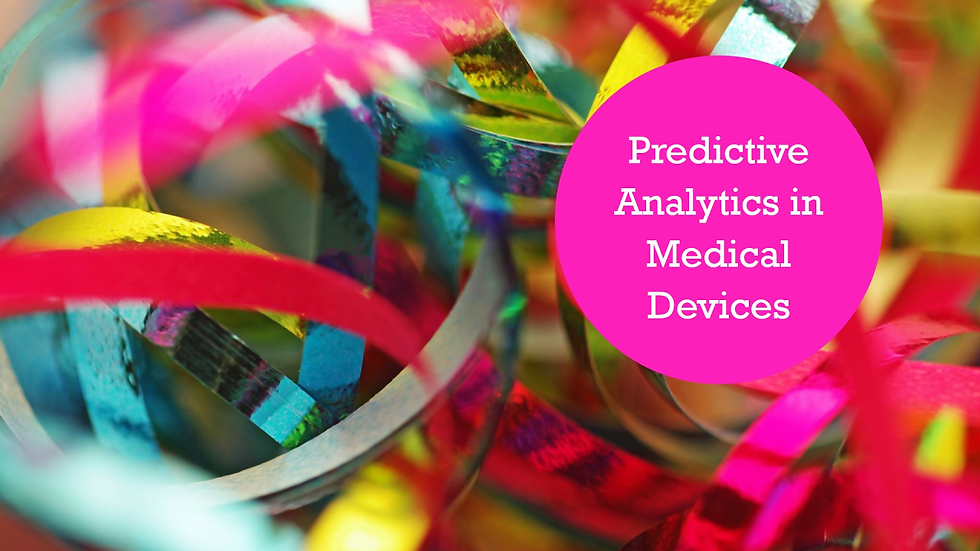
In an age where data reigns supreme, predictive analytics emerges as a powerful tool, especially in the healthcare sector. Medical device safety databases, repositories of crucial information regarding the performance and safety of medical devices, stand to benefit greatly from predictive analytics. This blog delves into the intricacies of leveraging predictive analytics within medical device safety databases, exploring its applications, benefits, challenges, and future prospects.
Understanding Predictive Analytics in Healthcare:
Predictive analytics involves the use of historical data, statistical algorithms, and machine learning techniques to forecast future events or behaviors. In the realm of healthcare, predictive analytics holds immense potential for improving patient outcomes, optimizing resource allocation, and enhancing decision-making processes. By analyzing patterns and trends within large datasets, predictive analytics can identify potential risks, predict patient outcomes, and guide personalized treatment plans.
The Role of Predictive Analytics in Medical Device Safety Databases:
Medical device safety databases serve as vital repositories of information regarding adverse events, device malfunctions, and patient outcomes associated with medical devices. By harnessing the power of predictive analytics, these databases can unlock valuable insights and enhance their effectiveness in several key areas.
1. Early Detection of Safety Issues
Predictive analytics can help identify early warning signs of potential safety issues associated with medical devices. By analyzing historical data on adverse events, device failures, and patient outcomes, predictive models can identify patterns or trends indicative of emerging safety concerns. Early detection enables regulatory authorities, healthcare providers, and manufacturers to take proactive measures to mitigate risks and prevent adverse events.
2. Risk Stratification and Prioritization
Not all medical devices pose the same level of risk to patients. Predictive analytics can assist in stratifying devices based on their risk profiles, enabling more targeted surveillance and intervention strategies. By analyzing factors such as device characteristics, patient demographics, and historical safety data, predictive models can prioritize devices that are most likely to pose a risk to patient safety. This risk-based approach allows for more efficient allocation of resources and regulatory oversight.
3. Enhanced Post-Market Surveillance
Post-market surveillance is critical for monitoring the safety and performance of medical devices after they have been approved for use. Predictive analytics can enhance post-market surveillance efforts by identifying potential safety signals and trends in real-time. By continuously analyzing data from medical device safety databases, predictive models can detect changes in device performance or patient outcomes that may warrant further investigation. This proactive approach helps regulatory authorities and healthcare providers identify and address safety issues before they escalate.
4. Predictive Maintenance and Quality Improvement
Predictive analytics can also be applied to improve the maintenance and quality of medical devices. By analyzing data on device usage, performance metrics, and maintenance history, predictive models can predict when a device is likely to experience a malfunction or failure. This enables healthcare providers and manufacturers to schedule preventive maintenance or replacement proactively, reducing the risk of unexpected downtime or adverse events. Additionally, predictive analytics can identify opportunities for quality improvement by identifying recurring issues or trends in device performance.
Implementing Predictive Analytics in Medical Device Safety Databases:
While the potential benefits of predictive analytics in medical device safety databases are significant, implementing predictive analytics poses several challenges and considerations.
1. Data Quality and Accessibility
The effectiveness of predictive analytics relies heavily on the quality and accessibility of data within medical device safety databases. Ensuring that data is accurate, complete, and standardized is essential for building reliable predictive models. Additionally, data accessibility is critical to enable seamless integration with predictive analytics tools and platforms.
2. Regulatory Compliance and Privacy
Compliance with regulatory requirements and patient privacy regulations is paramount when using predictive analytics in medical device safety databases. Healthcare organizations must adhere to regulations such as the Health Insurance Portability and Accountability Act (HIPAA) and the European Union's General Data Protection Regulation (GDPR) to safeguard patient data and maintain regulatory compliance.
3. Model Development and Validation
Developing and validating predictive models require expertise in data science, statistics, and domain-specific knowledge of medical devices. Healthcare organizations may need to collaborate with data scientists, statisticians, and healthcare professionals to develop and validate predictive models effectively. Additionally, ongoing validation and refinement of predictive models are necessary to ensure their accuracy and reliability over time.
4. Stakeholder Engagement and Collaboration
Successful implementation of predictive analytics in medical device safety databases requires collaboration and engagement among various stakeholders, including regulatory authorities, healthcare providers, manufacturers, and patients. Engaging stakeholders throughout the process helps ensure that predictive models align with their needs and priorities and fosters buy-in and support for predictive analytics initiatives.
Future Directions and Opportunities:
Despite the challenges associated with implementing predictive analytics in medical device safety databases, the future holds significant opportunities for leveraging predictive analytics to enhance patient safety and improve healthcare outcomes.
1. Advancements in Machine Learning and Artificial Intelligence
Advancements in machine learning and artificial intelligence (AI) present new opportunities for enhancing predictive analytics capabilities in medical device safety databases. Deep learning techniques, in particular, have shown promise for analyzing complex and heterogeneous datasets and identifying subtle patterns and trends that may not be apparent with traditional statistical methods.
2. Integration with Real-World Evidence
Integration of predictive analytics with real-world evidence (RWE) sources, such as electronic health records (EHRs) and patient registries, can enrich the predictive modeling process and provide a more comprehensive view of device safety and performance. By incorporating RWE into predictive models, healthcare organizations can leverage a broader range of data sources and enhance the accuracy and reliability of predictive analytics.
3. Patient-Centered Predictive Analytics
Incorporating patient-reported outcomes and experiences into predictive analytics models can provide valuable insights into the safety and performance of medical devices from the patient's perspective. Patient-centered predictive analytics can help identify patient-specific risk factors, preferences, and outcomes, enabling personalized risk assessment and treatment planning.
4. Collaborative Data Sharing Initiatives
Collaborative data sharing initiatives among healthcare organizations, regulatory authorities, and manufacturers can facilitate the sharing of data and insights for predictive analytics. Initiatives such as the International Medical Device Regulators Forum (IMDRF) promote data sharing and collaboration to improve device safety and enhance regulatory decision-making globally.
Conclusion:
Predictive analytics holds immense potential for enhancing the effectiveness of medical device safety databases and improving patient safety. By leveraging historical data, statistical algorithms, and machine learning techniques, predictive analytics can help identify early warning signs of safety issues, prioritize surveillance efforts, and inform decision-making processes.
While implementing predictive analytics in medical device safety databases poses challenges, the future holds significant opportunities for leveraging predictive analytics to enhance patient safety and improve healthcare outcomes. By embracing predictive analytics and collaborating across stakeholders, healthcare organizations can unlock valuable insights and drive continuous improvement in device safety and quality.
Interested to know more about our Medical Devices Safety Database? Book a demo!
Comments