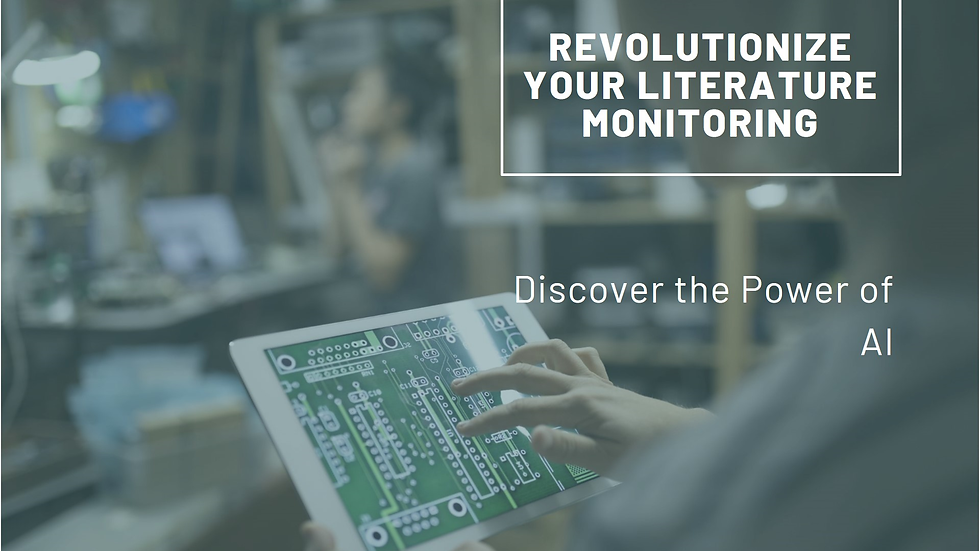
In an era where knowledge is expanding exponentially, staying updated with the latest research and information is paramount for professionals in various fields, particularly in healthcare, pharmaceuticals, and academia. Literature monitoring—the systematic identification and review of relevant research articles, clinical trials, and other scientific literature—plays a vital role in ensuring that organizations make informed decisions. With the advent of artificial intelligence (AI), the landscape of literature monitoring is undergoing a significant transformation. This blog will explore how to effectively use AI for comprehensive literature monitoring, its benefits, challenges, and best practices for implementation.
Understanding Literature Monitoring
Literature monitoring involves the continuous tracking of published research to identify relevant findings, trends, and emerging topics in a particular domain. In industries such as pharmaceuticals, this process is crucial for:
Regulatory Compliance: Ensuring that organizations adhere to industry regulations and guidelines.
Drug Safety: Monitoring for adverse events and safety signals associated with medications and medical devices.
Competitive Intelligence: Keeping abreast of competitors’ research and innovations.
Clinical Development: Supporting the design and execution of clinical trials by providing relevant background literature.
However, traditional literature monitoring methods can be labor-intensive, time-consuming, and prone to human error. This is where AI comes into play, offering innovative solutions to enhance efficiency and accuracy.
The Role of AI in Literature Monitoring
AI encompasses various technologies, including machine learning, natural language processing (NLP), and data mining, which can be leveraged to automate and optimize the literature monitoring process. Here’s how AI can be integrated into literature monitoring:
1. Automated Data Collection
AI can automate the process of gathering literature from various sources, including:
Academic Journals: Accessing databases such as PubMed, Scopus, and Web of Science to retrieve relevant articles.
Clinical Trial Registries: Monitoring ongoing and completed clinical trials for updates.
Preprint Servers: Tracking emerging research in real-time by accessing platforms like arXiv and bioRxiv.
By utilizing web scraping and API integrations, AI tools can continuously scan these sources and compile relevant data without manual intervention.
2. Enhanced Search Capabilities
Traditional keyword-based searches can yield a high volume of irrelevant results. AI-driven systems utilize NLP to understand the context of queries and improve search accuracy. Key features include:
Semantic Search: Instead of relying solely on keywords, AI systems can understand the intent behind a search query, leading to more relevant results.
Contextual Relevance: AI can prioritize articles based on their relevance to specific research questions or topics, allowing users to focus on the most pertinent literature.
3. Article Classification and Categorization
AI can automatically categorize and classify articles based on various criteria, such as:
Research Domain: Identifying the field of study (e.g., oncology, cardiology).
Type of Study: Differentiating between clinical trials, observational studies, systematic reviews, etc.
Relevance and Impact: Assessing the potential impact of findings based on citation metrics and publication reputation.
This classification helps researchers quickly locate literature that aligns with their interests and objectives.
4. Summarization and Insights Generation
AI can significantly reduce the time spent reading through extensive literature by generating summaries and extracting key insights from articles. This includes:
Abstract Extraction: Automatically extracting abstracts to provide a quick overview of the study’s objectives, methods, and conclusions.
Key Findings Highlighting: Identifying and highlighting critical findings, methodologies, and statistical analyses.
Trend Analysis: Analyzing patterns and trends in the literature over time, helping researchers identify emerging areas of interest.
5. Risk and Signal Detection
In fields like pharmacovigilance, AI plays a crucial role in detecting potential risks and safety signals associated with drugs and medical devices. By continuously monitoring the literature for adverse event reports and safety concerns, AI can:
Identify Patterns: Detect correlations between specific drugs and adverse events by analyzing a large volume of literature.
Generate Alerts: Provide real-time alerts when new safety signals are identified, enabling timely responses from regulatory bodies and organizations.
6. Integration with Existing Systems
AI tools can be seamlessly integrated into existing workflows and systems, enhancing literature monitoring without disrupting established processes. This includes:
Collaboration Platforms: Integrating AI with platforms such as Slack or Microsoft Teams to provide literature updates and insights.
Data Management Systems: Connecting AI tools to internal databases to enrich existing data with the latest research findings.
Benefits of Using AI for Literature Monitoring
Implementing AI in literature monitoring offers numerous advantages:
1. Increased Efficiency
AI-driven automation significantly reduces the time and effort required for literature monitoring, allowing researchers to focus on analyzing data and making informed decisions rather than spending hours searching for relevant articles.
2. Improved Accuracy
By utilizing NLP and advanced algorithms, AI enhances the accuracy of literature retrieval and classification, minimizing the risk of missing crucial information or being overwhelmed by irrelevant data.
3. Real-Time Updates
AI systems can provide real-time updates on newly published research, ensuring that organizations stay current with the latest findings and developments in their field.
4. Cost-Effectiveness
By streamlining the literature monitoring process, organizations can reduce the costs associated with manual monitoring, allowing resources to be allocated to other critical areas.
5. Enhanced Decision-Making
With access to comprehensive, up-to-date literature, organizations can make better-informed decisions regarding research directions, product development, and regulatory compliance.
Challenges in Implementing AI for Literature Monitoring
Despite its benefits, implementing AI in literature monitoring presents several challenges:
1. Data Quality and Consistency
AI relies on high-quality, consistent data to produce accurate results. Variations in publication formats, terminologies, and data sources can affect the performance of AI algorithms.
2. Integration Complexity
Integrating AI systems with existing workflows and databases can be complex, requiring technical expertise and resources to ensure seamless operation.
3. Ethical Considerations
The use of AI in literature monitoring raises ethical concerns related to data privacy, bias in algorithms, and the transparency of AI decision-making processes. Organizations must address these issues to build trust in AI systems.
4. Training and Adaptation
AI systems require continuous training and adaptation to stay relevant as research landscapes evolve. Organizations must invest time and resources in maintaining and updating AI tools.
Best Practices for Implementing AI in Literature Monitoring
To successfully implement AI for comprehensive literature monitoring, organizations should consider the following best practices:
1. Define Clear Objectives
Before implementing AI, organizations should define their objectives for literature monitoring. This includes identifying specific research questions, desired outcomes, and key performance indicators (KPIs) to measure success.
2. Choose the Right AI Tools
Selecting the appropriate AI tools and technologies is crucial for achieving desired outcomes. Organizations should evaluate various options based on factors such as:
Functionality: Assess whether the tool meets specific literature monitoring needs, such as automated data collection, NLP capabilities, and trend analysis.
User-Friendliness: Consider the ease of use and integration with existing workflows to ensure that team members can effectively utilize the tool.
Scalability: Ensure that the chosen AI solution can scale with the organization’s growth and evolving needs.
3. Prioritize Data Quality
Investing in data quality management is essential for the success of AI-driven literature monitoring. Organizations should implement processes to ensure that data collected from various sources is accurate, complete, and consistent.
4. Foster Collaboration and Communication
Encouraging collaboration between stakeholders involved in literature monitoring is vital for successful implementation. Regular communication ensures that team members are aligned and can provide feedback on AI tool performance.
5. Monitor and Evaluate Performance
Establishing metrics to monitor the performance of AI tools is crucial for continuous improvement. Organizations should regularly evaluate the effectiveness of their literature monitoring processes and make adjustments as needed.
6. Address Ethical Concerns
Organizations must address ethical considerations related to data privacy, algorithm bias, and transparency. This includes ensuring compliance with relevant regulations and maintaining an ethical framework for AI use.
7. Continuous Training and Development
AI technologies are rapidly evolving, and organizations must invest in ongoing training and development for their teams. Staying updated on the latest advancements in AI and literature monitoring techniques is essential for maintaining a competitive edge.
Conclusion:
The integration of AI into literature monitoring represents a significant advancement in how organizations track and analyze relevant research. By automating data collection, enhancing search capabilities, and providing real-time updates, AI can streamline the literature monitoring process and improve decision-making.
However, the successful implementation of AI requires careful planning, investment in data quality, and consideration of ethical concerns. By adopting best practices and continuously evaluating performance, organizations can leverage AI to enhance their literature monitoring efforts, ultimately leading to better research outcomes and improved public health.
Comments