AI models for signal detection.
Model Development: Collaborate with data scientists and pharmacovigilance experts to develop models that are aligned with the organization's goals.
Training Data: Use historical data on ADRs and drug safety to train AI models, allowing them to learn from past patterns and outcomes.
Continuous Improvement: Implement processes for continuous model improvement, allowing AI algorithms to adapt and evolve as new data becomes available.
Integrate AI with Existing Systems
AI should be integrated with existing pharmacovigilance systems and workflows to maximize its effectiveness.
System Integration: Ensure that AI tools are compatible with existing databases, reporting systems, and analysis platforms.
Workflow Alignment: Align AI-driven signal detection with existing workflows, allowing for seamless adoption by pharmacovigilance teams.
Monitor and Validate AI Performance
Monitoring and validating the performance of AI models is essential to ensure their accuracy and reliability in signal detection.
Performance Metrics: Establish metrics to evaluate the performance of AI models, such as accuracy, sensitivity, and specificity.
Regular Audits: Conduct regular audits of AI-driven signal detection processes to identify any potential issues and ensure compliance with regulatory standards.
Ensure Regulatory Compliance
AI-driven signal detection must comply with regulatory requirements and guidelines set forth by agencies such as the FDA, EMA, and other global regulatory bodies.
Documentation: Maintain thorough documentation of AI models, including their development, training, and validation processes.
Regulatory Reporting: Ensure that AI-generated signals are reported in accordance with regulatory guidelines, including proper documentation of the decision-making process.
Invest in Training and Change Management
Successful implementation of AI in signal detection requires investment in training and change management to ensure that all stakeholders are comfortable with the new technology.
Training Programs: Develop training programs to educate pharmacovigilance teams on the use of AI tools and the interpretation of AI-generated signals.
Change Management: Implement change management strategies to address any resistance to adopting AI-driven processes and to promote a culture of innovation.
The Future of AI in Drug Safety Signal Detection:
The future of AI in drug safety signal detection holds exciting possibilities, with ongoing advancements in technology and data science poised to further enhance pharmacovigilance efforts. Here are some emerging trends to watch:
Integration of Real-World Data (RWD)
AI will increasingly leverage real-world data from sources such as EHRs, patient registries, and wearable devices to provide a more comprehensive view of drug safety. This integration will enable more accurate and timely detection of safety signals in real-world settings.
Collaborative AI Models
Collaborative AI models that combine data and insights from multiple stakeholders, including pharmaceutical companies, regulatory agencies, and healthcare providers, will enhance the robustness of signal detection efforts. These models will facilitate the sharing of knowledge and resources, leading to more effective drug safety monitoring.
AI-Driven Predictive Analytics
Predictive analytics powered by AI will play a larger role in drug safety, enabling the identification of potential safety issues before they manifest. By predicting adverse events based on historical data and patient characteristics, AI will support proactive risk management and preventive measures.
Ethical AI and Transparency
As AI becomes more integral to drug safety, there will be an increased focus on ethical AI practices and transparency. Organizations will need to ensure that AI models are unbiased, transparent, and explainable, allowing stakeholders to trust and understand AI-driven decisions.
Conclusion:
AI has the potential to revolutionize signal detection in drug safety by enhancing accuracy, efficiency, and scalability. By leveraging machine learning, natural language processing, and data mining, AI can provide more comprehensive and timely insights into drug safety, ultimately improving patient outcomes and regulatory compliance. However, successful implementation requires careful planning, data readiness, and collaboration among stakeholders. As the pharmaceutical industry continues to embrace AI, the future of signal detection in drug safety looks promising, with AI-driven innovations paving the way for more effective and proactive pharmacovigilance.
Comments