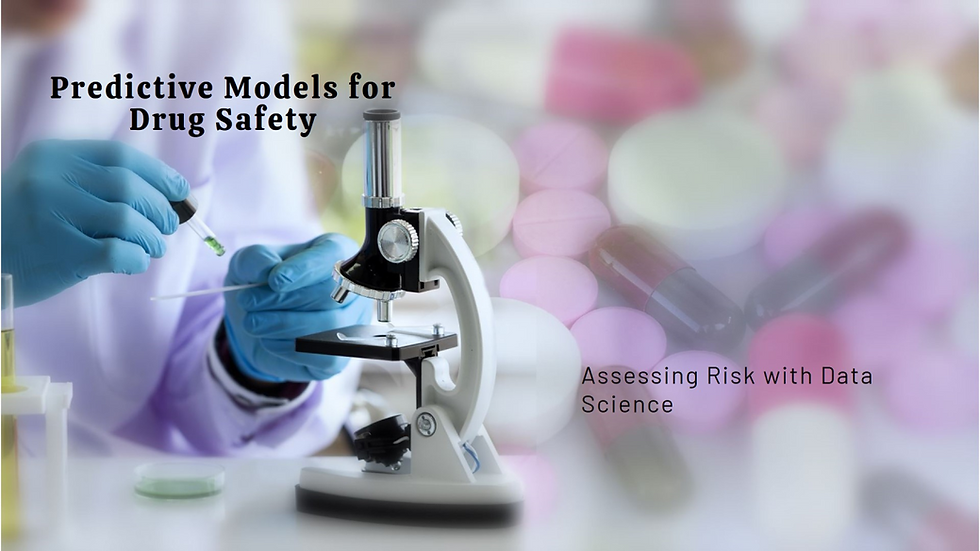
Predictive modeling in drug safety risk assessment has revolutionized pharmacovigilance by enabling proactive identification of potential adverse events associated with pharmaceutical products. This blog explores the principles of predictive modeling, its applications in drug safety, benefits, challenges, and future directions.
Understanding Predictive Models in Drug Safety:
Predictive models leverage statistical algorithms, machine learning (ML), and artificial intelligence (AI) techniques to analyze large datasets and forecast outcomes related to drug safety:
Risk Prediction: Assessing the likelihood and severity of adverse drug reactions (ADRs) based on patient characteristics, drug properties, and other factors.
Signal Detection: Identifying emerging safety concerns and potential safety signals from real-world data sources, complementing clinical trial findings.
Applications of Predictive Models in Drug Safety:
1. Early Warning Systems
Predictive models serve as early warning systems by:
Real-time Surveillance: Monitoring adverse events in real-time to detect safety signals promptly.
Pattern Recognition: Identifying patterns and trends in data that may indicate potential risks associated with specific medications.
2. Comparative Effectiveness Research
Assessing the safety profiles of drugs in real-world settings compared to standard treatments or alternative therapies:
Outcome Prediction: Predicting patient outcomes and treatment responses to optimize therapeutic decision-making.
Longitudinal Analysis: Analyzing longitudinal data to understand the cumulative effects and safety profiles of medications over time.
Benefits of Predictive Models in Drug Safety Risk Assessment:
1. Proactive Risk Management
Preventive Measures: Anticipating potential risks allows for proactive risk mitigation strategies.
Resource Allocation: Allocating resources efficiently based on predicted safety risks and priorities.
2. Enhanced Efficiency and Cost Savings
Streamlined Processes: Automating data analysis and risk assessment processes reduce manual effort and improve efficiency.
Cost-effective Interventions: Targeting interventions based on predicted risks minimizes costs associated with adverse events and safety monitoring.
3. Improved Decision-making and Regulatory Compliance
Evidence-based Decisions: Providing robust evidence for regulatory agencies to make informed decisions on drug approval, labeling, and safety alerts.
Compliance with Standards: Meeting regulatory requirements for pharmacovigilance and safety reporting through validated predictive models.
Challenges in Utilizing Predictive Models:
1. Data Quality and Integration
Data Variability: Ensuring consistency and quality of data across diverse sources for accurate modeling.
Data Accessibility: Accessing comprehensive and timely data from electronic health records (EHRs), adverse event reporting systems, and other databases.
2. Model Interpretability and Validation
Transparency: Ensuring transparency in model outputs and predictions to gain stakeholder trust and acceptance.
Validation Requirements: Validating predictive models with real-world data to assess accuracy, reliability, and generalizability.
3. Ethical and Regulatory Considerations
Privacy Concerns: Safeguarding patient confidentiality and adhering to data protection regulations (e.g., GDPR, HIPAA).
Bias and Fairness: Addressing biases in data and models to ensure equitable predictions and decisions in healthcare settings.
Best Practices and Implementation Strategies:
1. Collaborative Partnerships
Multi-disciplinary Teams: Engaging clinicians, data scientists, statisticians, and regulatory experts to develop and validate predictive models collaboratively.
Industry Collaboration: Sharing data and insights through industry partnerships to enhance predictive modeling capabilities across organizations.
2. Continuous Learning and Model Refinement
Iterative Improvement: Iteratively refining models based on new data inputs, emerging safety signals, and feedback from healthcare professionals.
Adaptive Algorithms: Developing adaptive algorithms that can evolve with changing healthcare landscapes and patient demographics.
3. Education and Training
Healthcare Professional Training: Providing training programs to educate healthcare providers on interpreting predictive model outputs and integrating findings into clinical practice.
Public Awareness: Educating patients and the public about the role of predictive modeling in drug safety and the importance of reporting adverse events.
Future Directions and Innovations:
1. Advanced Analytical Techniques
AI-driven Insights: Leveraging AI for deep learning and natural language processing to extract insights from unstructured data sources.
Real-time Analytics: Implementing real-time analytics platforms for continuous monitoring and rapid response to safety signals.
2. Personalized Medicine and Pharmacovigilance
Precision Pharmacovigilance: Tailoring safety assessments based on patient-specific factors, genetic profiles, and personalized treatment regimens.
Genomic Data Integration: Incorporating genomic data to understand individual variability in drug responses and safety outcomes.
3. Global Harmonization and Data Sharing
Standardization Efforts: Harmonizing data standards and regulatory frameworks to facilitate cross-border data sharing and collaborative research initiatives.
Open Data Initiatives: Promoting transparency and accessibility of predictive modeling results through open data platforms and public repositories.
Conclusion:
Predictive models are powerful tools in drug safety risk assessment, enabling healthcare stakeholders to anticipate and mitigate potential risks associated with medications. By overcoming challenges in data quality, interpretability, and ethical considerations, predictive modeling can enhance decision-making, regulatory compliance, and patient safety outcomes. Embracing technological advancements, collaborative partnerships, and continuous learning will be pivotal in advancing predictive modeling capabilities and shaping the future of pharmacovigilance in personalized medicine.
Commenti