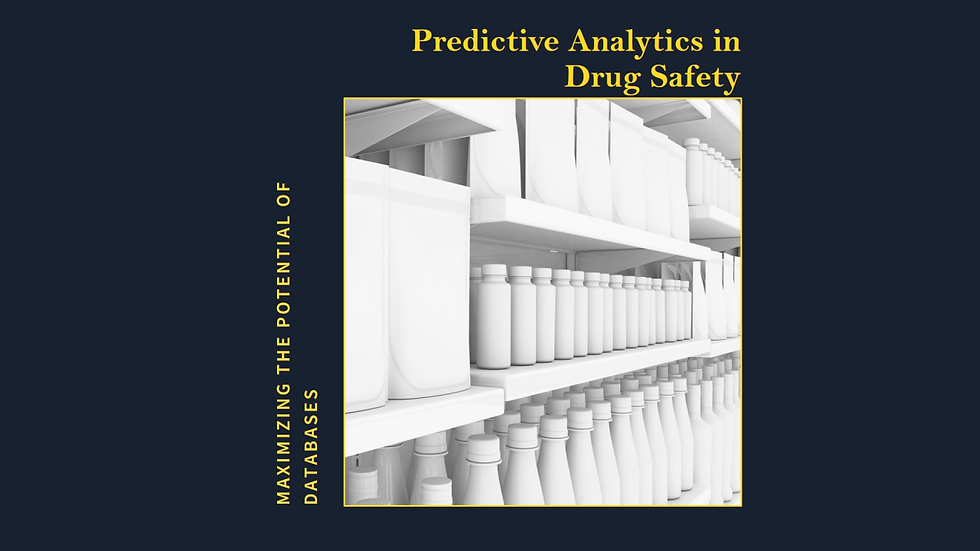
Predictive analytics has emerged as a powerful tool in the field of pharmacovigilance, offering the potential to identify safety concerns associated with pharmaceutical products more efficiently and effectively. Drug safety databases, which contain vast amounts of information on adverse events and drug reactions, are prime candidates for the application of predictive analytics techniques. This blog will explore the benefits of using predictive analytics in drug safety databases, common methods employed, and practical considerations for implementation.
Understanding Predictive Analytics in Pharmacovigilance:
Predictive analytics involves using historical data, statistical algorithms, and machine learning techniques to make predictions about future events or outcomes. In the context of pharmacovigilance, predictive analytics can be used to anticipate adverse drug reactions, identify potential safety signals, and prioritize resources for further investigation.
Benefits of Predictive Analytics in Drug Safety:
Early Detection of Safety Signals: Predictive analytics can help identify potential safety concerns earlier than traditional methods, allowing for timely intervention and risk mitigation.
Improved Efficiency: By automating the analysis of large volumes of data, predictive analytics can reduce the time and resources required to identify and investigate safety signals.
Enhanced Risk Assessment: Predictive models can provide more accurate risk assessments by analyzing multiple factors simultaneously, including patient demographics, medical history, and concomitant medications.
Personalized Medicine: Predictive analytics can help identify subpopulations at higher risk of adverse reactions, enabling personalized risk mitigation strategies and treatment plans.
Common Methods of Predictive Analytics in Drug Safety Databases:
Several predictive analytics techniques can be applied to drug safety databases to identify potential safety signals and assess the risk of adverse events. Some common methods include:
1. Signal Detection Algorithms
Signal detection algorithms analyze spontaneous reporting data to identify potential safety signals associated with specific drugs or medical products. These algorithms use statistical methods, such as disproportionality analysis and Bayesian modeling, to detect unusual patterns of adverse events that may indicate a safety concern.
2. Machine Learning Models
Machine learning models, such as logistic regression, decision trees, and neural networks, can be trained on historical data to predict the likelihood of adverse events or drug reactions. These models can incorporate various features, including patient characteristics, drug properties, and clinical outcomes, to make predictions with high accuracy.
3. Natural Language Processing (NLP)
NLP techniques can extract information from unstructured text data, such as medical narratives and adverse event reports, to identify relevant clinical information and adverse drug reactions. NLP algorithms can analyze text data from electronic health records, social media, and other sources to supplement structured data in drug safety databases.
4. Data Mining
Data mining techniques, such as association rule mining and clustering analysis, can uncover hidden patterns and relationships in drug safety databases. These techniques can identify co-occurring adverse events, common risk factors, and other insights that may not be apparent through traditional analysis methods.
Practical Considerations for Implementing Predictive Analytics:
While predictive analytics holds great promise for enhancing pharmacovigilance efforts, several practical considerations must be taken into account when implementing these techniques:
1. Data Quality and Accessibility
Effective predictive analytics relies on high-quality data that is accurate, complete, and accessible. Ensuring data integrity and standardization is essential for generating reliable predictions and insights.
2. Regulatory Compliance
Pharmaceutical companies and regulatory agencies must comply with stringent regulations governing the collection, storage, and analysis of drug safety data. Implementing predictive analytics techniques requires careful consideration of regulatory requirements and guidelines.
3. Interpretability and Transparency
Interpretable and transparent models are essential for gaining stakeholder trust and understanding the rationale behind predictive analytics predictions. Ensuring that models are explainable and interpretable can facilitate decision-making and risk assessment.
4. Collaboration and Communication
Successful implementation of predictive analytics in drug safety databases requires collaboration and communication among multidisciplinary teams, including data scientists, pharmacovigilance experts, clinicians, and regulatory professionals. Effective communication ensures that insights generated from predictive analytics are appropriately interpreted and acted upon.
5. Continuous Evaluation and Validation
Predictive analytics models should be continuously evaluated and validated to ensure their accuracy, reliability, and generalizability. Regular monitoring of model performance and validation against external data sources are critical for maintaining the effectiveness of predictive analytics techniques over time.
Conclusion:
Predictive analytics offers significant potential for enhancing pharmacovigilance efforts and improving patient safety. By leveraging historical data, statistical algorithms, and machine learning techniques, predictive analytics can help identify safety signals, assess the risk of adverse events, and inform risk mitigation strategies. While there are challenges and practical considerations associated with implementing predictive analytics in drug safety databases, the benefits of early detection, improved efficiency, and personalized risk assessment make it a valuable tool for pharmaceutical companies, regulatory agencies, and healthcare professionals. As the field of predictive analytics continues to evolve, it holds the promise of transforming drug safety surveillance and ultimately improving patient outcomes.
Comments