How Vaccine Safety Databases Aid in Detecting Rare Adverse Events
- Chaitali Gaikwad
- Jul 2, 2024
- 6 min read
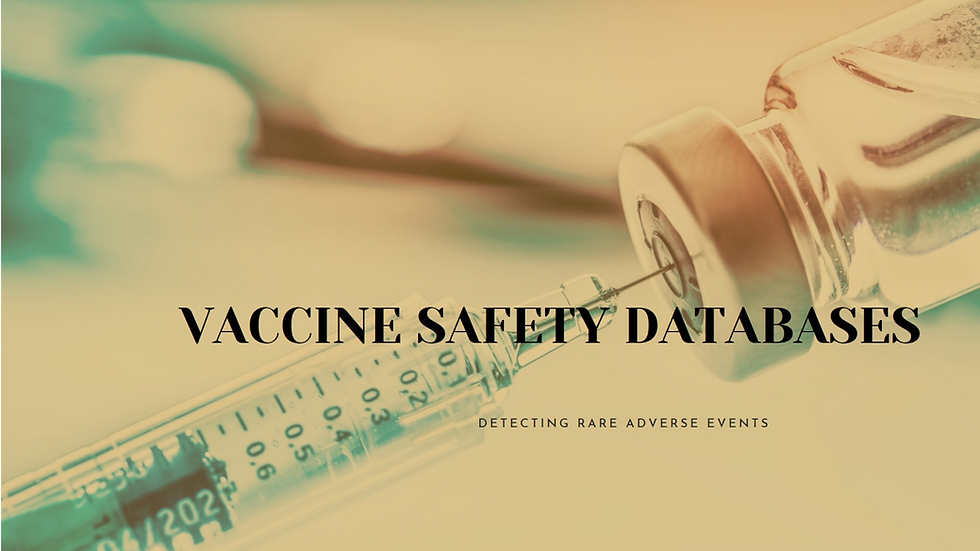
Vaccine safety databases play a critical role in public health by monitoring and detecting adverse events associated with vaccines. While vaccines are generally safe and effective, rare adverse events can occur. Detecting these rare events is crucial for maintaining public confidence in vaccination programs and ensuring the overall safety of vaccines. This blog explores how vaccine safety databases aid in detecting rare adverse events, the methods used to analyze data, and the challenges involved.
The Importance of Detecting Rare Adverse Events:
Rare adverse events are infrequent, serious, and often only become apparent after a vaccine is widely distributed. Detecting these events is essential for several reasons:
Public Trust: Transparency in identifying and managing adverse events helps maintain public confidence in vaccination programs.
Safety and Efficacy: Ensuring the safety and efficacy of vaccines protects individuals from potential harm.
Regulatory Compliance: Adhering to regulatory requirements for monitoring and reporting adverse events is crucial for vaccine approval and ongoing use.
Scientific Understanding: Identifying rare adverse events contributes to the broader understanding of vaccine-related risks and benefits.
How Vaccine Safety Databases Work:
Vaccine safety databases collect and analyze data on adverse events reported by healthcare providers, patients, and manufacturers. These databases use various surveillance methods to monitor vaccine safety:
Passive Surveillance: Systems like the Vaccine Adverse Event Reporting System (VAERS) in the United States rely on voluntary reporting of adverse events by healthcare providers, patients, and manufacturers.
Active Surveillance: Systems like the Vaccine Safety Datalink (VSD) actively collect data from specific populations through electronic health records (EHRs) and other sources.
Enhanced Surveillance: Combining elements of both passive and active surveillance to provide a more comprehensive view of vaccine safety.
Methods for Detecting Rare Adverse Events:
Several analytical methods are employed to detect rare adverse events in vaccine safety databases:
Signal Detection
Statistical Analysis
Machine Learning and Artificial Intelligence
Data Linkage
Case-Control Studies
1. Signal Detection
Signal detection involves identifying patterns or trends in the data that suggest a possible association between a vaccine and an adverse event. This process typically includes:
Proportional Reporting Ratios (PRRs): Comparing the proportion of a specific adverse event reported for a particular vaccine to the proportion reported for other vaccines.
Bayesian Data Mining: Using Bayesian statistical methods to identify signals by comparing observed and expected rates of adverse events.
Empirical Bayes Screening: Adjusting for multiple comparisons and identifying signals by comparing observed and expected frequencies of adverse events.
2. Statistical Analysis
Statistical analysis is essential for validating signals and determining whether an observed association between a vaccine and an adverse event is likely to be causal. Key statistical methods include:
Chi-Square Tests: Comparing the observed and expected frequencies of adverse events to determine if there is a significant association.
Relative Risk (RR): Calculating the risk of an adverse event occurring in vaccinated individuals compared to unvaccinated individuals.
Confidence Intervals (CIs): Estimating the range of values within which the true effect size is likely to lie, providing a measure of precision for the observed association.
3. Machine Learning and Artificial Intelligence
Machine learning (ML) and artificial intelligence (AI) techniques can enhance the detection of rare adverse events by analyzing large and complex datasets. These techniques include:
Supervised Learning: Training algorithms on labeled data to predict the occurrence of adverse events based on patterns in the data.
Unsupervised Learning: Identifying clusters or patterns in the data without prior knowledge of specific adverse events.
Natural Language Processing (NLP): Analyzing unstructured data, such as free-text adverse event reports and medical records, to identify potential signals.
4. Data Linkage
Data linkage involves combining data from multiple sources to create a more comprehensive dataset for analysis. This can include linking:
Electronic Health Records (EHRs): Integrating EHR data with vaccine safety databases to capture detailed clinical information on adverse events.
Pharmacy Records: Including data on medication usage to identify potential interactions between vaccines and other drugs.
Registry Data: Using disease registries to identify populations at higher risk of adverse events and monitor long-term outcomes.
5. Case-Control Studies
Case-control studies are observational studies that compare individuals who have experienced an adverse event (cases) with those who have not (controls). These studies are useful for:
Identifying Risk Factors: Determining which factors are associated with an increased risk of adverse events.
Quantifying Associations: Estimating the strength of the association between vaccination and adverse events.
Hypothesis Generation: Developing hypotheses about potential causal relationships that can be tested in further studies.
Challenges in Detecting Rare Adverse Events:
Detecting rare adverse events in vaccine safety databases is challenging due to several factors:
Data Quality and Completeness
Underreporting
Confounding Factors
Statistical Power
Timeliness
1. Data Quality and Completeness
Ensuring high-quality and complete data is essential for accurate analysis. Challenges include:
Inconsistent Reporting: Variability in how adverse events are reported can affect data quality.
Missing Data: Incomplete records can lead to biased analysis and incorrect conclusions.
Data Standardization: Lack of standardized terminology and coding can make it difficult to compare data across different sources.
2. Underreporting
Underreporting of adverse events is a significant challenge, particularly in passive surveillance systems. Factors contributing to underreporting include:
Awareness: Healthcare providers and patients may not be aware of the reporting system or the importance of reporting adverse events.
Perceived Severity: Mild or common adverse events may not be reported as frequently as serious events.
Administrative Burden: The process of reporting adverse events can be time-consuming and complex.
3. Confounding Factors
Confounding factors can complicate the analysis of adverse events. These include:
Underlying Health Conditions: Pre-existing health conditions can affect the risk of adverse events and complicate the analysis.
Co-Administration of Vaccines: When multiple vaccines are administered simultaneously, it can be challenging to determine which vaccine is associated with an adverse event.
External Factors: Environmental and behavioral factors can also influence the risk of adverse events.
4. Statistical Power
Detecting rare adverse events requires large sample sizes to achieve sufficient statistical power. Challenges include:
Sample Size: Small sample sizes can limit the ability to detect rare events with statistical significance.
Data Availability: Limited access to comprehensive data can constrain the analysis.
5. Timeliness
Timely detection of adverse events is crucial for prompt intervention. Challenges include:
Data Collection and Processing: Delays in data collection, processing, and analysis can hinder timely detection of adverse events.
Reporting Lag: Time lag between the occurrence of an adverse event and its reporting can delay detection and response.
Strategies for Improving Detection of Rare Adverse Events:
Addressing the challenges in detecting rare adverse events requires a multifaceted approach. Strategies include:
Enhancing Data Collection and Quality
Promoting Reporting and Awareness
Improving Analytical Methods
Collaborative Efforts
Leveraging Technology
1. Enhancing Data Collection and Quality
Improving data collection and quality involves:
Standardized Reporting: Implementing standardized reporting formats and terminologies to ensure consistency and comparability of data.
Training and Education: Providing training and education to healthcare providers and data collectors on accurate and complete reporting of adverse events.
Data Validation and Cleaning: Implementing data validation checks and regular cleaning to identify and correct errors, inconsistencies, and missing values.
2. Promoting Reporting and Awareness
Promoting reporting and awareness involves:
Education Campaigns: Raising awareness among healthcare providers and the public about the importance of reporting adverse events.
Simplified Reporting Processes: Streamlining reporting processes to reduce administrative burden and encourage reporting.
Incentives: Providing incentives for healthcare providers and patients to report adverse events.
3. Improving Analytical Methods
Enhancing analytical methods involves:
Advanced Statistical Techniques: Employing advanced statistical techniques, such as Bayesian methods and machine learning, to improve signal detection and analysis.
Real-Time Monitoring: Developing real-time monitoring systems to detect and respond to adverse events promptly.
Integration of Multiple Data Sources: Integrating data from diverse sources to create a comprehensive dataset for analysis.
4. Collaborative Efforts
Collaborative efforts involve:
Global Partnerships: Collaborating with international organizations, such as the World Health Organization (WHO), to share data and insights on vaccine safety.
Cross-Sector Collaboration: Working with pharmaceutical companies, regulatory authorities, and healthcare organizations to improve data collection and analysis.
Public-Private Partnerships: Establishing public-private partnerships to leverage resources and expertise for vaccine safety monitoring.
5. Leveraging Technology
Leveraging technology involves:
Artificial Intelligence (AI): Utilizing AI and machine learning algorithms to analyze large and complex datasets and identify patterns.
Blockchain: Implementing blockchain technology to ensure data integrity and security.
Mobile Health (Health): Using Health technologies, such as mobile apps and wearable devices, to collect real-time data on adverse events.
Conclusion:
Vaccine safety databases are indispensable tools for detecting rare adverse events and ensuring the safety of vaccines. By employing robust surveillance
Comentários