Leveraging Machine Learning: Advancements in Safety Database Analysis
- Chaitali Gaikwad
- Apr 22, 2024
- 4 min read
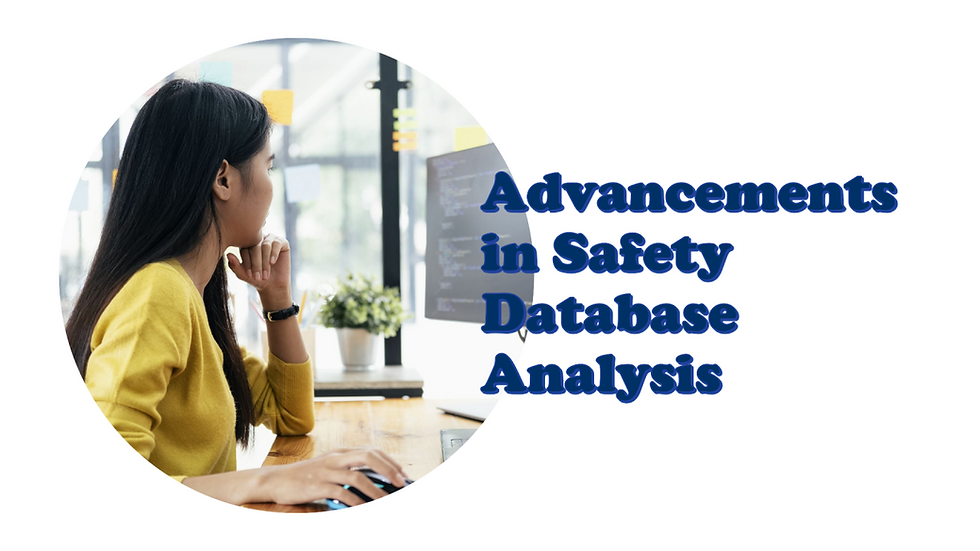
In an era where data is abundant and technology is rapidly evolving, industries across the board are harnessing the power of machine learning to drive innovation and efficiency. One such field where machine learning is making significant strides is safety database analysis. With the advent of sophisticated algorithms and the availability of vast amounts of data, organizations are now able to extract valuable insights from safety databases to enhance safety measures, identify potential risks, and improve overall operational efficiency.
Safety database analysis plays a critical role in various industries, including healthcare, aviation, automotive, manufacturing, and pharmaceuticals. These industries rely on extensive safety databases to record and monitor incidents, accidents, adverse events, and other safety-related information. Traditionally, analyzing these databases involved manual review processes, which were time-consuming, labor-intensive, and prone to errors. However, with the integration of machine learning techniques, organizations can now automate and streamline the analysis process, enabling them to uncover hidden patterns, trends, and correlations that may have otherwise gone unnoticed.
One of the key advancements in safety database analysis enabled by machine learning is predictive analytics. By leveraging historical data and machine learning algorithms, organizations can predict potential safety issues before they occur, allowing them to proactively implement preventive measures and mitigate risks. For example, in the healthcare industry, predictive analytics can be used to anticipate patient safety incidents such as medication errors, hospital-acquired infections, or adverse drug reactions. By analyzing patient data, medical records, and other relevant information, machine learning models can identify patients at high risk of experiencing adverse events, enabling healthcare providers to intervene early and prevent harm.
Another area where machine learning is revolutionizing safety database analysis is anomaly detection. Anomalies, or deviations from normal patterns or behaviors, can indicate potential safety hazards or security breaches. Traditional methods of anomaly detection often rely on predefined rules or thresholds, which may not capture all types of anomalies or may generate false positives. Machine learning-based anomaly detection algorithms, on the other hand, can adapt and learn from data, making them more effective at detecting subtle deviations and outliers. In the aviation industry, for instance, machine learning algorithms can analyze flight data, maintenance records, and other relevant information to identify anomalies that may indicate safety issues, such as unusual aircraft behavior or equipment malfunctions.
Furthermore, machine learning enables organizations to perform complex pattern recognition tasks on safety databases, facilitating the identification of recurring trends or common risk factors. By analyzing vast amounts of structured and unstructured data, machine learning models can uncover hidden correlations and causal relationships that traditional statistical methods may overlook. This capability is particularly valuable in industries such as pharmaceuticals, where adverse drug reactions and safety concerns need to be closely monitored and investigated. Machine learning algorithms can analyze clinical trial data, patient reports, and other sources to identify patterns of adverse reactions or safety risks associated with specific drugs or medical treatments, enabling regulatory agencies and pharmaceutical companies to take appropriate actions to ensure patient safety.
Additionally, machine learning techniques such as natural language processing (NLP) are being increasingly utilized to extract valuable insights from unstructured data sources, such as incident reports, safety narratives, and social media posts. NLP algorithms can analyze text data, identify key phrases or keywords, and extract relevant information to supplement structured data analysis. For example, in the automotive industry, NLP can be used to analyze customer complaints and feedback to identify potential safety issues or product defects. By combining structured and unstructured data analysis techniques, organizations can gain a more comprehensive understanding of safety-related risks and trends, enabling them to make more informed decisions and take proactive measures to mitigate risks.
Moreover, machine learning-based safety database analysis allows for the integration of real-time data streams and sensor data, enabling organizations to monitor safety-critical systems and environments in real-time. By continuously analyzing incoming data streams, machine learning models can detect anomalies, predict potential safety hazards, and trigger timely alerts or interventions. This capability is particularly valuable in industries such as manufacturing and energy, where safety incidents can have severe consequences for personnel and the environment. Real-time monitoring and analysis enable organizations to identify and address safety issues promptly, minimizing the likelihood of accidents or disruptions.
However, despite the numerous benefits of leveraging machine learning for safety database analysis, there are also challenges and considerations that organizations need to address. One major challenge is data quality and consistency. Safety databases often contain heterogeneous data from various sources, including different formats, structures, and levels of granularity. Ensuring data quality and consistency is essential for the accuracy and reliability of machine learning models. Organizations need to invest in data preprocessing and cleansing techniques to standardize and harmonize data before feeding it into machine learning algorithms.
Another challenge is interpretability and transparency. Machine learning models, particularly deep learning models, are often criticized for their lack of interpretability, making it difficult for stakeholders to understand how decisions are made. In safety-critical applications, such as healthcare and aviation, interpretability is crucial for building trust and confidence in machine learning-based systems. Organizations need to develop techniques and tools for explaining and visualizing the decisions made by machine learning models, enabling stakeholders to understand the rationale behind safety recommendations or predictions.
Furthermore, ensuring the security and privacy of sensitive data is paramount when leveraging machine learning for safety database analysis. Safety databases may contain confidential or personally identifiable information, such as patient records or proprietary product data. Organizations need to implement robust data security measures, such as encryption, access controls, and anonymization techniques, to protect sensitive information from unauthorized access or disclosure. Additionally, compliance with data protection regulations, such as the Health Insurance Portability and Accountability Act (HIPAA) or the General Data Protection Regulation (GDPR), is essential to avoid legal and regulatory repercussions.
Conclusion:
In conclusion, leveraging machine learning for safety database analysis holds immense promise for enhancing safety measures, identifying potential risks, and improving operational efficiency across various industries. From predictive analytics and anomaly detection to pattern recognition and real-time monitoring, machine learning enables organizations to extract valuable insights from safety databases and take proactive measures to mitigate risks and ensure safety. However, addressing challenges related to data quality, interpretability, and data security is crucial to realizing the full potential of machine learning in safety-critical applications. By overcoming these challenges and harnessing the power of machine learning, organizations can create safer and more resilient systems and environments for the benefit of society as a whole.
Comments