I
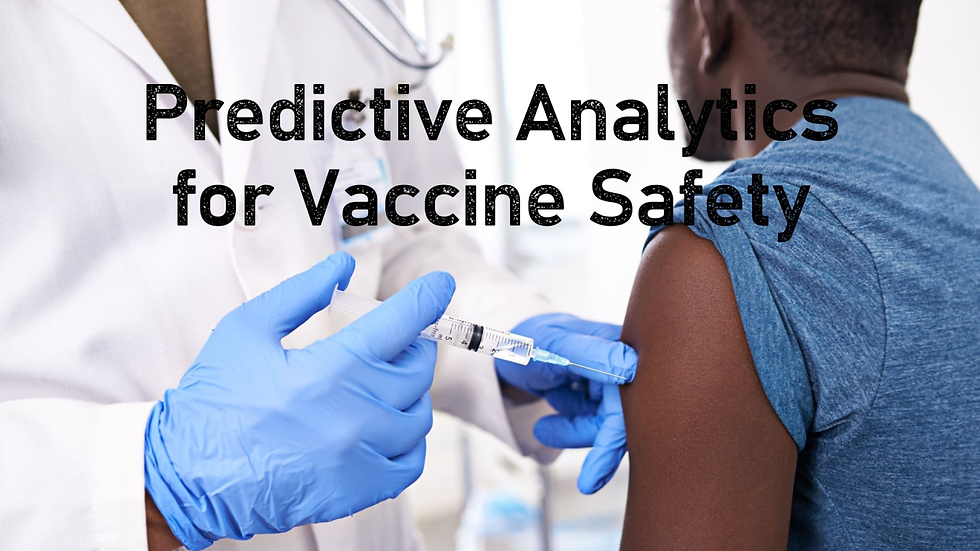
n the realm of public health, ensuring the safety and efficacy of vaccines is paramount. Vaccine safety databases play a crucial role in monitoring adverse events following immunization (AEFI) to identify potential risks and ensure timely intervention. With the advent of predictive analytics powered by Artificial Intelligence (AI) and Machine Learning (ML), these databases can go beyond traditional surveillance methods to forecast adverse events, personalize vaccination strategies, and enhance overall public health outcomes. This blog explores the application of predictive analytics in vaccine safety databases, its benefits, challenges, and future implications for vaccine safety monitoring.
Understanding Predictive Analytics in Vaccine Safety
1. Definition and Scope:
Predictive analytics involves using historical and real-time data to forecast future outcomes or trends. In vaccine safety, predictive analytics aims to identify patterns and predict adverse events based on various factors such as demographics, medical history, vaccine characteristics, and environmental factors.
2. Role in Vaccine Safety Databases:
Vaccine safety databases integrate predictive analytics to enhance early detection of adverse events, identify vulnerable populations, and optimize vaccine distribution strategies.
By analyzing large datasets, predictive models can generate insights that aid in decision-making, regulatory compliance, and public health policy development.
Benefits of Using Predictive Analytics:
1. Early Detection of Adverse Events:
Predictive models can detect subtle patterns in data that may indicate potential adverse events before they become widespread.
Early detection enables proactive measures to mitigate risks, such as adjusting vaccine administration protocols or enhancing surveillance in high-risk populations.
2. Personalized Vaccination Strategies:
By analyzing individual-level data, predictive analytics can tailor vaccination recommendations based on factors like age, medical history, genetic predisposition, and previous vaccine reactions.
Personalized strategies optimize vaccine efficacy and safety while minimizing adverse reactions, fostering patient-centric care.
3. Resource Allocation and Planning:
Predictive analytics inform resource allocation by predicting demand for vaccines, identifying geographical areas at higher risk of adverse events, and optimizing healthcare resource distribution.
Efficient planning improves response times to public health emergencies and enhances overall healthcare system resilience.
Implementing Predictive Analytics in Vaccine Safety Databases:
1. Data Collection and Integration:
Vaccine safety databases aggregate diverse data sources, including electronic health records (EHRs), clinical trials, adverse event reports, and demographic information.
Integration of structured and unstructured data ensures comprehensive analysis and robust predictive modeling.
2. Algorithm Selection and Development:
Selecting appropriate AI and ML algorithms depends on the specific objectives of predictive analytics in vaccine safety.
Algorithms such as decision trees, logistic regression, neural networks, and ensemble methods are tailored to analyze different types of data and predict outcomes effectively.
3. Model Training and Validation:
Training predictive models involves feeding them with labeled datasets to learn patterns and associations related to vaccine safety.
Rigorous validation processes assess model accuracy, reliability, and generalizability across diverse populations and vaccine formulations.
4. Real-Time Monitoring and Feedback Loop:
Continuous monitoring of predictive models ensures timely updates based on new data inputs and emerging trends.
Establishing a feedback loop enables iterative refinement of models, improving predictive accuracy and adaptability to evolving vaccine safety challenges.
Challenges and Considerations:
1. Data Quality and Integration:
Ensuring data completeness, accuracy, and consistency across disparate sources is crucial for reliable predictive analytics.
Data integration challenges may arise from varying data formats, standards, and interoperability issues between healthcare systems and databases.
2. Ethical and Privacy Concerns:
Protecting patient privacy and confidentiality while leveraging predictive analytics requires adherence to regulatory frameworks (e.g., GDPR, HIPAA).
Transparent communication and informed consent mechanisms uphold ethical standards in data use and analysis.
3. Interpretability and Transparency:
Complex AI and ML models may lack interpretability, posing challenges in understanding and validating predictive outcomes.
Incorporating explainable AI (XAI) techniques enhances model transparency, fostering trust among healthcare professionals, regulators, and the public.
Case Studies and Success Stories:
1. Flu Vaccine Distribution Optimization:
Predictive analytics optimized flu vaccine distribution by forecasting demand based on historical vaccination rates, demographic data, and influenza season predictions.
Efficient allocation reduced vaccine wastage and ensured equitable access, enhancing public health outcomes.
2. COVID-19 Vaccine Safety Monitoring:
During the COVID-19 pandemic, predictive models monitored vaccine safety by analyzing real-world data on adverse events and population-level health outcomes.
Rapid detection of safety signals informed regulatory decisions and public health communications, bolstering confidence in vaccination efforts.
Future Directions and Innovations:
1. Enhanced Data Interoperability:
Advancements in interoperable healthcare systems facilitate seamless data sharing and integration, supporting more comprehensive predictive analytics in vaccine safety.
2. Integration of Genomic Data:
Incorporating genomic information into predictive models enhances personalized medicine approaches to vaccine safety, considering genetic predispositions to adverse reactions.
3. AI-Driven Vaccine Development:
AI-driven simulations and predictive modeling accelerate vaccine development timelines by predicting vaccine efficacy, safety profiles, and immunogenicity.
Conclusion
Predictive analytics represents a transformative approach to enhancing vaccine safety through proactive risk assessment, personalized healthcare interventions, and optimized resource allocation. By leveraging AI and ML technologies within vaccine safety databases, healthcare systems can anticipate adverse events, tailor vaccination strategies, and uphold transparency in public health practices. Addressing challenges related to data quality, ethical considerations, and model interpretability is essential for maximizing the potential of predictive analytics in safeguarding global immunization efforts. As innovation continues, the integration of predictive analytics promises to redefine vaccine safety management and advance public health resilience in the face of emerging infectious diseases and evolving healthcare landscapes.
Comments