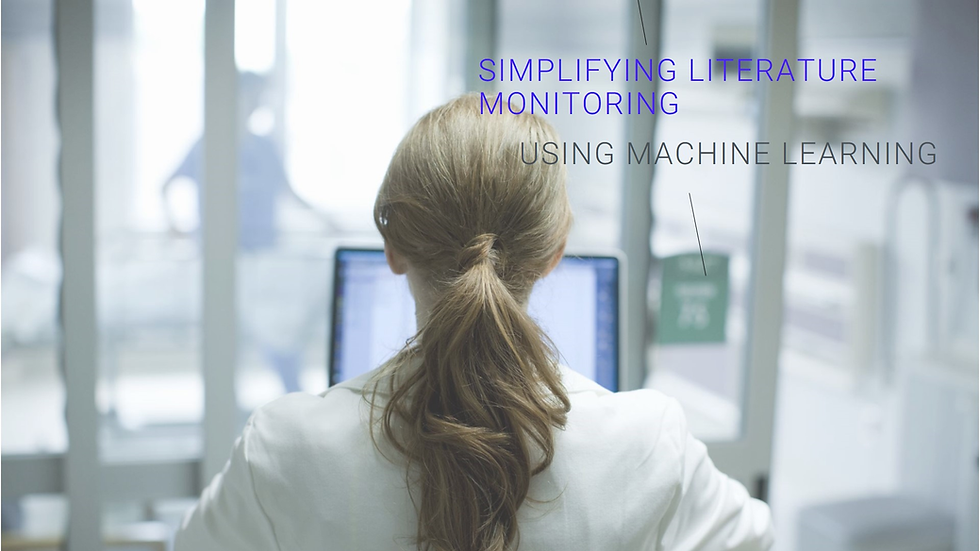
In the rapidly evolving landscape of research, staying updated with the latest literature is crucial for academics, healthcare professionals, and industry experts alike. The sheer volume of published articles, studies, and reports can be overwhelming, making it challenging for individuals to keep up with relevant findings. Traditional methods of literature monitoring—such as manual searches and setting up alerts—often fall short in efficiency and comprehensiveness. This is where Machine Learning (ML) steps in, offering innovative solutions that simplify and enhance the literature monitoring process. In this blog, we will explore how to effectively use machine learning to streamline literature monitoring, making it more efficient and effective.
1. Understanding Literature Monitoring
Literature monitoring refers to the systematic process of reviewing, evaluating, and synthesizing newly published research and information in a specific field. This practice is critical for various reasons:
1.1. Importance of Literature Monitoring
Informed Decision-Making: Keeping abreast of new findings helps researchers, clinicians, and decision-makers make informed choices in their work.
Identifying Trends: Literature monitoring enables the identification of emerging trends, gaps in research, and areas that require further exploration.
Enhancing Collaboration: Being aware of the latest research fosters collaboration among scholars and professionals, leading to more robust research outcomes.
1.2. Challenges in Traditional Literature Monitoring
Traditional methods of literature monitoring come with several challenges:
Time-Consuming: Manual searches can take significant time, leading to delays in accessing critical information.
Information Overload: The volume of new publications can result in researchers missing important studies or becoming overwhelmed by irrelevant content.
Inconsistent Quality: Not all published research is of high quality, making it essential for researchers to critically evaluate sources, which can be a daunting task.
2. The Role of Machine Learning in Literature Monitoring
Machine learning, a subset of artificial intelligence, involves the use of algorithms to analyze data, identify patterns, and make predictions. By applying ML techniques to literature monitoring, researchers can automate and optimize various aspects of the process. Here are some key benefits of using machine learning for literature monitoring:
2.1. Automated Literature Search
Machine learning algorithms can automate literature searches across multiple databases, enabling researchers to identify relevant articles quickly and efficiently.
Implementation: Tools powered by ML can scan platforms like PubMed, Scopus, and Google Scholar, retrieving relevant publications based on predefined criteria.
2.2. Natural Language Processing (NLP)
Natural Language Processing, a branch of machine learning, allows computers to understand, interpret, and respond to human language. NLP can be used to extract meaningful information from research papers, helping researchers to:
Summarize Articles: ML models can generate concise summaries of lengthy articles, providing researchers with essential insights without requiring them to read the entire text.
Identify Key Findings: NLP techniques can extract significant data points, findings, and conclusions from articles, streamlining the review process.
2.3. Relevance Ranking and Filtering
Machine learning algorithms can analyze literature and rank articles based on their relevance to specific research queries. This ensures that researchers receive the most pertinent information, eliminating the need to sift through irrelevant studies.
Personalized Recommendations: Machine learning systems can learn from user preferences and behavior, offering tailored recommendations for new literature based on previously reviewed articles.
2.4. Sentiment Analysis and Trend Detection
Machine learning can be employed to analyze sentiments expressed in research articles and track trends over time. This can help researchers understand the context of findings and gauge the impact of specific studies within the field.
Example Application: Sentiment analysis can be particularly useful in fields like healthcare and social sciences, where understanding public opinion and attitudes is essential.
3. Implementing Machine Learning for Literature Monitoring
To effectively use machine learning for literature monitoring, researchers should adopt a structured approach:
3.1. Identify Relevant Machine Learning Tools
Begin by identifying machine learning tools and platforms that align with your research needs. Popular tools for literature monitoring include:
Mendeley: This reference management software utilizes machine learning algorithms to recommend relevant articles based on user activity and preferences.
EndNote: EndNote offers features for organizing references and automated literature searches, enhanced by machine learning capabilities.
Rayyan: Rayyan is a web-based application that employs machine learning to assist researchers in conducting systematic reviews efficiently.
3.2. Define Keywords and Search Parameters
Once you have selected your tools, define the keywords and search parameters that will guide your literature monitoring. Consider the following:
Broad and Specific Keywords: Use a combination of broad and specific terms to capture a wide range of literature relevant to your field.
Boolean Operators: Incorporate Boolean operators (AND, OR, NOT) to refine searches and construct complex queries.
Synonyms and Variants: Include synonyms and related terms to ensure comprehensive coverage of the literature.
3.3. Train Machine Learning Models
If you are using custom-built machine learning models, you will need to train them on relevant datasets. The training process involves:
Data Collection: Gather a dataset of research articles that are representative of your field.
Labeling Data: Label the dataset with relevant categories, such as topic areas, quality assessments, or sentiment scores.
Model Selection: Choose an appropriate machine learning algorithm (e.g., decision trees, support vector machines, or neural networks) to train on the labeled dataset.
3.4. Automate Literature Monitoring
Once the machine learning model is trained, set up automated processes for literature monitoring:
Scheduled Searches: Schedule regular automated searches to retrieve new publications based on your predefined criteria.
Real-Time Alerts: Configure real-time alerts for newly published articles that match your search parameters, ensuring you receive timely updates.
3.5. Evaluate and Refine the System
Regularly evaluate the performance of your machine learning system to ensure it meets your research needs:
Performance Metrics: Assess the accuracy and relevance of the articles retrieved by the system. Common metrics include precision, recall, and F1 score.
User Feedback: Gather feedback from users to identify areas for improvement, such as adjusting search parameters or enhancing the model's ability to filter irrelevant content.
4. Case Studies: Successful Implementation of Machine Learning in Literature Monitoring
4.1. Healthcare Research
In healthcare research, staying updated with the latest clinical trials and medical literature is essential for patient safety and effective treatment. One example of successful machine learning implementation is the development of an automated literature monitoring system for pharmacovigilance.
Application: A pharmaceutical company utilized machine learning algorithms to monitor literature related to adverse drug reactions (ADRs). The system automatically scanned databases for relevant articles, extracting data on ADRs and flagging potential safety signals.
Outcome: By automating this process, the company significantly reduced the time spent on literature monitoring and improved its ability to respond to emerging safety concerns promptly.
4.2. Academic Research
In academia, machine learning is increasingly being used to simplify the literature review process for systematic reviews and meta-analyses. Research teams have adopted ML-powered tools to enhance their efficiency.
Application: A research team investigating the effectiveness of educational interventions employed machine learning algorithms to automate their literature review. The tool screened thousands of articles, extracting relevant data and summarizing key findings.
Outcome: The team completed their systematic review in a fraction of the time it would have taken using traditional methods, allowing them to focus on analysis and interpretation rather than manual searches.
5. Overcoming Challenges in Machine Learning Implementation
While machine learning offers numerous advantages for literature monitoring, challenges may arise during implementation:
5.1. Data Quality and Availability
Machine learning algorithms rely on high-quality, well-structured data. Researchers must ensure that the datasets used for training models are reliable and relevant.
Solution: Regularly evaluate data sources for credibility and consider cross-referencing findings with established databases to validate results.
5.2. Complexity of Algorithms
Machine learning algorithms can be complex, requiring researchers to have a certain level of technical expertise to implement and manage them effectively.
Solution: Utilize user-friendly ML tools that offer intuitive interfaces and provide comprehensive documentation and support.
5.3. Ethical Considerations
The use of machine learning raises ethical considerations, particularly regarding data privacy and the responsible use of algorithms.
Solution: Familiarize yourself with ethical guidelines related to machine learning and ensure compliance with regulations governing data use.
6. Conclusion
Machine learning is transforming the literature monitoring process, enabling researchers to stay updated with the latest findings efficiently. By automating literature searches, utilizing natural language processing, and implementing relevance ranking, machine learning simplifies the complexities of monitoring vast volumes of research.
As machine learning technology continues to advance, its applications in literature monitoring will likely expand, offering even more innovative solutions for researchers across various fields. By embracing these advancements, researchers can ensure they remain informed, competitive, and able to make impactful contributions to their disciplines. With the right approach, machine learning can truly simplify literature monitoring, allowing researchers to focus on what matters most—driving meaningful research and innovation.
留言